Performance Analysis of Meta-Learning Based Bayesian Deep Kernel Transfer Methods for Regression Tasks
SIU(2023)
摘要
Meta-learning aims to apply existing models on new tasks where the goal is "learning to learn" so that learning from a limited amount of labeled data or learning in a short amount of time is possible. Deep Kernel Transfer (DKT) is a recently proposed meta-learning approach based on Bayesian framework. DKT's performance depends on the used kernel functions and it has two implementations, namely DKT and GPNet. In this paper, we use a large set of kernel functions on both DKT and GPNet implementations for two regression tasks to study their performances and train them under different optimizers. Furthermore, we compare the training time of both implementations to clarify the ambiguity in terms of which algorithm runs faster for the regression based tasks.
更多查看译文
关键词
Meta-learning,Few-shot learning,Deep Kernel Transfer,Regression,Kernel learning
AI 理解论文
溯源树
样例
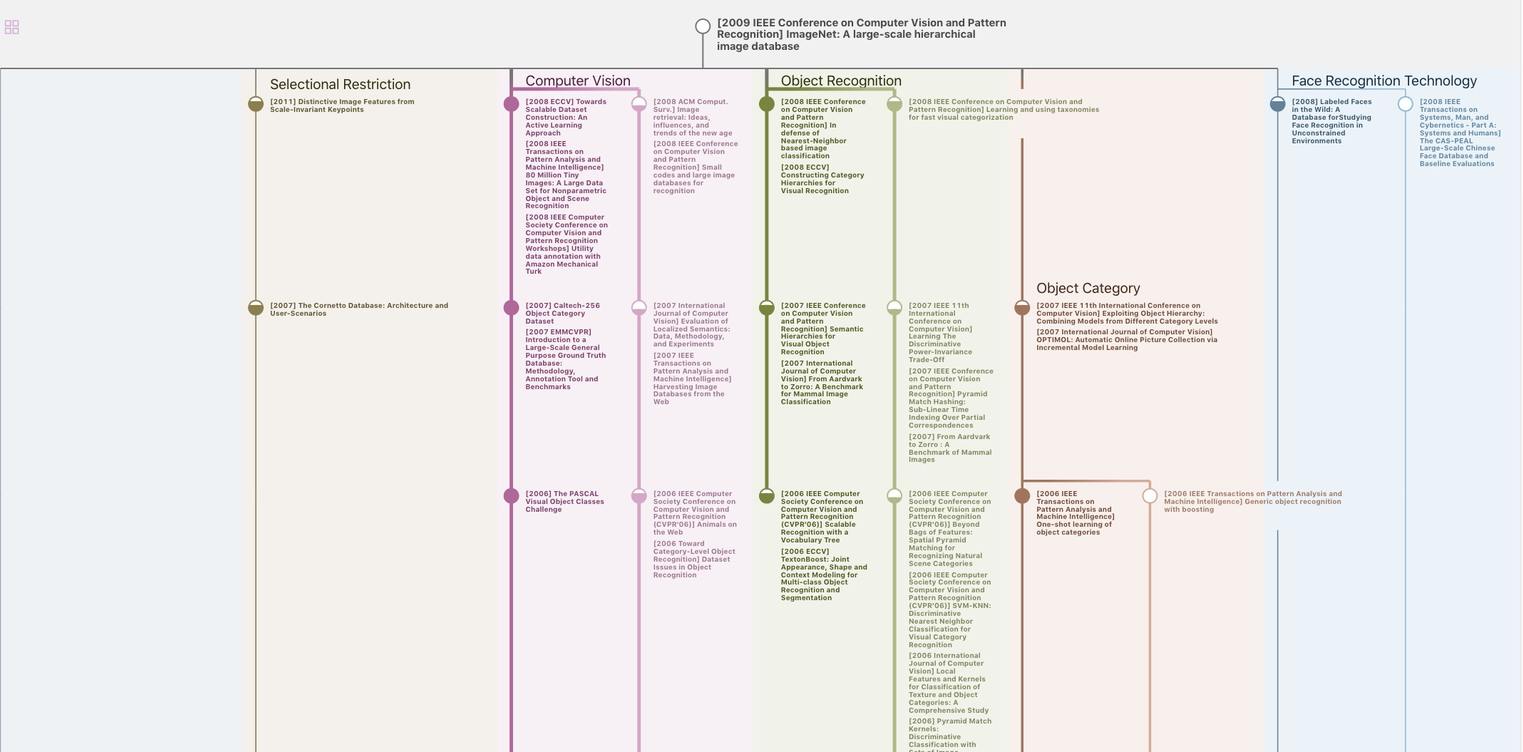
生成溯源树,研究论文发展脉络
Chat Paper
正在生成论文摘要