REFRESH: Responsible and Efficient Feature Reselection guided by SHAP values
PROCEEDINGS OF THE 2023 AAAI/ACM CONFERENCE ON AI, ETHICS, AND SOCIETY, AIES 2023(2023)
摘要
Feature selection is a crucial step in building machine learning models. This process is often achieved with accuracy as an objective, and can be cumbersome and computationally expensive for large-scale datasets. Several additional model performance characteristics such as fairness and robustness are of importance for model development. As regulations are driving the need for more trustworthy models, deployed models need to be corrected for model characteristics associated with responsible artificial intelligence. When feature selection is done with respect to one model performance characteristic (eg. accuracy), feature selection with secondary model performance characteristics (eg. fairness and robustness) as objectives would require going through the computationally expensive selection process from scratch. In this paper, we introduce the problem of feature reselection, so that features can be selected with respect to secondary model performance characteristics efficiently even after a feature selection process has been done with respect to a primary objective. To address this problem, we propose REFRESH, a method to reselect features so that additional constraints that are desirable towards model performance can be achieved without having to train several new models. REFRESH's underlying algorithm is a novel technique using SHAP values and correlation analysis that can approximate for the predictions of a model without having to train these models. Empirical evaluations on three datasets, including a large-scale loan defaulting dataset show that REFRESH can help find alternate models with better model characteristics efficiently. We also discuss the need for reselection and REFRESH based on regulation desiderata.
更多查看译文
关键词
fairness,robustness,explainability
AI 理解论文
溯源树
样例
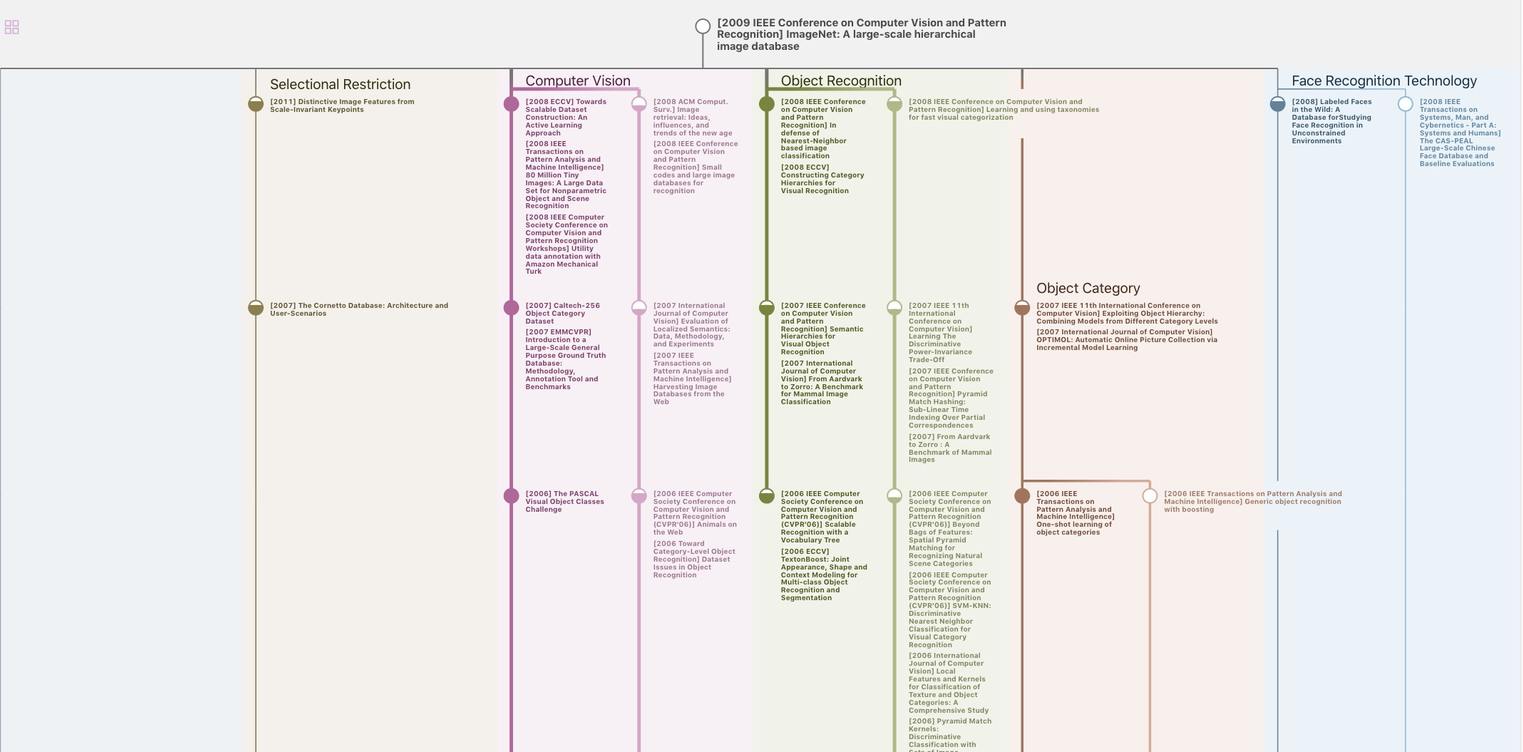
生成溯源树,研究论文发展脉络
Chat Paper
正在生成论文摘要