Self-Supervised Implicit 3D Reconstruction via RGB-D Scans.
ICME(2023)
摘要
Recently, 3D reconstruction methods based on the neural radiance fields have demonstrated remarkable generative performance. However, these methods frequently tend to be resource hungry and are challenging to regulate large low-textured regions in typical indoor scenes. In this work, we analyze and integrate inherent semantic geometry cues for self-supervised 3D reconstruction training via a unified framework of volume rendering and signed distance implicit representations. In contrast to previous neural implicit methods, we simultaneously incorporate the pixel-aligned features and image patches for multi-view consistency, thereby enabling us to depict a large indoor scene from challenging scenarios with rich visual details and large smooth backgrounds. Extensive experiments and comparisons demonstrate that our proposed method has achieved state-of-the-art results by a large margin in various tasks (e.g. actual surface reconstruction, novel view synthesis, and learning a universal scheme in occlusion or distorted regions).
更多查看译文
关键词
Indoor reconstruction,implicit neural rendering,self-supervised guidance
AI 理解论文
溯源树
样例
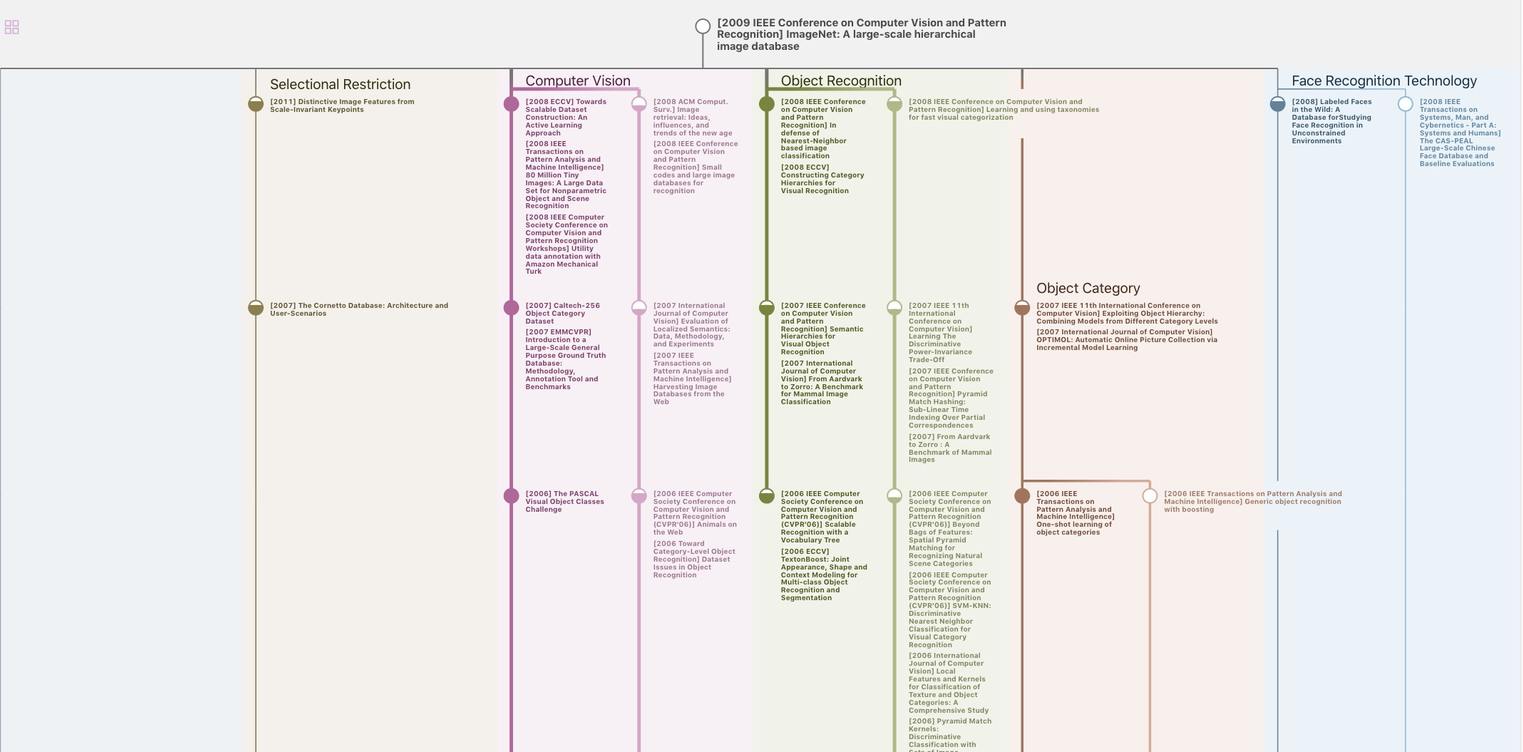
生成溯源树,研究论文发展脉络
Chat Paper
正在生成论文摘要