Hybrid Recommendation System with Graph Neural Collaborative Filtering and Local Self-attention Mechanism.
NCAA (2)(2023)
摘要
As the problem of information overload becomes increasingly serious, traditional recommendation algorithms are difficult to efficiently provide assistance to users. In this context, more and more scholars have applied deep learning methods to recommendation systems, such as deep reinforcement learning, self-attention mechanisms, graph neural collaborative filtering and so on. Experimental results have shown that these methods significantly improve the performance of systems. However, most studies directly apply existing models to recommendation algorithms, wasting a lot of potential features. In recent years, the latest studies have optimized model performance through method fusion. In this paper, we propose a hybrid model NGCF-A that combines graph neural collaborative filtering and self-attention mechanisms. In a system based on graph neural collaborative filtering, local self-attention layers are added to embedding propagation layer, so that we can capturing high level associations between users and items. In addition, we add an implicit feedback transformation method and a dataset splitting strategy that conforms to change in interest, aiming to make better use of features. The experimental results show that this model achieves better performance than traditional graph neural collaborative filtering model, and the new implicit feedback transformation method further optimizes the model.
更多查看译文
关键词
graph neural collaborative filtering,hybrid recommendation system,self-attention
AI 理解论文
溯源树
样例
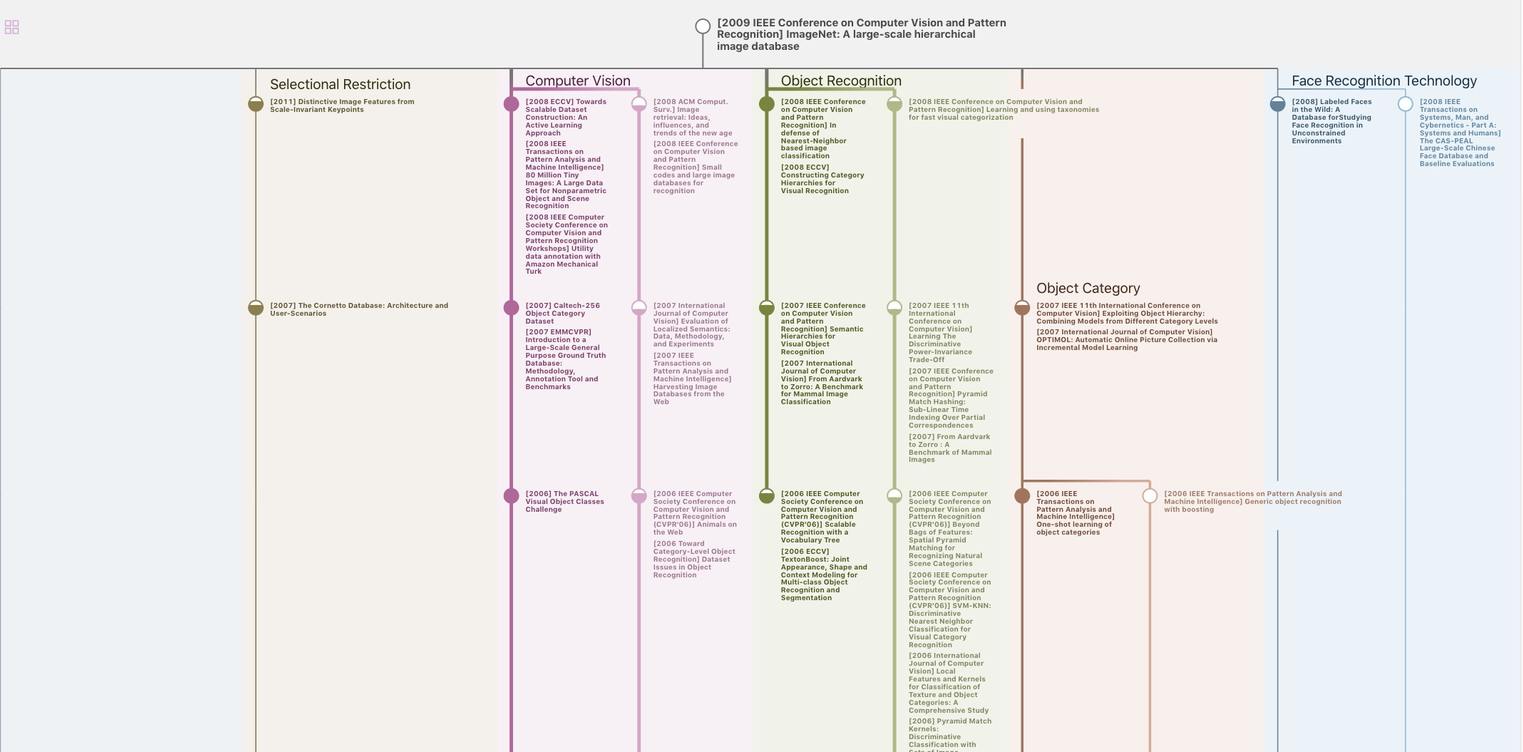
生成溯源树,研究论文发展脉络
Chat Paper
正在生成论文摘要