LBKENet:Lightweight Blur Kernel Estimation Network for Blind Image Super-Resolution
IMAGE ANALYSIS AND PROCESSING, ICIAP 2023, PT II(2023)
摘要
Blind image super-resolution (Blind-SR) is the process of leveraging a low-resolution (LR) image, with unknown degradation, to generate its high-resolution (HR) version. Most of the existing blind SR techniques use a degradation estimator network to explicitly estimate the blur kernel to guide the SR network with the supervision of ground truth (GT) kernels. To solve this issue, it is necessary to design an implicit estimator network that can extract discriminative blur kernel representation without relying on the supervision of ground-truth blur kernels. We design a lightweight (LBKENet) approach for blind super-resolution (Blind-SR) that estimates the blur kernel and restores the HR image based on a deep convolutional neural network (CNN) and a deep super-resolution residual convolutional generative adversarial network. Since the blur kernel for blind image SR is unknown, following the image formation model of the blind super-resolution problem, we first introduce a neural network-based model to estimate the blur kernel. This is achieved by (i) a Super Resolver that, from a low-resolution input, generates the corresponding SR image; and (ii) an Estimator Network generating the blur kernel from the input datum. The output of both models is used in a novel loss formulation. The proposed network is end-to-end trainable. The methodology proposed is substantiated by both quantitative and qualitative experiments. Results on benchmarks demonstrate that our computationally efficient approach (12x fewer parameters than the state-of-the-art models) performs favorably with respect to approaches that have less number of parameters and can be used on devices with limited computational capabilities.
更多查看译文
关键词
blind image super-resolution (Blind-SR),isotropic blur kernel,anisotropic blur kernels
AI 理解论文
溯源树
样例
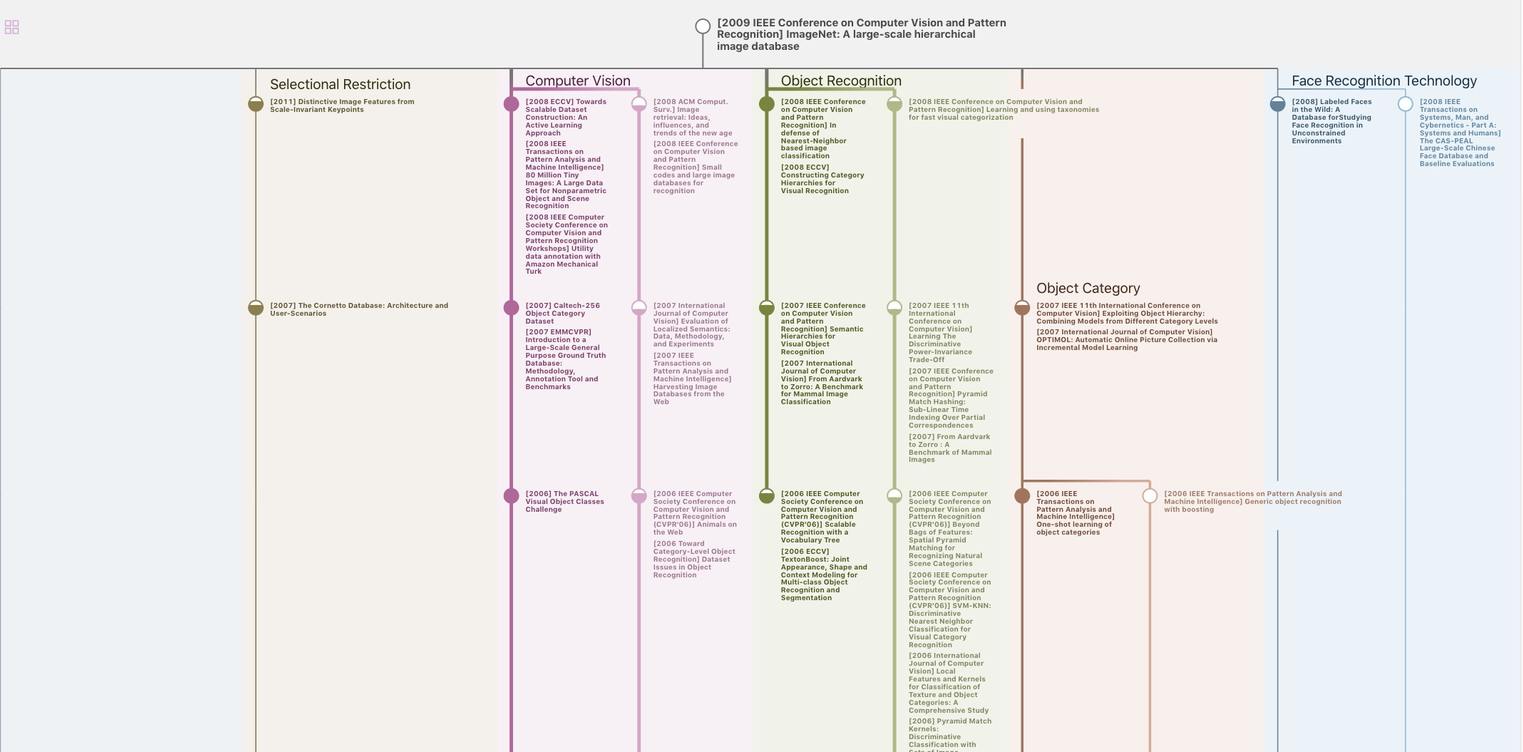
生成溯源树,研究论文发展脉络
Chat Paper
正在生成论文摘要