A Pseudo-Dual Self-Rectification Framework for Semantic Segmentation.
ICME(2023)
摘要
Semantic segmentation has achieved remarkable success in various applications. However, the training process for such techniques necessitates a significant amount of labeled data. Although semi-supervised frameworks can alleviate this issue, traditional approaches typically require multiple baseline models to form a dual model. To allow a semi-supervised semantic segmentation framework to be used in robotic systems with precious computation and memory resources, we propose a framework utilizing a single baseline model only. The overall framework is composed of three parts: an encoder, a shallow decoder, and a deep decoder. It distills knowledge from the ensemble of two decoders to improve the encoder, which can implicitly form a pseudo-dual model. It also calculates class-wise likelihoods according to the similarity between features and class prototypes learned from different decoders and rectifies low-confidence pseudo-labels. Our framework outperforms state-of-the-art frameworks on benchmark datasets with a significant amount of decrease in using computing resources.
更多查看译文
关键词
Semantic segmentation, Semi-supervised learning, Error rectification
AI 理解论文
溯源树
样例
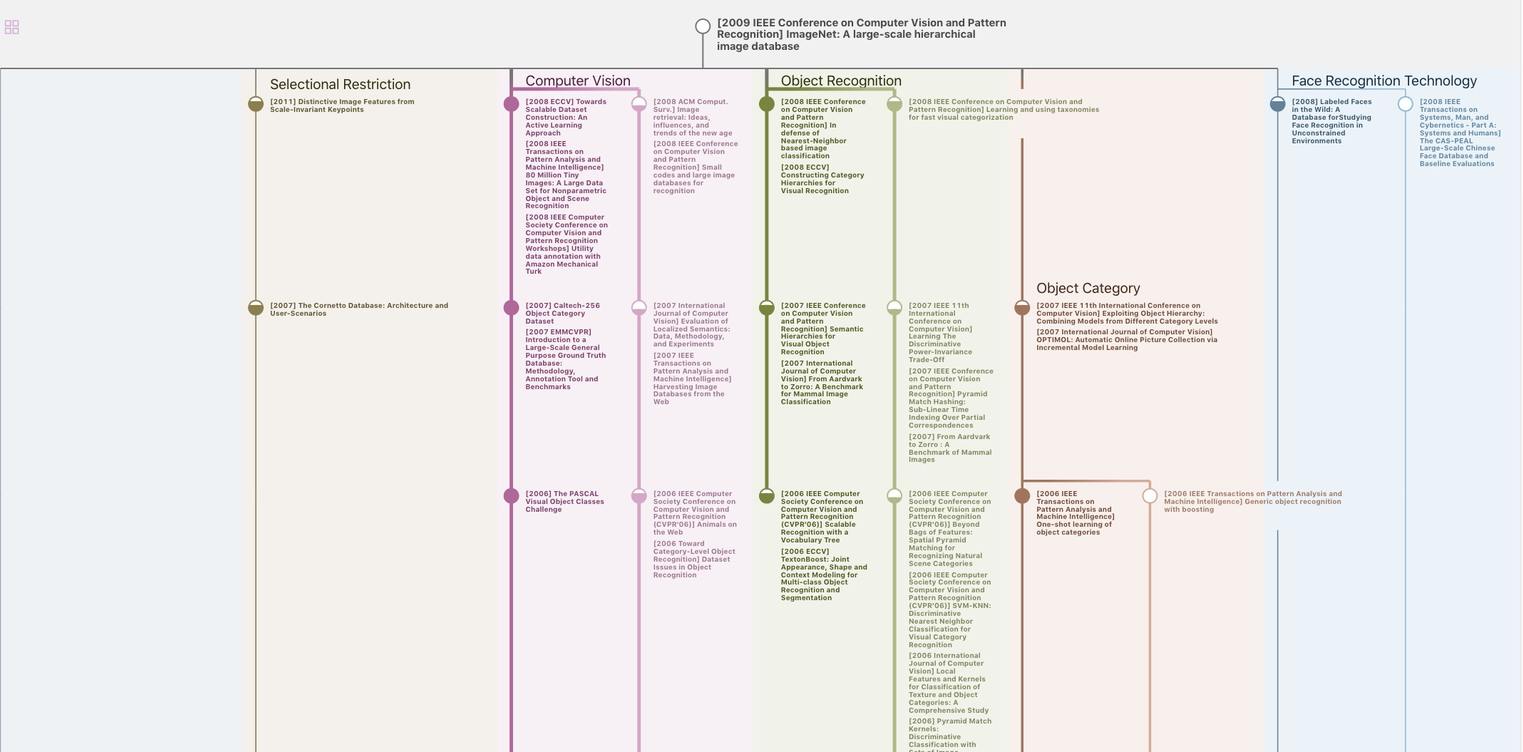
生成溯源树,研究论文发展脉络
Chat Paper
正在生成论文摘要