Discovering Coherent Topics from Urdu Text: A Comparative Study of Statistical Models, Clustering Techniques and Word Embedding.
ICICT(2023)
摘要
The volume of data on the internet is continuously expanding due to the abundance of news sources, journals, blogs, contents, and other online publications. The use of Urdu online has grown significantly, much like other languages. Information retrieval (IR) is getting more challenging as data amount rises. The natural language processing (NLP) technique of topic modelling (TM) is crucial for extracting themes or aspects from text. Although there is a long tradition of TM in both English and other western languages, Urdu falls behind in terms of sophisticated NLP tools and resources for TM. The rich morphology of the Urdu language makes TM a challenging task. In this study, we developed a framework of TM and analysed word embedding, statistical models, and clustering techniques for Urdu documents. The aim of this work is to evaluate and compare three distinct approaches based on the coherence measure of extracted topics. The findings of a thorough experiment and evaluation demonstrate that word embedding fails to extract coherent topics in Urdu language, and that the average coherence measure of topics retrieved by clustering approaches outperforms that discovered through statistical models.
更多查看译文
关键词
topic modeling,coherent topics,LDA,word embedding,seeded-LDA,natural language processing,NMF,K-means
AI 理解论文
溯源树
样例
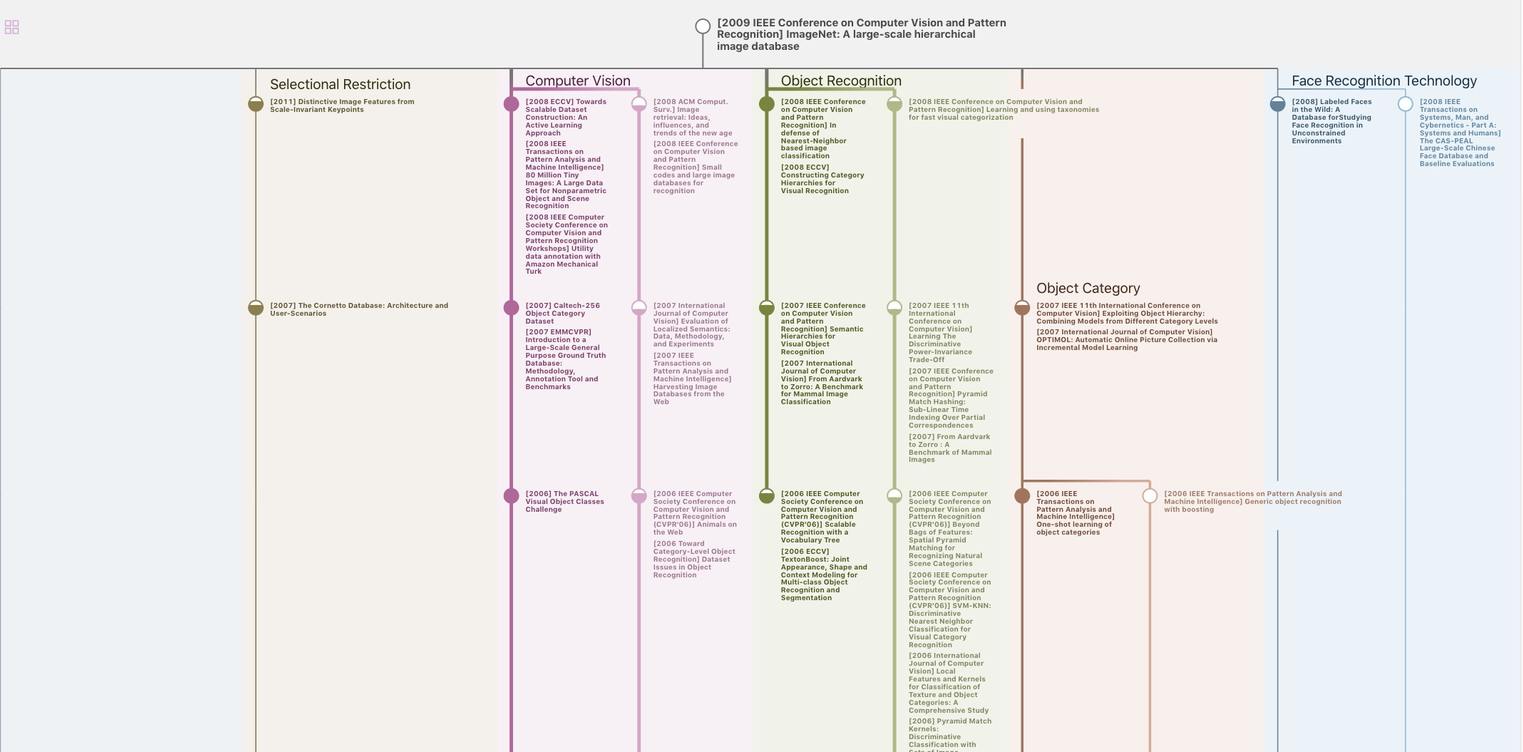
生成溯源树,研究论文发展脉络
Chat Paper
正在生成论文摘要