Feature Relevance in NAT Detection Using Explainable AI.
ISCC(2023)
摘要
Network Address Translation (NAT) was developed to overcome the IPv4 address exhaustion problem. NAT allows multiple devices on a local network to share a single public IP address. However, NAT can result in communication concerns, security flaws, network administration issues, and difficulties locating network faults. This paper addresses the limitations of existing NAT detection techniques through adoption of an innovative machine learning-based approach. The NAT detection model achieves promising results by utilizing novel traffic features. The current techniques used in NAT detection face challenges in terms of explainability and transparency. To address this issue, our proposed method integrates explainable artificial intelligence (XAI) techniques to increase the transparency and interpretability of NAT detection models, thereby improving their efficiency and effectiveness. The results obtained from explain ability highlight the significance of incorporating new features in NAT detection. Furthermore, explainable results indicate that the presence of NAT is closely correlated with specific features.
更多查看译文
关键词
Network Address Translation,Traffic Analysis,Obfuscation,Machine Learning,Explainable AI
AI 理解论文
溯源树
样例
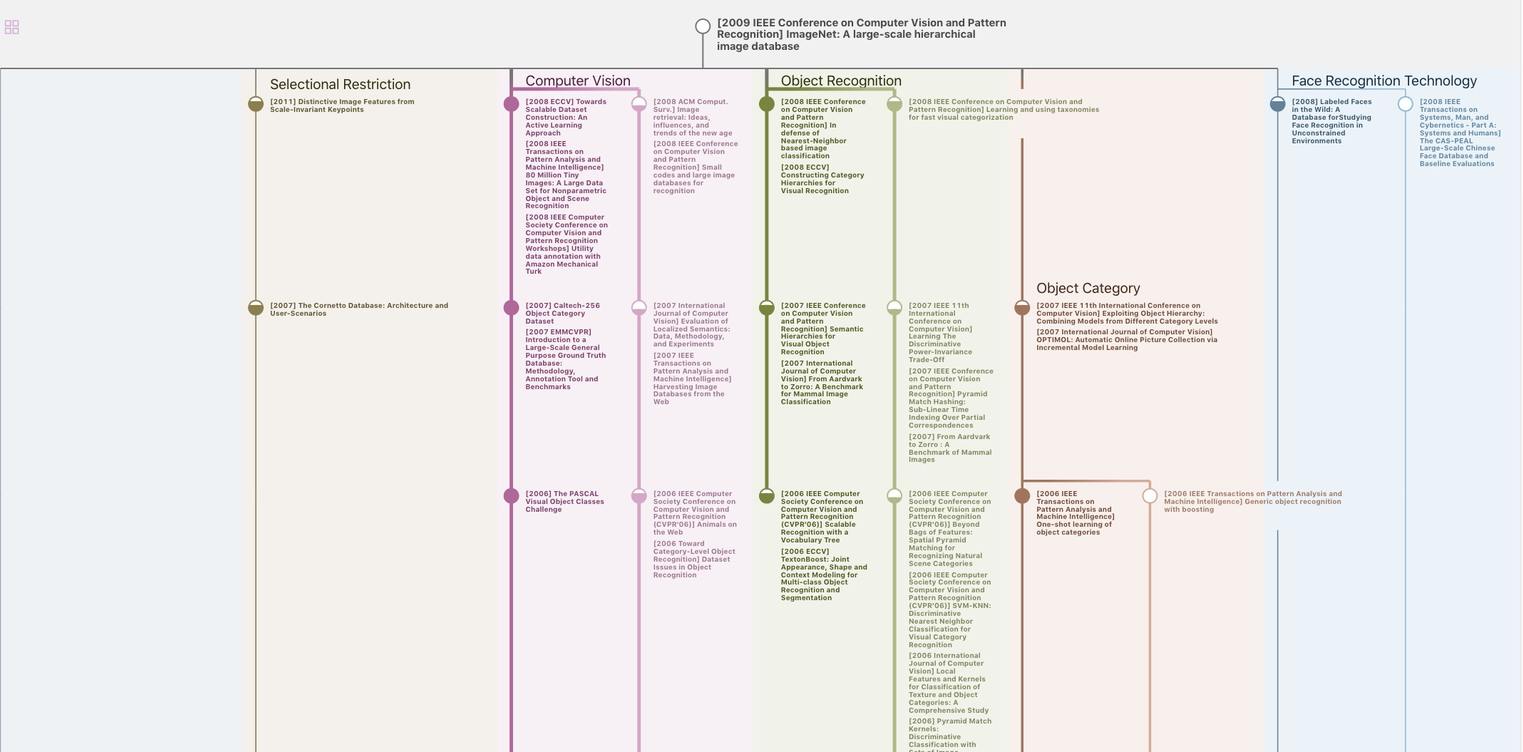
生成溯源树,研究论文发展脉络
Chat Paper
正在生成论文摘要