A Scheme for Robust Federated Learning with Privacy-preserving Based on Krum AGR.
ICCC(2023)
摘要
The sensitive information of participants would be leaked to an untrustworthy server through gradients in federated learning. Encrypted aggregation of uploaded parameters could resolve this issue. However, it brings challenges to the defense of model poisoning attacks in federated learning while solving the privacy problem. To address this issue, a robust federated learning scheme with privacy-preserving (RFLP) is proposed to eliminate the impact of model poisoning attacks while protecting the privacy of participants against untrusted servers. Specifically, an abnormal gradients detecting method is designed to achieve robust federated learning under encrypted aggregation using Pailliar homomorphic encryption. It is based on the concept of Krum aggregation algorithm (AGR), but utilizes privacy-preserving data features, thereby ensuring privacy. To reduce the rounds of communication in robust aggregation, a multidimensional homomorphic encryption approach is constructed. Besides, an aggregated signature authentication method is also constructed to ensure data integrity during transmission. The experiment results show that the training accuracy of RFLP with 10% malicious participants is 11.9% and 15.3% higher than that without robust aggregation.
更多查看译文
关键词
Robust Federated Learning,Homomorphic Encryption,Model Poisoning Attack,Signature Authentication
AI 理解论文
溯源树
样例
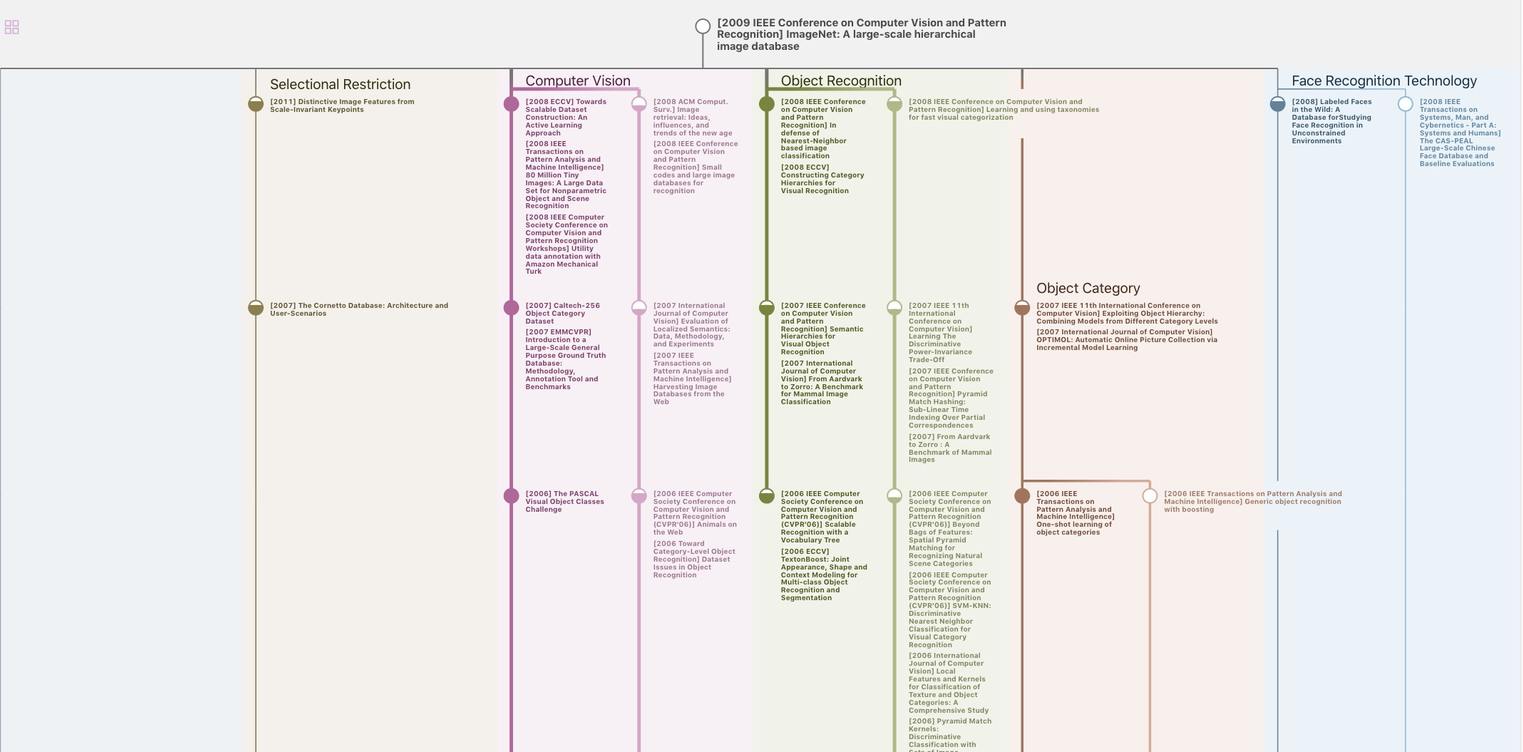
生成溯源树,研究论文发展脉络
Chat Paper
正在生成论文摘要