A Hybrid Search Method for Accelerating Convolutional Neural Architecture Search.
ICMLC(2023)
摘要
The performance evaluation of candidate architectures is a key step in evolution-based neural architecture search (ENAS). Generally, high-fidelity evaluation is desired for finding the optimal architecture but suffers from expensive computational cost while low-fidelity evaluation can reduce search cost but may influence the search performance. In this work, a hybrid search method, HybridNAS, is proposed by synergizing these two evaluation strategies to improve the search effectiveness of ENAS. This method consists of two stages: global exploration and local exploitation. The former applies low-fidelity evaluation to explore the promising architectures efficiently. As for the latter, it exploits the most promising ones identified by the former to generate the better-performancing architectures. Those two stages are iteratively repeated toward the optimal architecture. Extensive experiments on NAS-Bench-101 and NAS-Bench-201 reflect that HybridNAS can achieve comparable accuracy compared to existing popular NAS but at the expense of only 60%-80% computational cost.
更多查看译文
AI 理解论文
溯源树
样例
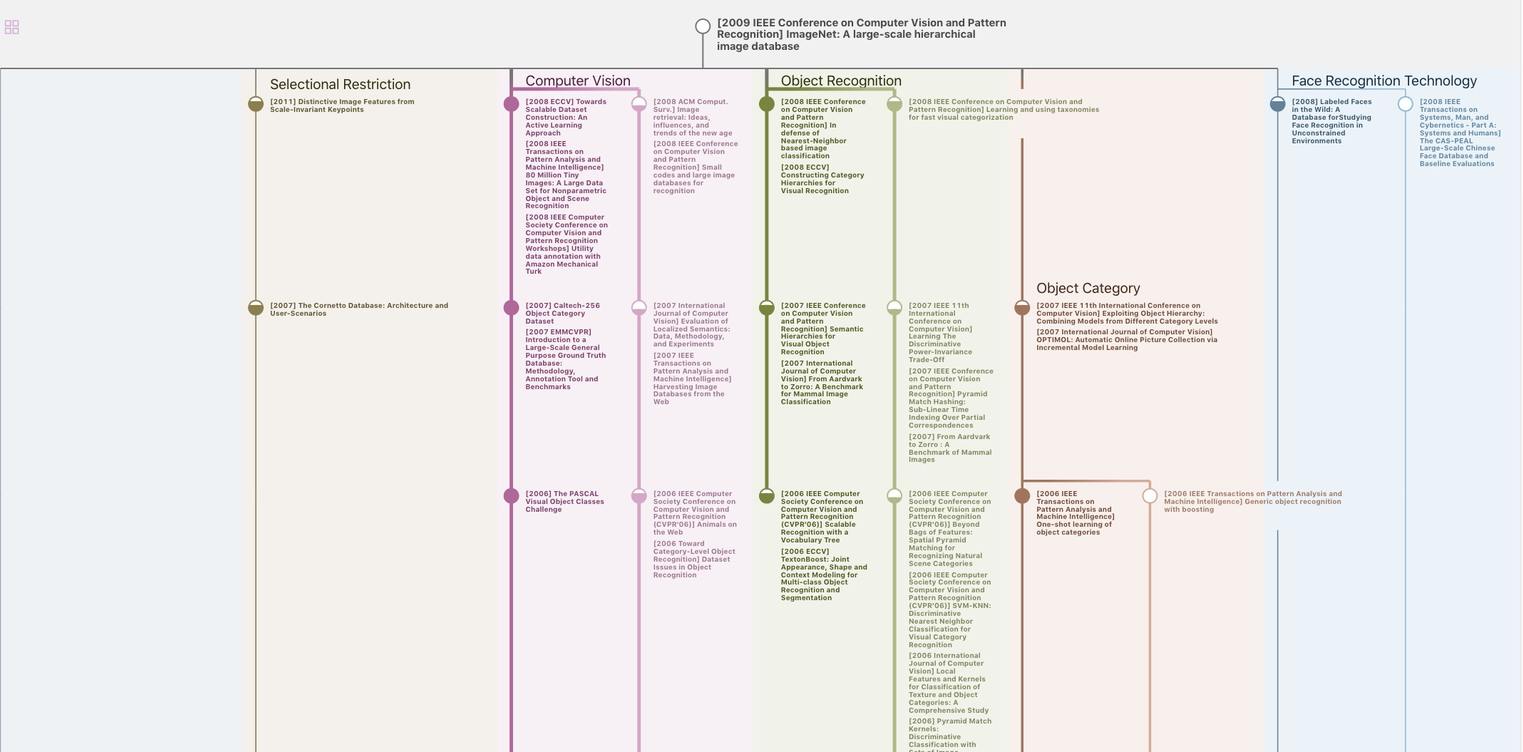
生成溯源树,研究论文发展脉络
Chat Paper
正在生成论文摘要