Learning-Based Congestion Control Assisted by Recurrent Neural Networks for Real-Time Communication.
ISCC(2023)
摘要
In recent years, Real-Time Communication (RTC) has been widely used in many scenarios, and Congestion Control (CC) is one of the important ways to improve the experience of such applications. Accurate bandwidth prediction is the key to CC schemes. However, designing an efficient congestion control scheme with accurate bandwidth prediction is challenging, largely because it is essentially a Partially Observable MDP (POMDP) problem, making it difficult to use traditional hand-crafted methods to solve. We propose a novel hybrid CC scheme LRCC, which combines attention-based Long Short-Term Memory (LSTM) and Reinforcement Learning (RL), realizing more accurate bandwidth prediction and congestion control by adding bandwidth memory information provided by the recurrent neural network to the RL decision-making process. Trace-driven experiments show that our proposed method can significantly reduce packet loss and improve bandwidth utilization in various network scenarios, outperforming baseline methods on overall QoE.
更多查看译文
关键词
Real-Time Communication,Congestion Control,Partially Observable MDP,Attention-Based LSTM,Reinforcement Learning
AI 理解论文
溯源树
样例
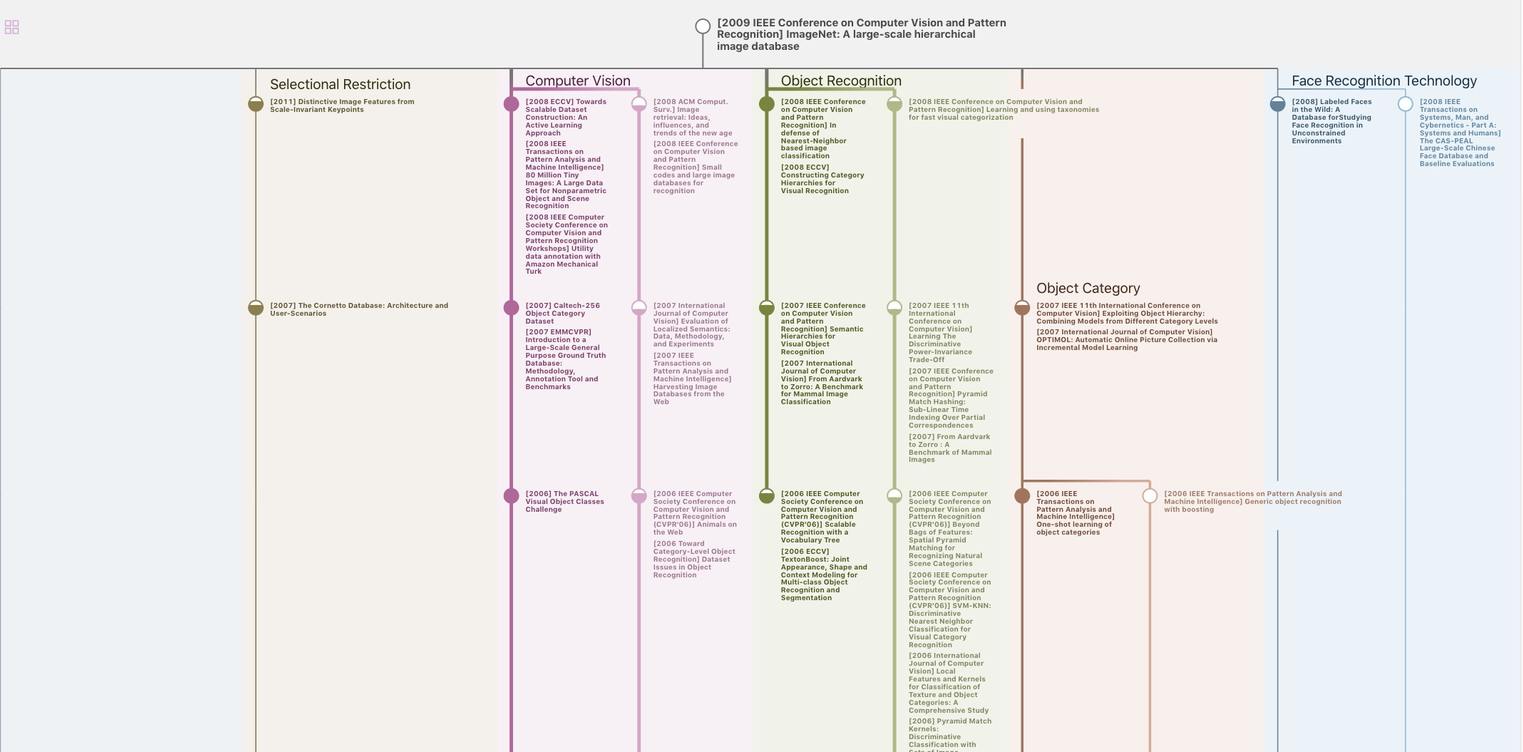
生成溯源树,研究论文发展脉络
Chat Paper
正在生成论文摘要