RGB-2-Hyper-Spectral Image Reconstruction for Food Science Using Encoder/Decoder Neural Architectures.
ISCC(2023)
摘要
Hyper-spectral imaging captures spatial and spectral information of a subject. This is used for the identification of substances within a scene, and food analysis. Presented is an investigation into the capabilities of encoder/decoder deep learning architectures for hyper-spectral image reconstruction from RGB images. For this analysis state-of-the-art (SOTA) techniques for hyper-spectral image reconstruction and other architectures from different fields have been used. Our approach examines a food science case study, using a CPU-based server and different accelerators. An in-house multi-sensor setup was used to capture the dataset which contains hyper-spectral images of twenty slices of different Spanish Ham in the range of 400-100∼nm and their analogous RGB images. The results show no degradation in the output when moving outside of the visual range. This study shows that the SOTA methods for reconstructing from RGB do not produce the most accurate reconstruction of the spectral domain within the range of 400-1000∼nm.
更多查看译文
关键词
Hyper-spectral image reconstruction,RGB,deep learning,GPU accelerators
AI 理解论文
溯源树
样例
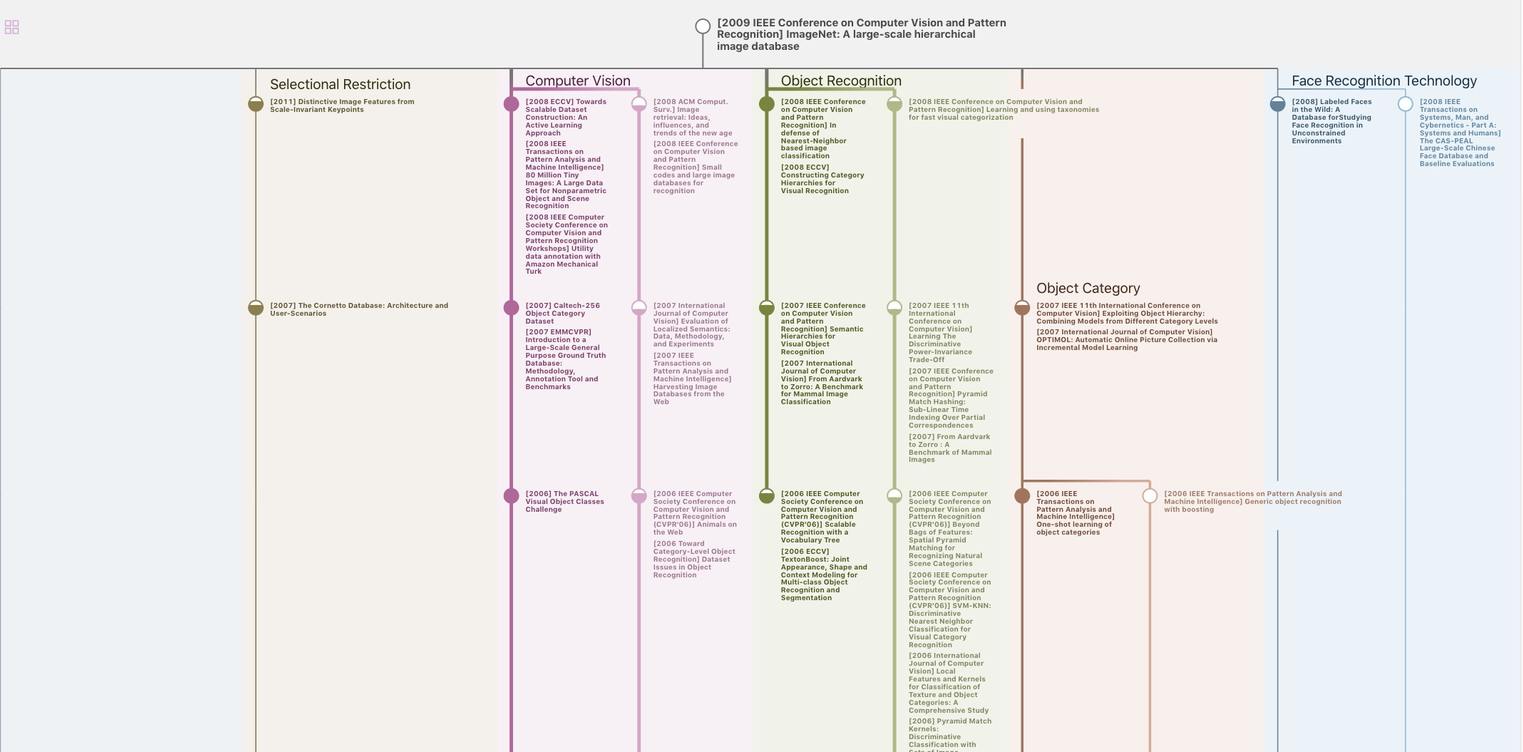
生成溯源树,研究论文发展脉络
Chat Paper
正在生成论文摘要