Complementary Environmental Selection for Evolutionary Many-Objective Optimization.
NCAA (1)(2023)
摘要
In many-objective evolutionary algorithms (MaOEAs), environmental selection is an important operation, which can greatly affect the performance of convergence and distribution of solutions. However, different environmental selection strategies have different preferences. It is difficult to design an appropriate environment selection strategy to balance the convergence and population diversity. To address this issue, this paper proposes a complementary environmental selection strategy for evolutionary many-objective optimization (called CES-MaOEA). Firstly, a dual-population mechanism is utilized. The first population uses the environmental selection of NSGA-III, and the second population employs the environmental selection of radial space division based evolutionary algorithm (RSEA). Through complementary cooperation of two populations, the proposed strategy can make full use of the advantages of the two environmental selection methods. In order to verify the effectiveness of our approach, two well-known benchmark sets including DTLZ and MaF are tested. Performance of CES-MaOEA is compared with five state-of-the-art MaOEAs. Experimental results show that CES-MaOEA achieves competitive performance in terms of convergence and population diversity.
更多查看译文
关键词
evolutionary,optimization,selection,many-objective
AI 理解论文
溯源树
样例
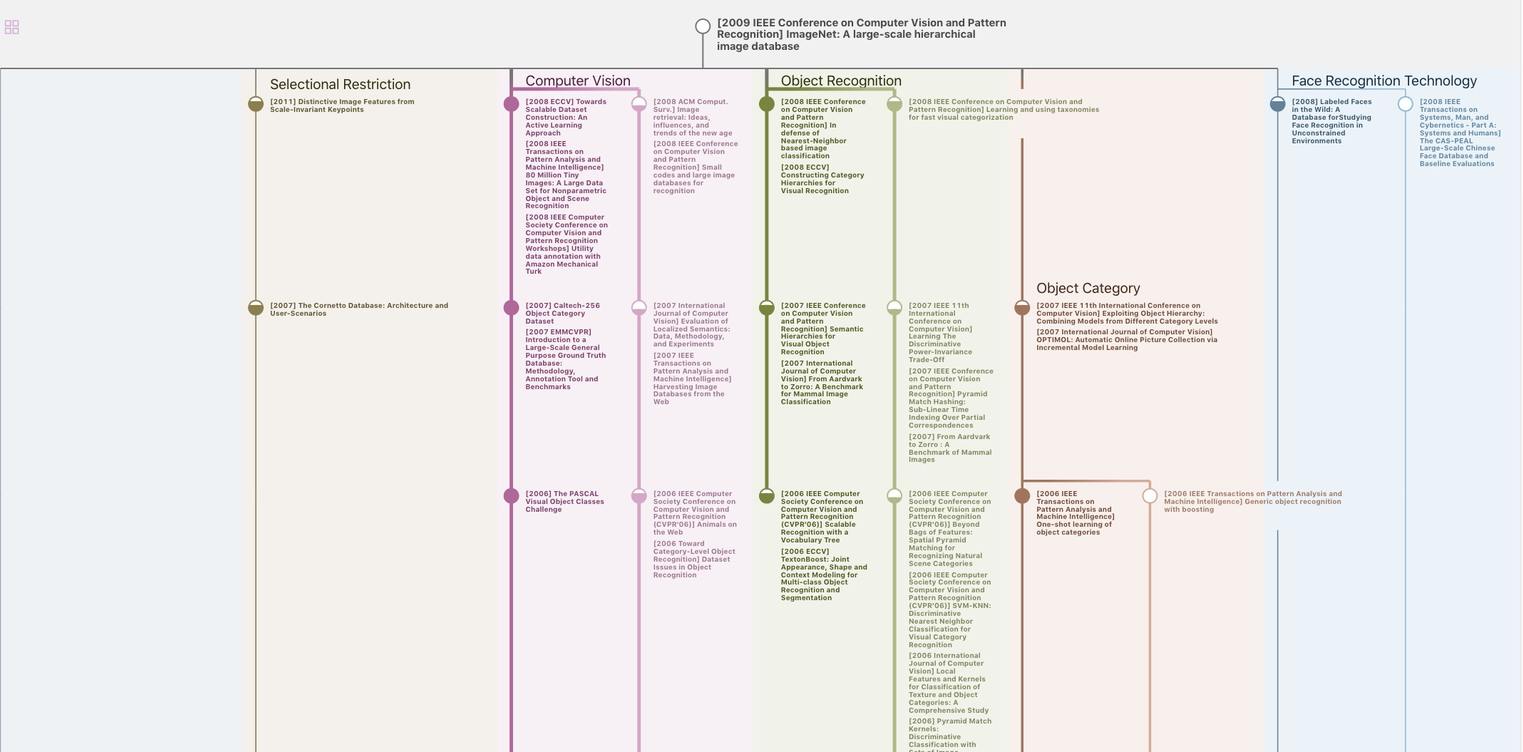
生成溯源树,研究论文发展脉络
Chat Paper
正在生成论文摘要