A Shallow Information Enhanced Efficient Small Object Detector Based on YOLOv5
ARTIFICIAL NEURAL NETWORKS AND MACHINE LEARNING, ICANN 2023, PT VII(2023)
摘要
Shallowinformation is crucial in small object detection. Based on YOLOv5, an efficient small object detection algorithm (ES-YOLO) is proposed to improve identification accuracy using novel shallow feature extraction strategies. First of all, a detection head corresponding to shallow features is used to replace the original detection head corresponding to the deepest features in YOLOv5. Secondly, an attentionmodule is directly added to the output layers of the backbone to filter redundant information and select representative original shallow features. Next, half of the inputs to the SPPF module are processed by the cross-stage partial connection method to reduce model parameters. Finally, the SIoU (SCYLLA-IoU) loss is used during the training stage to ensure fast convergence. Ablation studies are performed on two publicly available small object datasets. Results show that all the proposed models increase the model detection accuracy. Compared with the YOLOv5, the proposed model increases the identification accuracy by 2.4% and 3.5% on the BDD100K and VisDrone datasets, respectively. In addition, compared with the other 8 commonly used or up-to-date one-stage models, the proposed model achieves the best performance in identification accuracy. Source code is released in https://gitee.com/bai-hexiang/es-yolo.
更多查看译文
关键词
Small object detection,Shallow information,YOLOv5
AI 理解论文
溯源树
样例
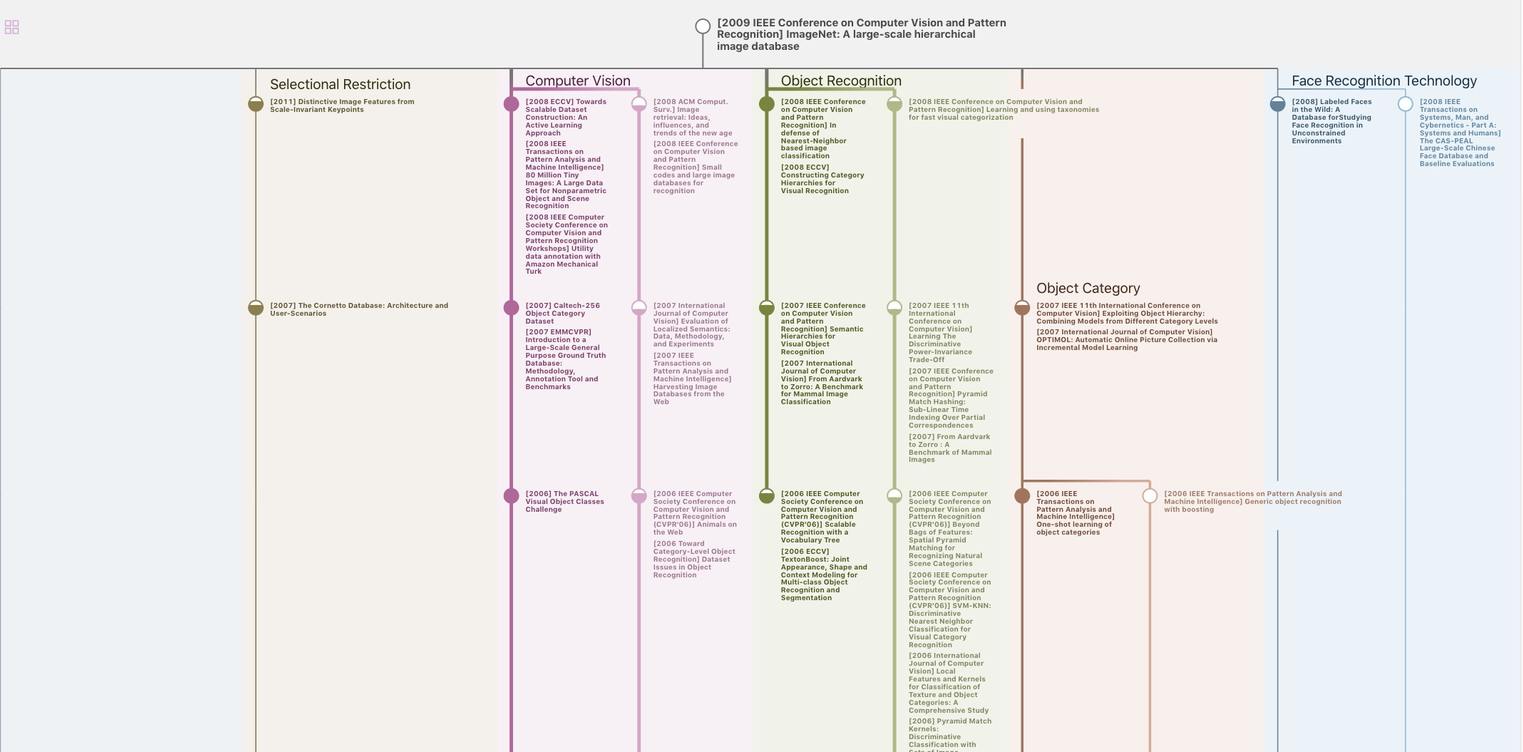
生成溯源树,研究论文发展脉络
Chat Paper
正在生成论文摘要