Adapt then Generalize: A Simple Two-Stage Framework for Semi-Supervised Domain Generalization.
ICME(2023)
摘要
Semi-supervised domain generalization (SSDG) focuses on training a model on a single labeled source domain and several unlabeled source domains simultaneously for the purpose of generalizing to out-of-distribution domain. To prevent model overfitting on the unlabeled source domains and enhance model generalization, we propose an effective two-stage framework that disentangle the SSDG task into a burn-in stage and a mutual-training stage. In the burn-in stage, we train a domain adaptation model to reduce domain gap and produce high-accuracy pseudo labels for the unlabeled data. In the second stage, we devise two peer networks equipped with style randomization modules to take advantage of both the style and class information from the pseudo-labeled data. The peer networks are mutually teaching each other, which allows them to avoid overfitting to noisy data and ultimately improves generalization ability. Extensive experiments show that our method achieves state-of-the-art performance on various benchmarks, demonstrating the effectiveness of our method on SSDG.
更多查看译文
关键词
domain generalization,semi-supervised learning,transfer learning,image recognition
AI 理解论文
溯源树
样例
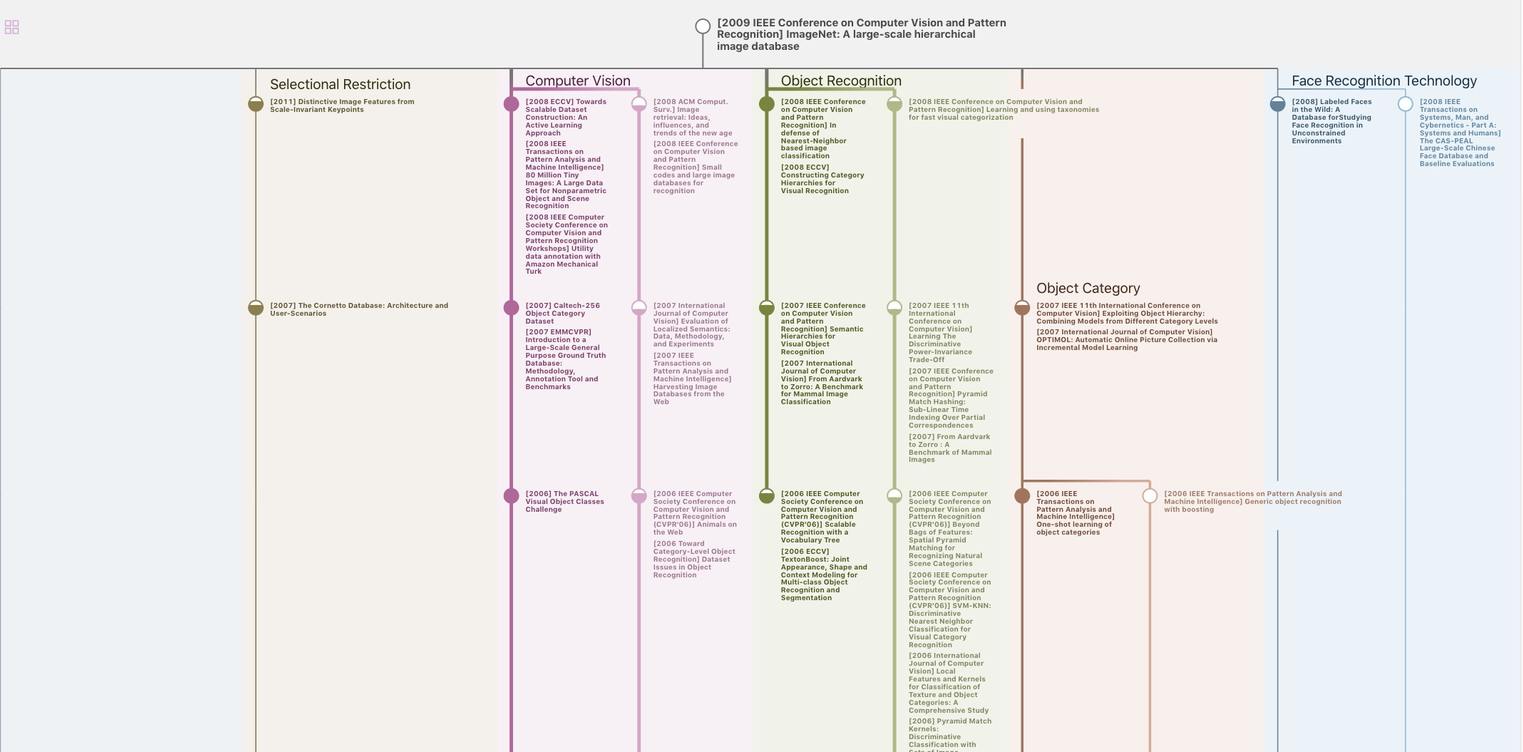
生成溯源树,研究论文发展脉络
Chat Paper
正在生成论文摘要