Region-Aware Semantic Consistency for Unsupervised Domain-Adaptive Semantic Segmentation.
ICME(2023)
摘要
As acquiring pixel-wise labels for semantic segmentation is labor-intensive, unsupervised domain adaptation (UDA) techniques aim to transfer knowledge from synthetic data to real-scene data. To overcome the distribution misalignment between the source domain and the target domain, Teacher-Student (TS) methods are widely-used and promising. In TS methods, the student resorts to the one-hot pseudo labels generated by the teacher. However, the generated one-hot pseudo labels are dubious and ignore the semantic correlation among classes. Besides, in the same position of the same image, the output distributions between the student and the teacher should be consistent. Such prediction consistency is defined as Region-Aware Semantic Consistency (RASC). Correspondingly, we propose an RASC module to assimilate the output distributions of the teacher and the student. Our RASC module is flexible and easily plugged into TS state-of-the-arts (SOTAs) based on either CNNs or Transformers.
更多查看译文
关键词
Unsupervised Domain Adaptation, Semantic Segmentation, Self-training, Teacher-student Methods
AI 理解论文
溯源树
样例
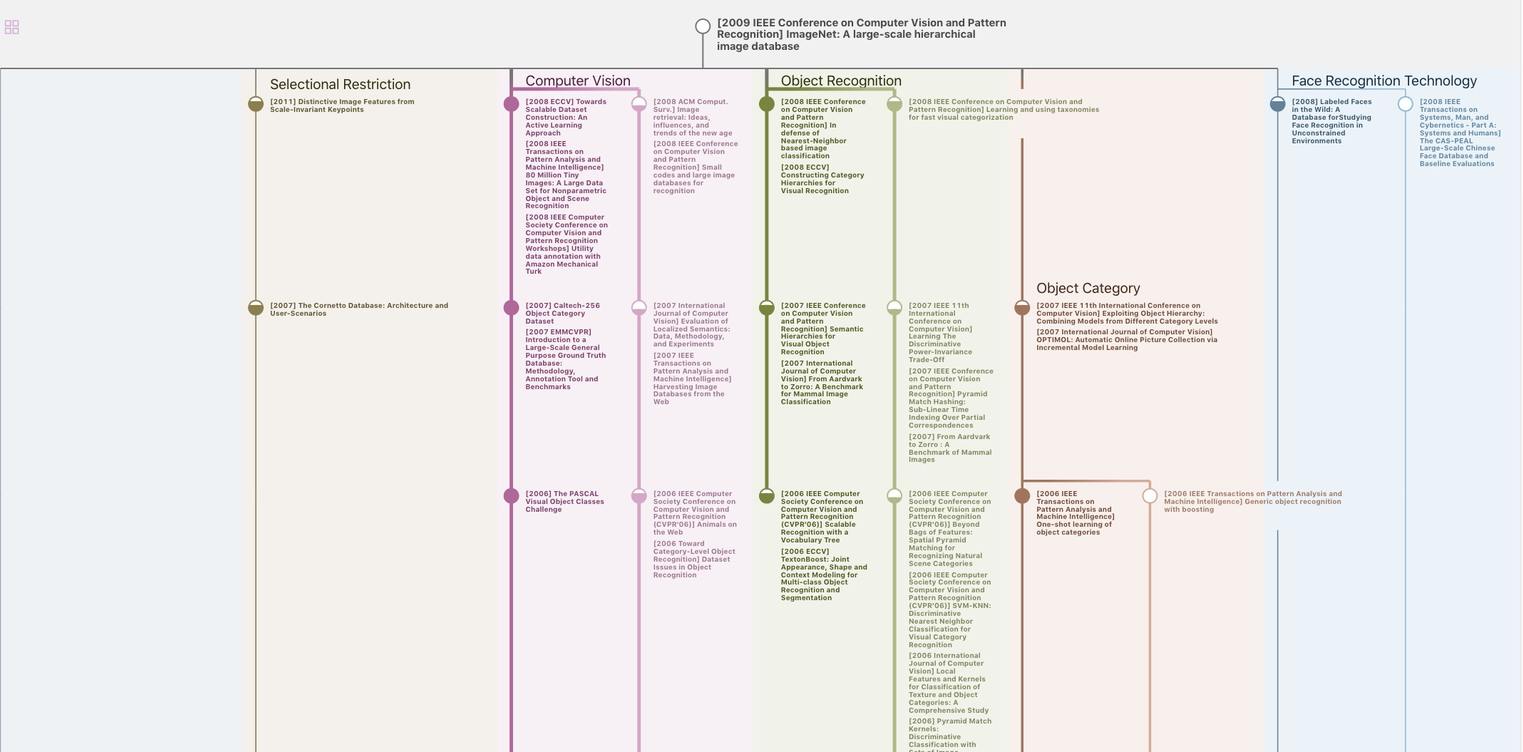
生成溯源树,研究论文发展脉络
Chat Paper
正在生成论文摘要