Interpretable Reinforcement Learning of Behavior Trees.
ICMLC(2023)
摘要
The interpretability of reinforcement learning (RL) algorithms has become one of the significant challenges for artificial intelligence (AI) researchers. Behavior Trees (BTs) have enabled developers to design AI policies visually and comprehend the agent’s behaviors in robotics and computer games. Combining their strengths, researchers have proposed to utilize the RL algorithm to generate BTs to present learned policies automatically. Existing methods are devoted to the incremental generation or modification of pre-designed BTs. These efforts necessitate specialized knowledge and the manual design of initial BTs. In this paper, we present intelligent generation methods that directly represent the policies generated by Q-learning and its derived algorithms in the form of BTs to enhance the interpretability of RL. We investigate the tradeoff between the size and performance of BTs while attaining interpretability, intending to obtain balanced policies that are easy to comprehend and good in performance. Evaluations in several classic OpenAI Gym environments validate the effectiveness of our methods.
更多查看译文
AI 理解论文
溯源树
样例
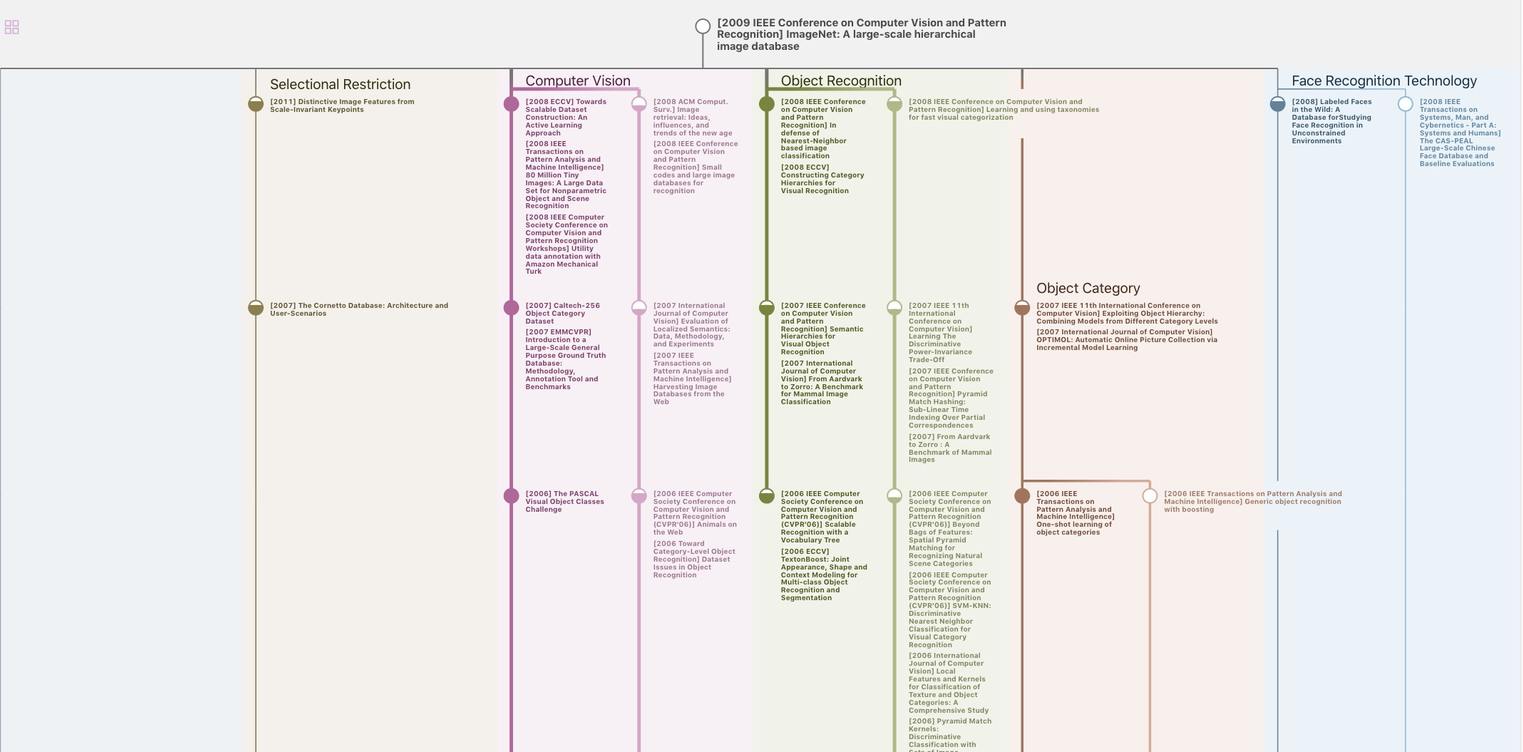
生成溯源树,研究论文发展脉络
Chat Paper
正在生成论文摘要