Enhanced Feature Importance Learning for the Click-Through Rate Prediction.
ICMLC(2023)
摘要
The prediction of click-through rate (CTR) is critical in the recommender system, which aims to predict the probability of the user clicking on the recommended item. Considering the increasing number of features used by modern recommender systems, it is worth learning the feature importance. Inspired by FiBiNet’s introduction of SENet into the recommendation system, we propose an Enhanced Feature Importance (EFI) learning framework for the embedding layer. EFI uses two feature importance vectors to reweight the original feature embedding vector, which can better describe the unique expression of the feature and improve the model’s ability to learn the importance of features. Besides, EFI uses end-to-end training methods and can be a common part of most models in the task of CTR prediction. With extensive experiments on two public datasets, we verified the validity of EFI on the state-of-the-art models.
更多查看译文
AI 理解论文
溯源树
样例
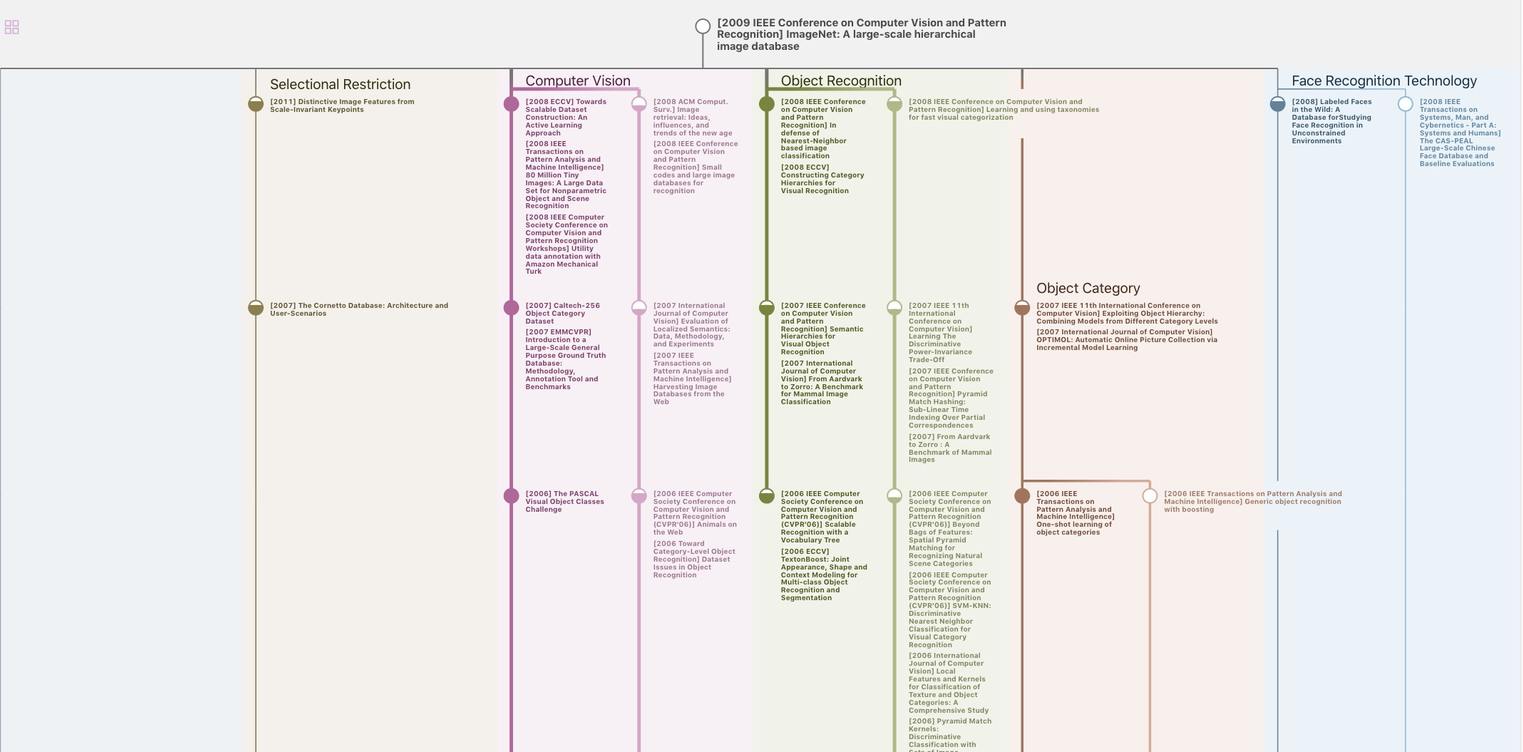
生成溯源树,研究论文发展脉络
Chat Paper
正在生成论文摘要