A Deep Learning Approach For Airport Runway Detection and Localization From Satellite Imagery.
ISCC(2023)
摘要
The US lacks a complete national database of private prior permission required airports due to insufficient federal requirements for regular updates. The initial data entry into the system is usually not refreshed by the Federal Aviation Administration (FAA) or local state Department of Transportation. However, outdated or inaccurate information poses risks to aviation safety. This paper suggests a deep learning (DL) approach using Google Earth satellite imagery to identify and locate airport landing sites. The study aims to demonstrate the potential of DL algorithms in processing satellite imagery and improve the precision of the FAA's runway database. We evaluate the performance of Faster Region-based Convolutional Neural Networks using advanced backbone architectures, namely Resnet101 and Resnet-X152, in the detection of airport runways. We incorporate negative samples, i.e., highways images, to enhance the performance of the model. Our simulations reveal that Resnet-X152 outperformed Resnet101 achieving a mean average precision of 76%.
更多查看译文
关键词
Machine learning,Object detection,Airport runway detection
AI 理解论文
溯源树
样例
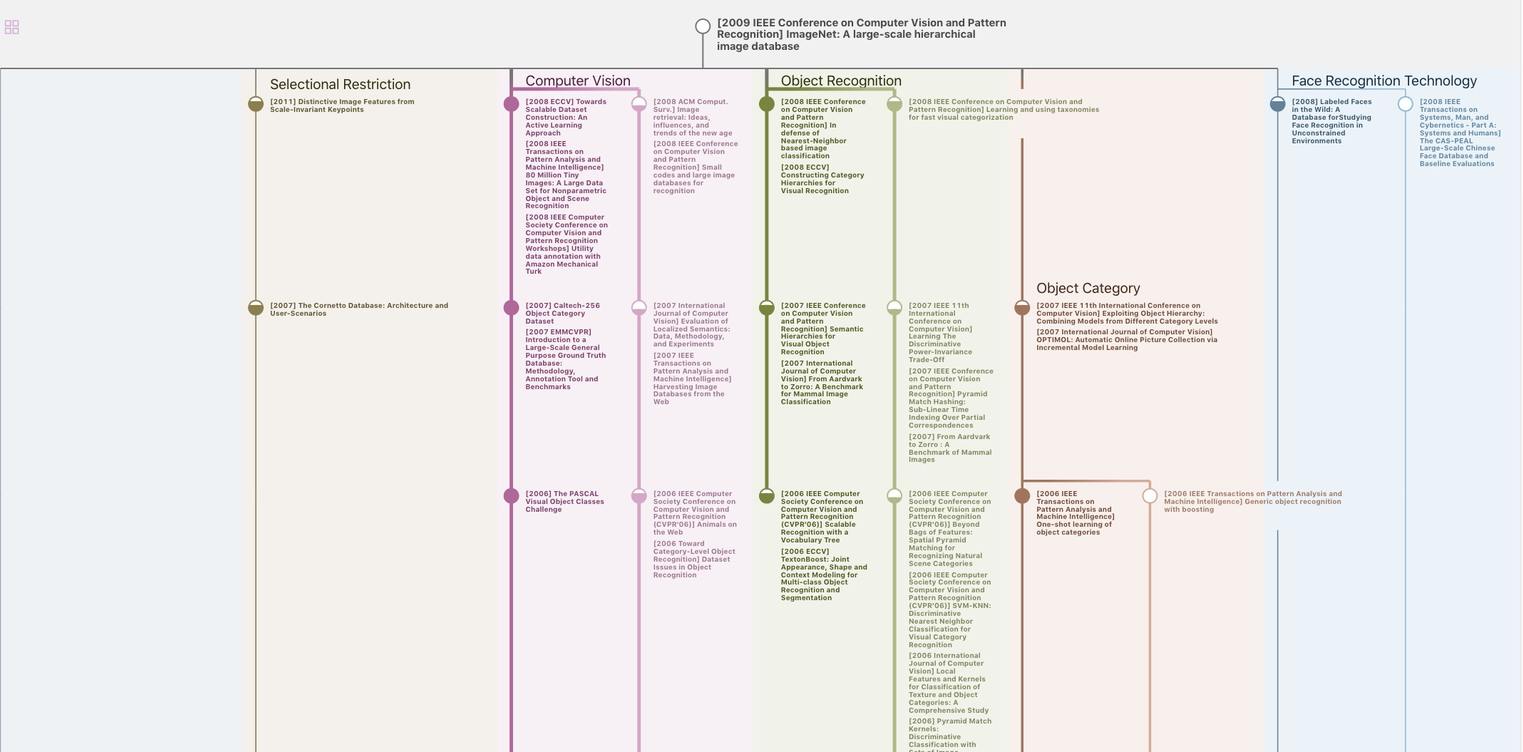
生成溯源树,研究论文发展脉络
Chat Paper
正在生成论文摘要