Meta Perturbed Re-Id Defense.
ICME(2023)
摘要
Adversarial attacks have gained significant attention in object re-identification (Re-Id). However, very few works target defense, and they primarily adopt adversarial training. While adversarial training has been shown to be a major line of defense, we observe that vanilla adversarial training alone does not provide a robust defense against adversarial attacks. Towards this, we propose a novel meta perturbed defense algorithm for Re-Id task. Our contributions are, (i) we introduce anisotropic and isotropic perturbations to design a stochastic neural network, and train it with a novel meta-learning strategy with tasks as vanilla, perturbed, and perturbed-adversarial training; (ii) we show the generalizability of our model against various unseen attacks; and (iii) we derive a novel feature covariance alignment (FCA) loss which gives us a high clean performance while providing robustness against different attacks. Extensive experiments on Market-1501, MSMT17 and VeRi-776 reveal SOTA performance.(1)
更多查看译文
关键词
Re-Id,Adversarial Defense,Perturbation,Meta-Learning
AI 理解论文
溯源树
样例
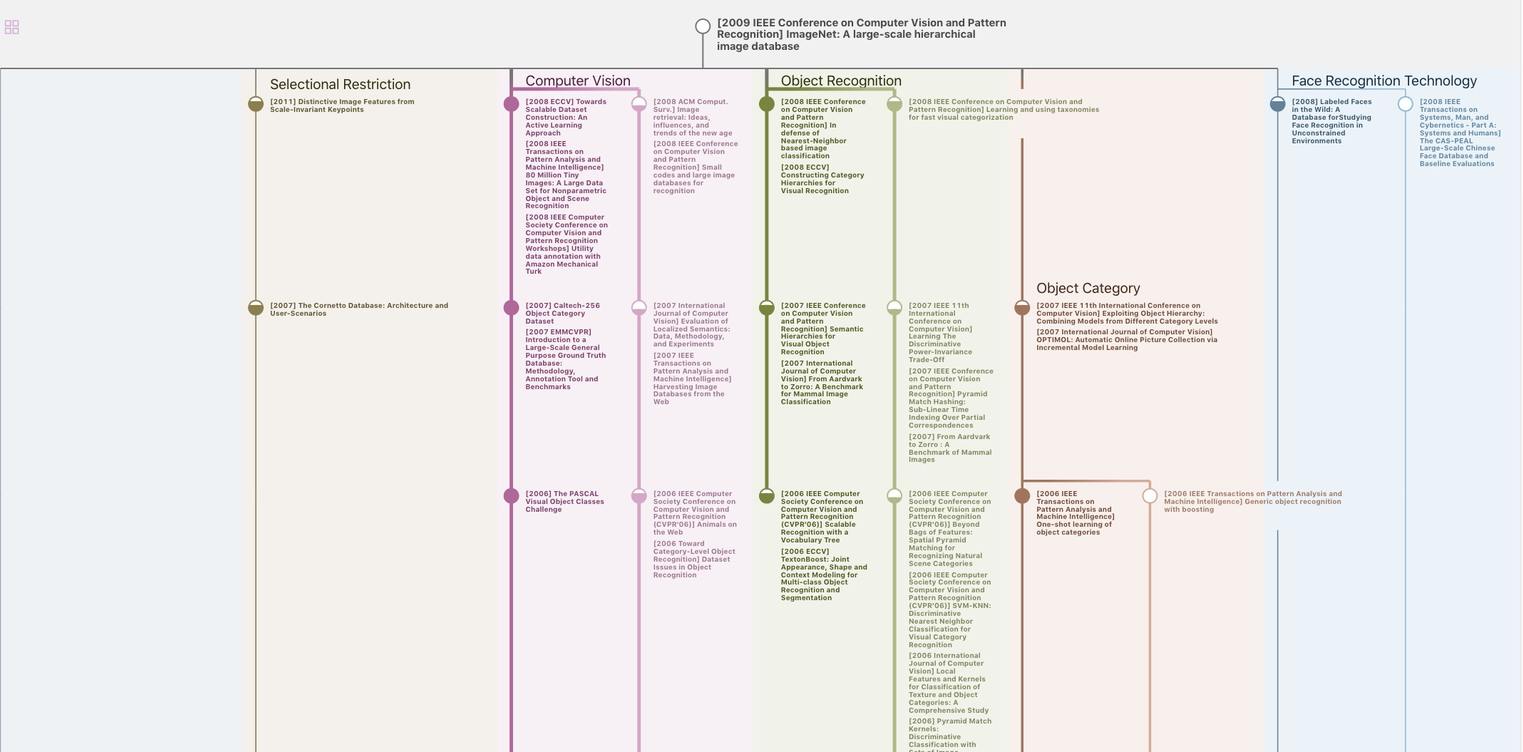
生成溯源树,研究论文发展脉络
Chat Paper
正在生成论文摘要