A Novel Approach of ESN Reservoir Structure Learning for Improved Predictive Performance
ISCC(2023)
摘要
This paper presents a novel method to enhance the predictive performance of the Echo State Network (ESN) model by adopting reservoir topology learning. ESNs are a type of Recurrent Neural Network (RNN) that have demonstrated considerable potential in various applications, but they can be challenging to train and optimize due to their random initialization. To improve the learning capabilities of ESNs and enhance their effectiveness in a broad range of predictive tasks, we utilize a structure learning algorithm. The proposed approach modifies the ESN reservoir's connectivity by applying techniques such as reversing, deleting, and adding new connections. We evaluate our proposal performance using both synthetic and real datasets, and our results indicate that it can substantially improve predictive accuracy compared to traditional ESNs.
更多查看译文
关键词
Echo State Network,Reservoir Computing,Structure Learning,K2 algorithm
AI 理解论文
溯源树
样例
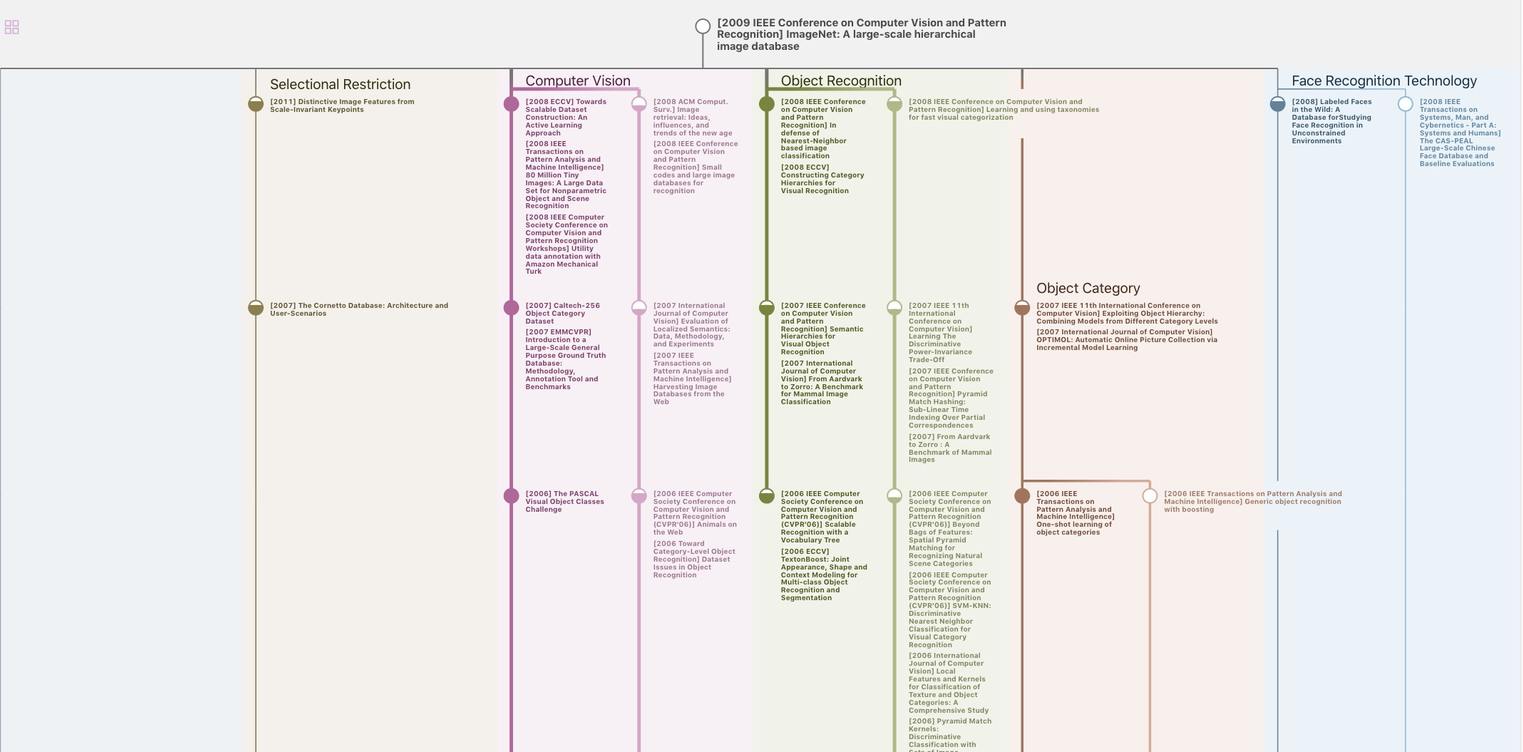
生成溯源树,研究论文发展脉络
Chat Paper
正在生成论文摘要