Breast Cancer Detection Using Transformer and BiLSTM Based Ensemble Learning.
SIU(2023)
摘要
Breast cancer is one of the most common types of cancer among women, and early diagnosis is essential for effective treatment. In recent years, deep learning techniques have shown promising results in solving image classification problems. In this study, labeled image patches obtained from whole slide images in the BACH dataset were used for breast cancer classification. The performances of pre-trained deep learning models for breast cancer classification on labeled image patches were compared. Vision Transformer (ViT) gives better performance with the local features extracted by focusing on the important regions in the image due to the transformer structure and its Attention mechanism. Moreover, the model extending the Xception backbone architecture with Bidirectional Long Short-Term Memory (BiLSTM) layers, XceptionBiLSTM, significantly improved classification performance by learning the spatial relationships between image patches. Furthermore, data augmentation techniques were applied to dataset containing a limited number of image patches, which increased the models' generalization capacity and prevented overfitting. With the proposed architecture, which is the result of combining the predictions of the individual Xception, ViT, and XceptionBiLSTM models used with the ensemble learning approach, 90% accuracy in the hidden test dataset, 95% accuracy and 94.9% F1-Score in the validation dataset were obtained. The results obtained demonstrate the significant potential of the proposed ensemble learning-based architecture for breast cancer classification.
更多查看译文
关键词
breast cancer, image classification, deep learning, BACH dataset, medical imaging
AI 理解论文
溯源树
样例
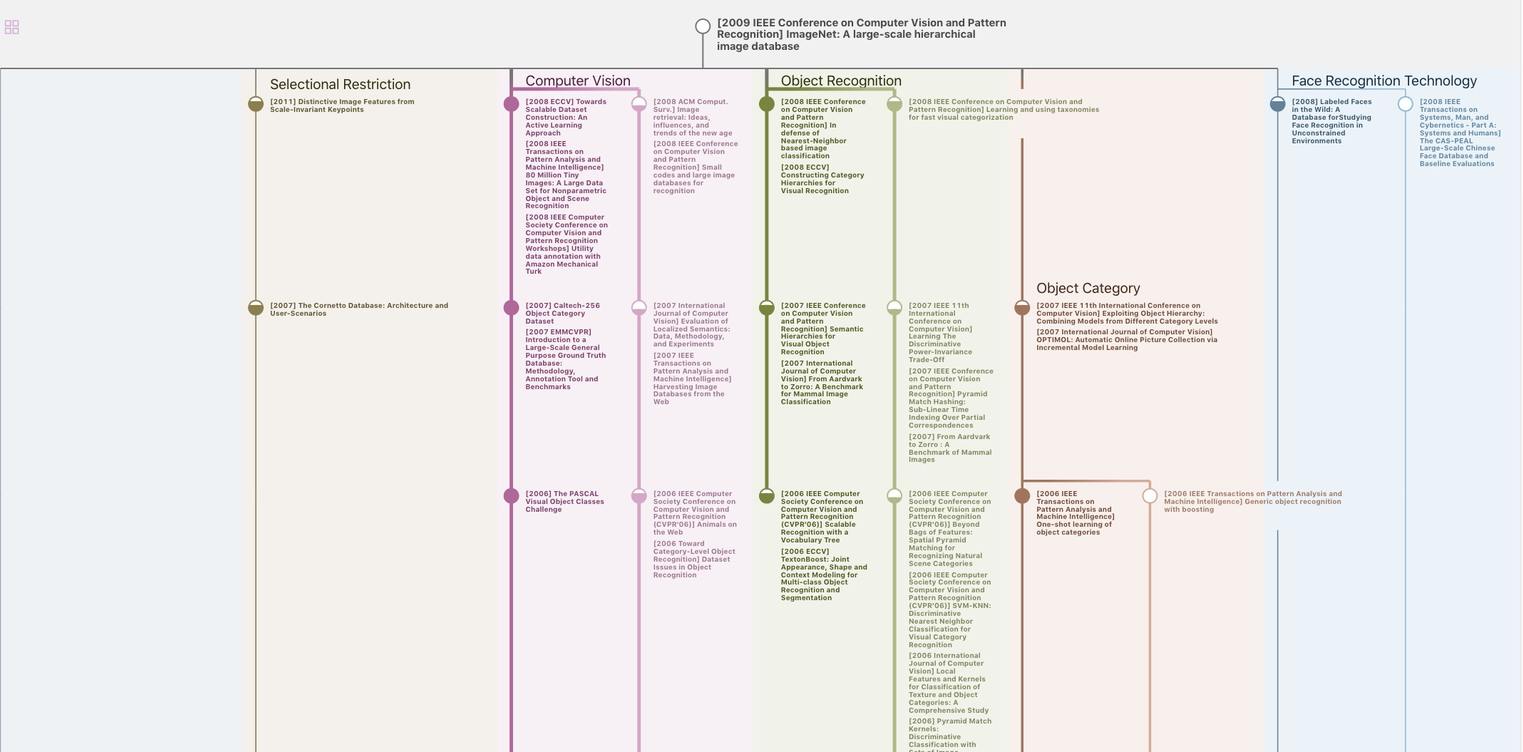
生成溯源树,研究论文发展脉络
Chat Paper
正在生成论文摘要