Cross-Lingual Candidate Retrieval and Re-ranking for Biomedical Entity Linking.
CLEF(2023)
摘要
Biomedical entity linking is an essential building block for various clinical applications and downstream NLP tasks. However, only few annotated biomedical datasets with grounded entity mentions for non-English languages are available for training supervised machine learning models. Moreover, the majority of concept aliases in medical vocabularies are also only available in English. In this work, we consider the problem of linking disease mentions in Spanish clinical case reports to concept identifiers in SNOMED CT, a comprehensive medical terminology system. For these concepts, only a limited number of aliases in the source language are given, but many more can be obtained from other languages and medical vocabularies. We propose a system that utilizes these multilingual aliases to retrieve candidate concepts for a given entity mention and re-ranks retrieved candidates using a trainable cross-encoder. We evaluate our system on the DisTEMIST shared task dataset of the 10 th BioASQ challenge. Our results show that supervised re-ranking outperforms the previously best-performing rule-based system, while requiring much less task-specific hyperparameter tuning. Detailed ablation experiments demonstrate that multilingual aliases are highly beneficial to improve recall during candidate generation, but hardly affect re-ranking performance.
更多查看译文
关键词
cross-lingual,re-ranking
AI 理解论文
溯源树
样例
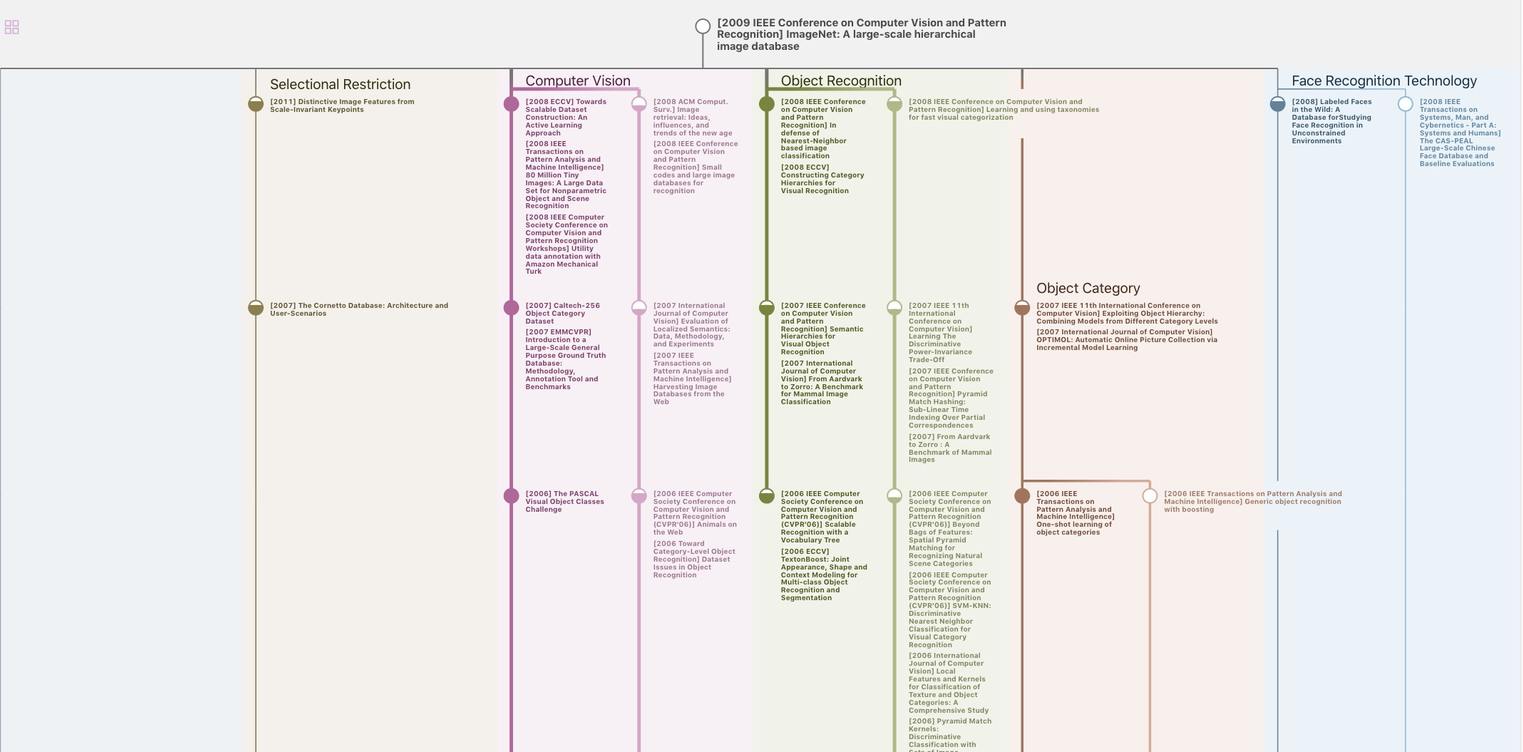
生成溯源树,研究论文发展脉络
Chat Paper
正在生成论文摘要