Performance-Aware Energy-Efficient GPU Frequency Selection using DNN-based Models
PROCEEDINGS OF THE 52ND INTERNATIONAL CONFERENCE ON PARALLEL PROCESSING, ICPP 2023(2023)
摘要
Energy efficiency will be important in future accelerator-based HPC systems for sustainability and to improve overall performance. This study proposes a deep neural network (DNN)-based learning model for execution time and power consumption of workloads across GPUs DVFS design space. Micro-architectural data obtained by running SPEC-ACCEL, DGEMM, and STREAM benchmarks are used for model training. These features are consistent for a workload unaffected by frequency and input size reducing the data required significantly. For real-world applications - LAMMPS, NAMD, GRO-MACS, LSTM, BERT, and ResNet50 power and time models show 89% - 98% accuracy on NVIDIA Ampere. Multi-objective functions help select optimal frequencies that lower power and minimize performance impact showing maximum energy savings of 27% at a performance loss of 1.8%. The same models trained on Ampere showed an accuracy of greater than 93% on an NVIDIA Volta, thereby demonstrating model portability across architectures.
更多查看译文
关键词
GPU,dynamic voltage frequency scaling,Ampere GPU,Volta GPU,energy-efficiency,high-performance computing
AI 理解论文
溯源树
样例
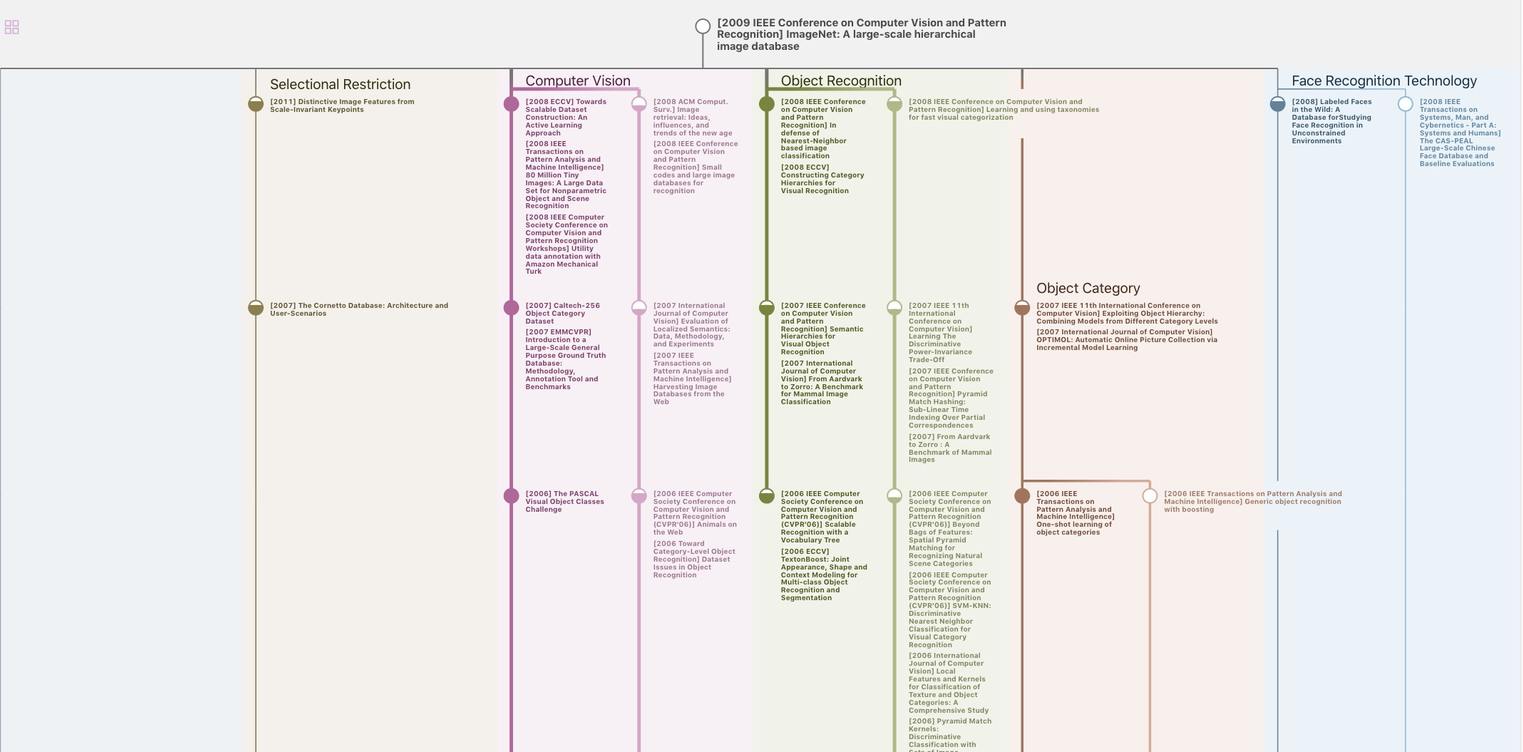
生成溯源树,研究论文发展脉络
Chat Paper
正在生成论文摘要