End-To-End Part-Level Action Parsing With Transformer.
ICME(2023)
摘要
The divide-and-conquer strategy, which interprets part-level action parsing as a detect-then-parsing pipeline, has been widely used and become a general tool for part-level action understanding. However, existing methods that derive from the strategy usually suffer from either strong dependence on prior detection or high computational complexity. In this paper, we present the first fully end-to-end part-level action parsing framework with transformers, termed PATR. Unlike existing methods, our method regards part-level action parsing as a hierarchical set prediction problem and unifies person detection, body part detection, and action state recognition into one model. In PATR, predefined learnable representations, including general instance representations and general part representations, are guided to adaptively attend to the image features that are relevant to target body parts. Then, conditioning on corresponding learnable representations, attended image features are hierarchically decoded into corresponding semantics (i.e., person location, body part location, and action states for each body part). In this way, PATR relies on characteristics of body parts, instead of prior predictions like bounding boxes, to parse action states, thus removing the strong dependence between sub-tasks and eliminating the computational burdens caused by the multi-stage paradigm. Extensive experiments conducted on challenging Kinetic-TPS indicate that our method achieves very competitive results. In particular, our model outperforms all stateof-the-art part-level action parsing approaches by a margin, reaching around 3.8 +/- 2.0% Accp higher than previous methods. These findings indicate the potential of PATR to serve as a new baseline for part-level action parsing methods in the future. Our code and models are publicly available.
更多查看译文
关键词
human parsing, part identification, action recognition
AI 理解论文
溯源树
样例
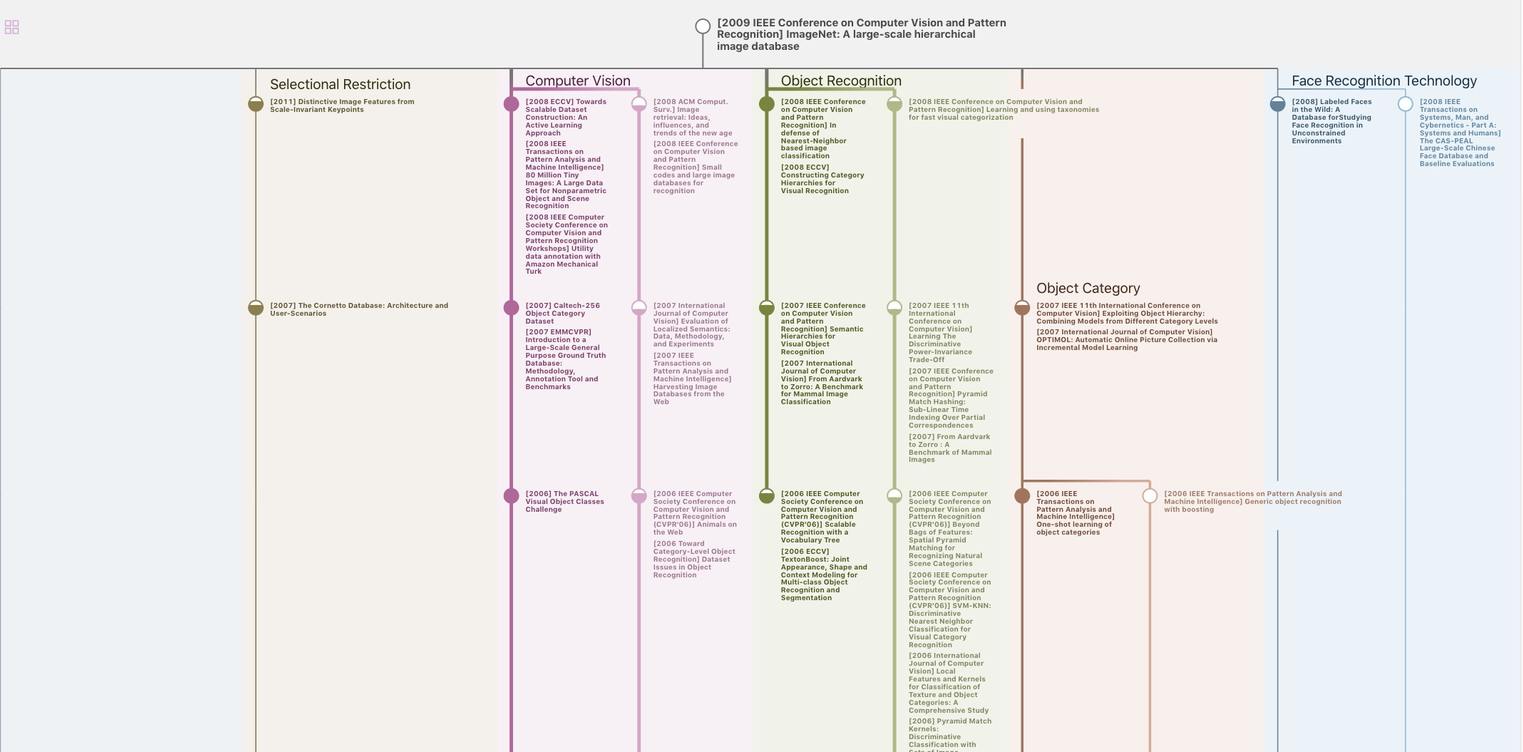
生成溯源树,研究论文发展脉络
Chat Paper
正在生成论文摘要