Anomaly Detection in Heterogeneous Time Series Data for Server-Monitoring Tasks.
ISCC(2023)
摘要
When conducting anomaly detection on server monitoring data, it is important to consider the heterogeneity of the data, which is characterized by the diverse and irregular nature of events. The event values can vary widely, encompassing both continuous and discrete values, and there may be a multitude of randomly occurring events. However, many commonly used anomaly detection methods tend to overlook or discard this heterogeneous data, resulting in a significant loss of valuable information. As such, we propose a novel method, called Heterogeneous Time Series Anomaly Detection (HTSAD), to overcome this difficulty. The approach introduces event gates in the Long Short-Term Memory (LSTM) model while using unsupervised learning to overcome the challenges mentioned above. The results of our experiments on real-world datasets show that HTSAD could achieve an f-score of 0.958, which demonstrates the effectiveness of our approach in detecting anomalies in heterogeneous time series data.
更多查看译文
关键词
heterogeneous,time series,anomaly detection,event gate
AI 理解论文
溯源树
样例
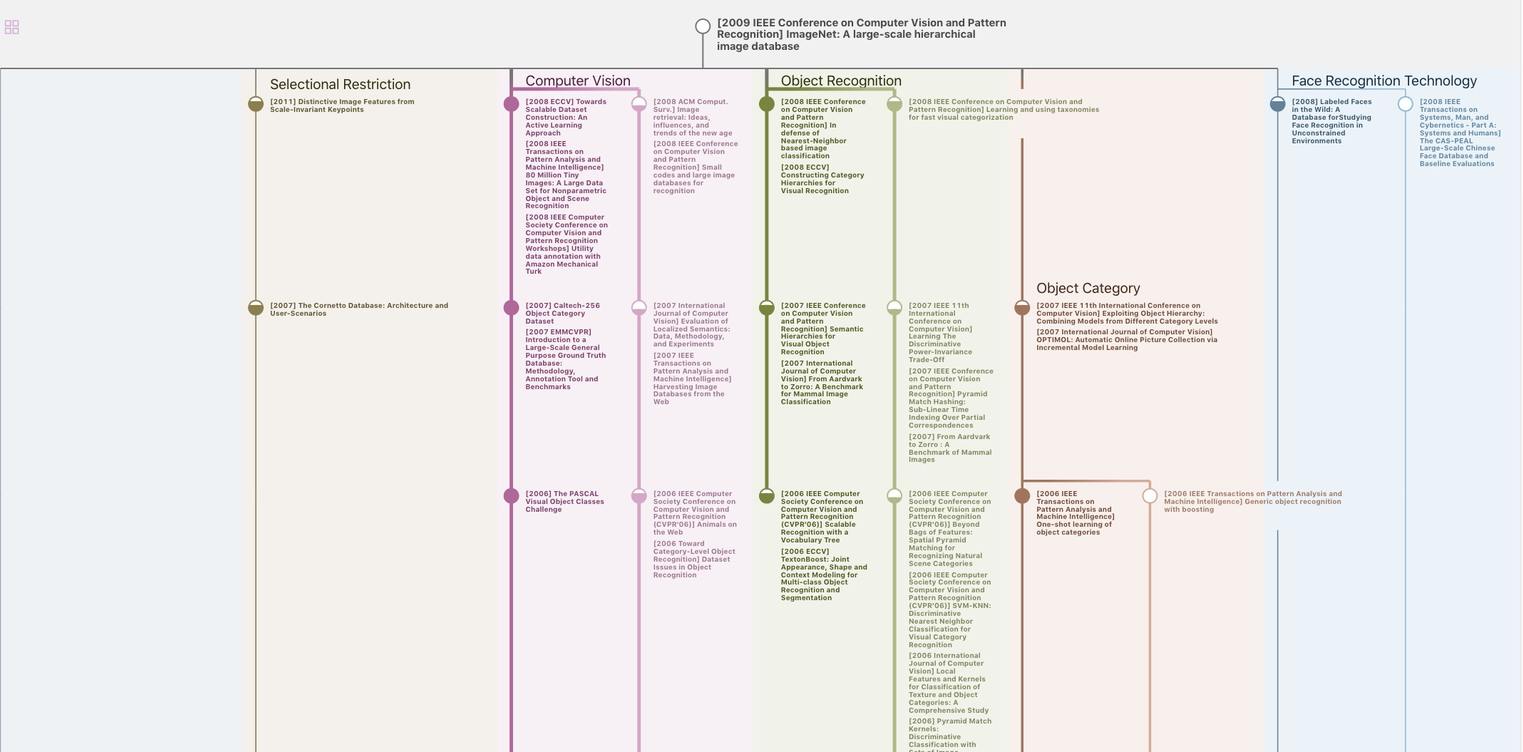
生成溯源树,研究论文发展脉络
Chat Paper
正在生成论文摘要