Not So Fair: The Impact of Presumably Fair Machine Learning Models
PROCEEDINGS OF THE 2023 AAAI/ACM CONFERENCE ON AI, ETHICS, AND SOCIETY, AIES 2023(2023)
摘要
When bias mitigation methods are applied to make fairer machine learning models in fairness-related classification settings, there is an assumption that the disadvantaged group should be better off than if no mitigation method was applied. However, this is a potentially dangerous assumption because a "fair" model outcome does not automatically imply a positive impact for a disadvantaged individual-they could still be negatively impacted. Modeling and accounting for those impacts is key to ensure that mitigated models are not unintentionally harming individuals; we investigate if mitigated models can still negatively impact disadvantaged individuals and what conditions affect those impacts in a loan repayment example. Our results show that most mitigated models negatively impact disadvantaged group members in comparison to the unmitigated models. The domain-dependent impacts of model outcomes should help drive future bias mitigation method development.
更多查看译文
关键词
fairness,impact,machine learning,synthetic data
AI 理解论文
溯源树
样例
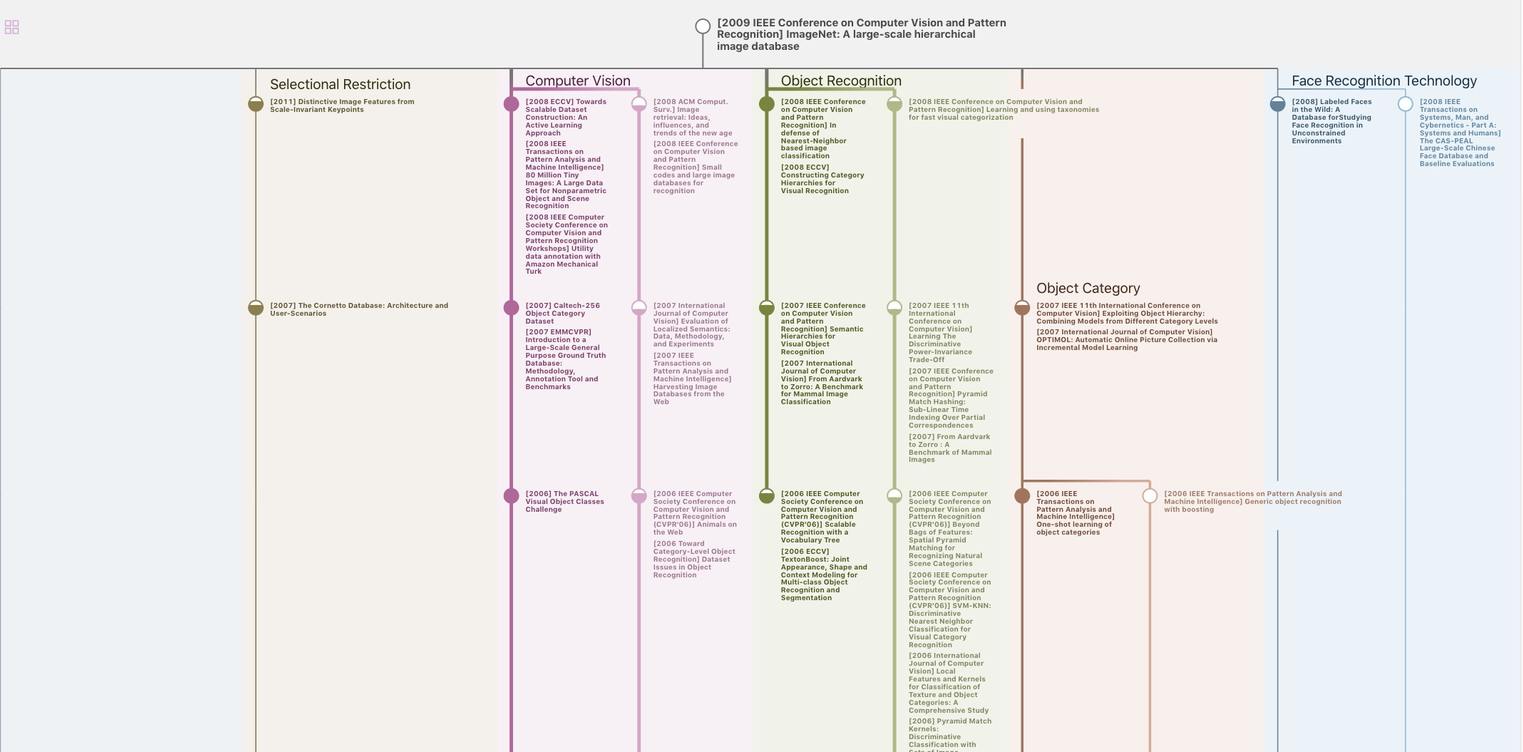
生成溯源树,研究论文发展脉络
Chat Paper
正在生成论文摘要