Adaptive Token Excitation with Negative Selection for Video-Text Retrieval
ARTIFICIAL NEURAL NETWORKS AND MACHINE LEARNING, ICANN 2023, PT VII(2023)
摘要
Video-text retrieval aims to efficiently retrieve videos from large collections based on the given text, whereas methods based on the large-scale pre-trained model have drawn sustained attention recently. However, existing-methods neglect detailed information in video and text, thus failing to align cross-modal semantic features well and leading to performance bottlenecks. Meanwhile, the general training strategy often treats semantically similar pairs as negatives, which provides the model with incorrect supervision. To address these issues, an adaptive token excitation (ATE) model with negative selection is proposed to adaptively refine features encoded by a large-scale pre-trained model to obtain more informative features without introducing additional complexity. In detail, ATE is first advanced to adaptively aggregate and align different events described in text and video using multiple non-linear event blocks. Then a negative selection strategy is exploited to mitigate false negative effects, which stabilizes the training process. Extensive experiments on several datasets demonstrate the feasibility and superiority of the proposed ATE compared to other state-of-the-art methods. The source code of this work can be found in https://mic.tongji.edu.cn.
更多查看译文
关键词
Video-text retrieval,Adaptive token excitation,Negative selection
AI 理解论文
溯源树
样例
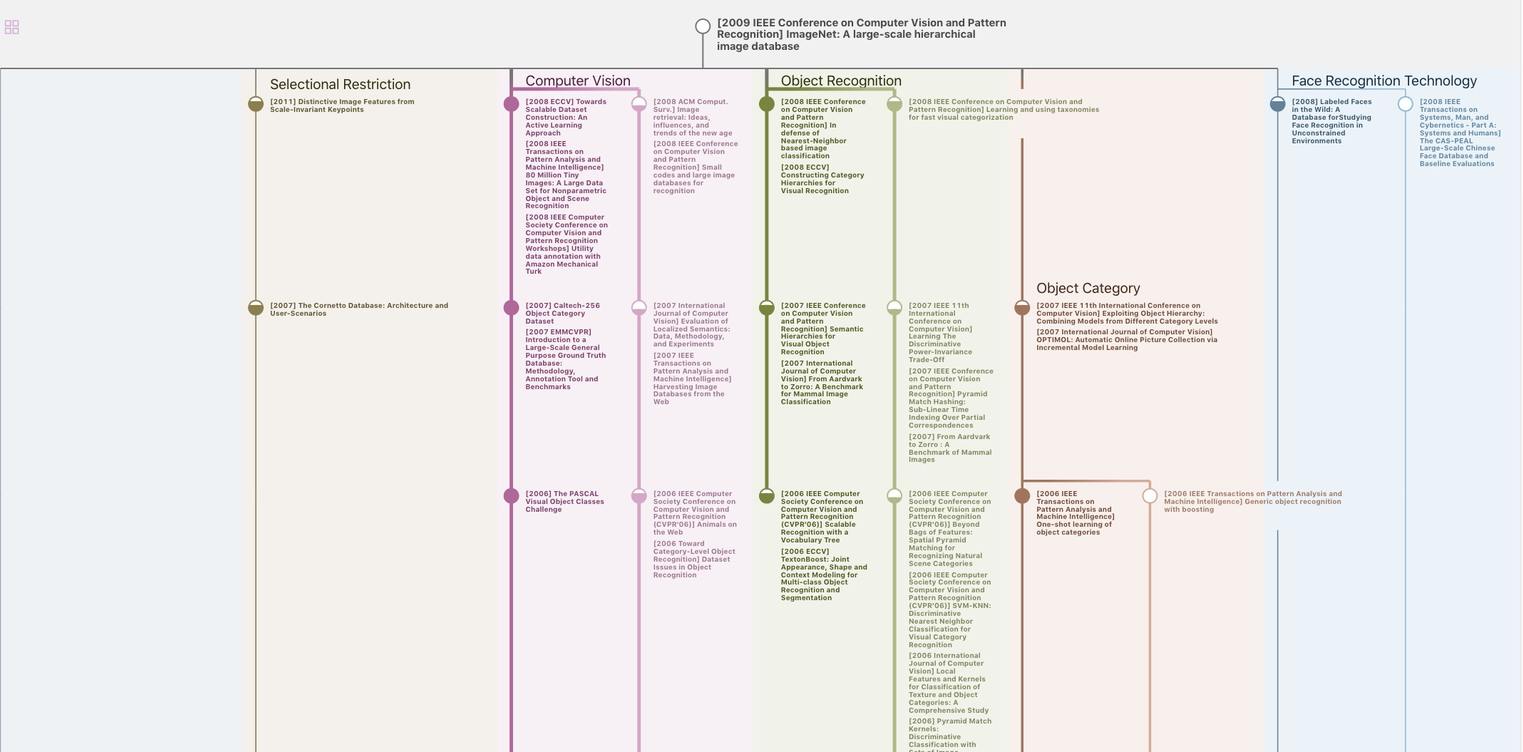
生成溯源树,研究论文发展脉络
Chat Paper
正在生成论文摘要