Embracing Uncertainty for Equity in Resource Allocation in ML Training
PROCEEDINGS OF THE 52ND INTERNATIONAL CONFERENCE ON PARALLEL PROCESSING, ICPP 2023(2023)
摘要
To reduce the Deep Learning (DL) model training time and hence resource consumption, it is critical to avoid stragglers. However, the dynamics and uncertainty features of resource availability pose a challenge to avoiding stragglers caused. To handle this challenge, we propose a Straggler-Avoiding job Scheduling approach (SAS), which smartly ensures that the tasks of a job receive resources with similar dynamics and uncertainty so that the tasks can complete at approximately the same time. Specifically, SAS uses an ML method to predict available resource amounts with probability in future times, groups nodes with similar available resource amounts and probabilities, and then assigns each job to one node group with the objective of minimizing job completion time ( JCT). To reduce the decision making time, we also propose a reinforcement learning (RL) based scheduling approach (SAS-RL) that assigns each job to a node group. In addition, we propose a distributed parameter server (PS) load reassignment method to handle PS stragglers. Our trace-driven real experiments show that SAS reduce up to 45% JCT and 63% stragglers compared with existing job schedulers, and our PS load reassignment reduces up to 48% JCT compared with the previous PS load distribution scheme.
更多查看译文
AI 理解论文
溯源树
样例
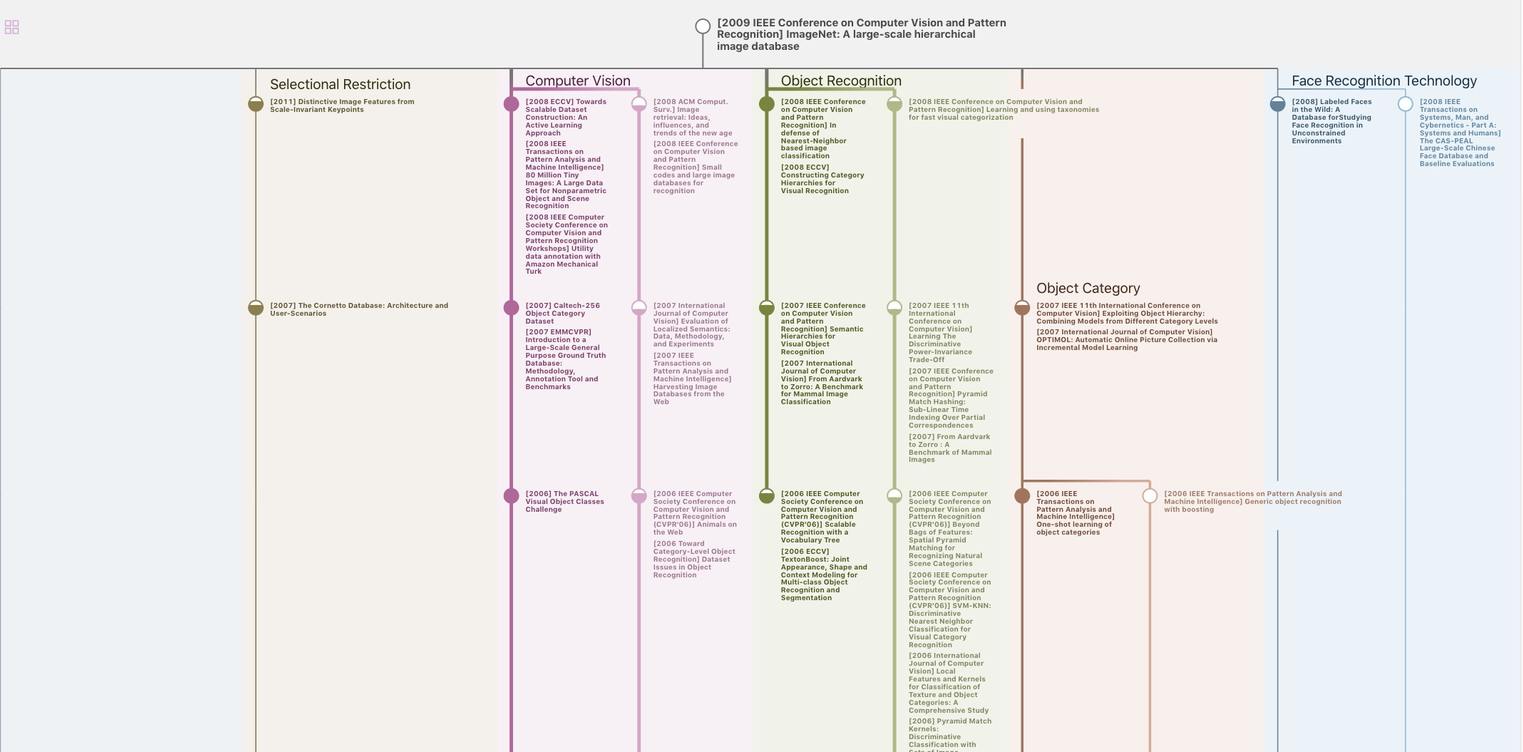
生成溯源树,研究论文发展脉络
Chat Paper
正在生成论文摘要