MBMS-GAN: Multi-Band Multi-Scale Adversarial Learning for Enhancement of Coded Speech at Very Low Rate
ARTIFICIAL NEURAL NETWORKS AND MACHINE LEARNING, ICANN 2023, PT VII(2023)
摘要
Speech coding is to effectively represent speech signals in the form of digital signals. Existing solutions usually have large quantization error when coding at very low rate. This would result in serious spectrum energy distortion of the reconstructed speech, and most of the current approaches do not consider that the distortion is often unevenly distributed across the spectrum. To address these issues, we propose a novel multi-band multi-scale generative adversarial network (MBMS-GAN) for speech coding. In particular, the speech coding is trained in an adversarial manner at different sub-bands and scales to consider both the global and local spectral energy distortion. Besides, a unified codebook matching strategy is designed by integrating the Euclidean distance and cosine similarity to consider both the absolute distance and directions of two vectors in the matching. We very effectiveness of our method on the popular CSTR-VCTK dataset, and the results demonstrate that our method can significantly improve the quality of reconstructed speech at 600 bps by 0.19 in terms of MOS score. Our study has high application value in the scenario of narrow communication channels such as satellite communication.
更多查看译文
关键词
speech coding,adversarial learning,multi-band,multi-scale,codebook matching
AI 理解论文
溯源树
样例
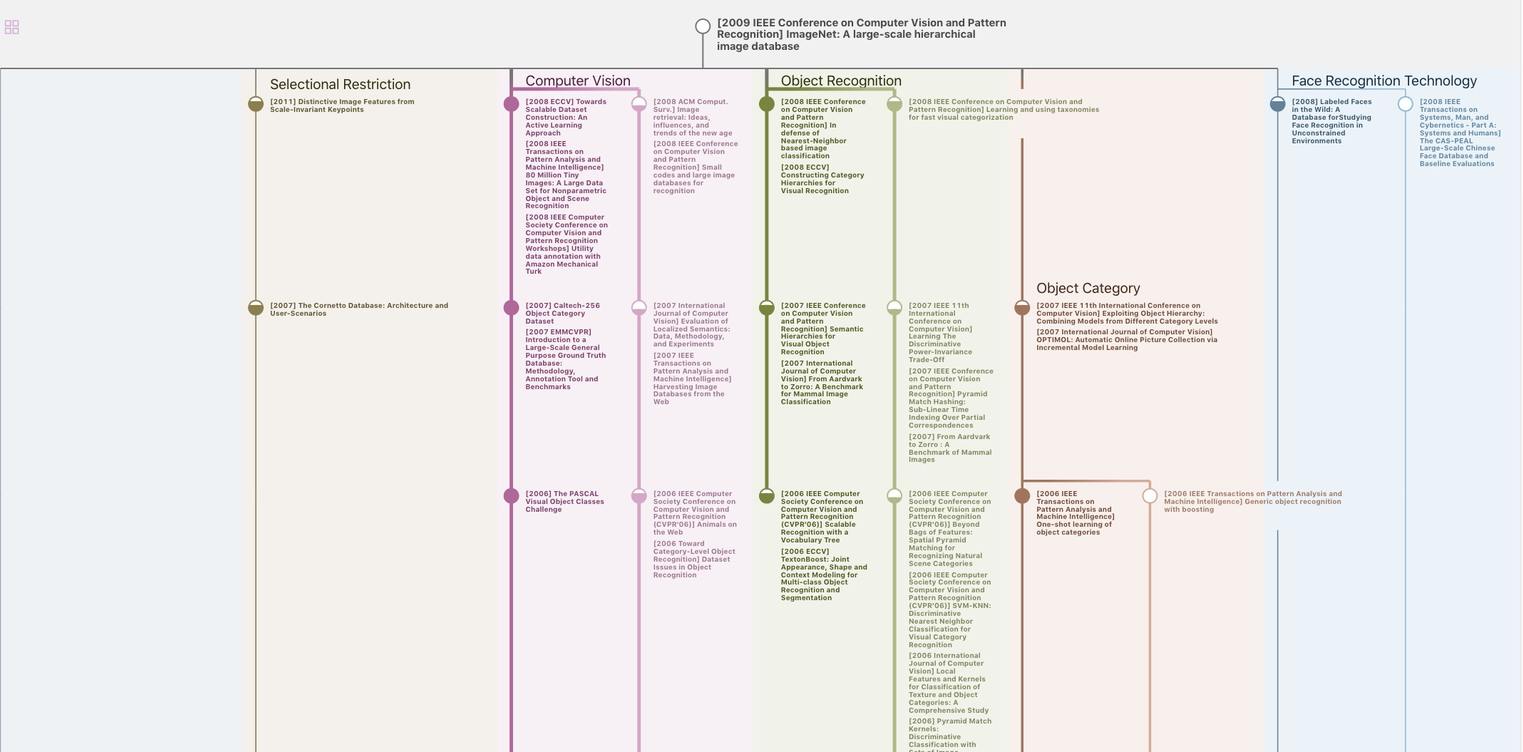
生成溯源树,研究论文发展脉络
Chat Paper
正在生成论文摘要