A Theory of Merge-and-Shrink for Stochastic Shortest Path Problems.
ICAPS(2023)
摘要
The merge-and-shrink framework is a powerful tool to construct state space abstractions based on factored representations. One of its core applications in classical planning is the construction of admissible abstraction heuristics. In this paper, we develop a compositional theory of merge-and-shrink in the context of probabilistic planning, focusing on stochastic shortest path problems (SSPs). As the basis for this development, we contribute a novel factored state space model for SSPs. We show how general transformations, including abstractions, can be formulated on this model to derive admissible and/or perfect heuristics. To formalize the merge-and-shrink framework for SSPs, we transfer the fundamental merge-and-shrink transformations from the classical setting: shrinking, merging, and label reduction. We analyze the formal properties of these transformations in detail and show how the conditions under which shrinking and label reduction lead to perfect heuristics can be extended to the SSP setting.
更多查看译文
关键词
stochastic shortest path problems,merge-and-shrink
AI 理解论文
溯源树
样例
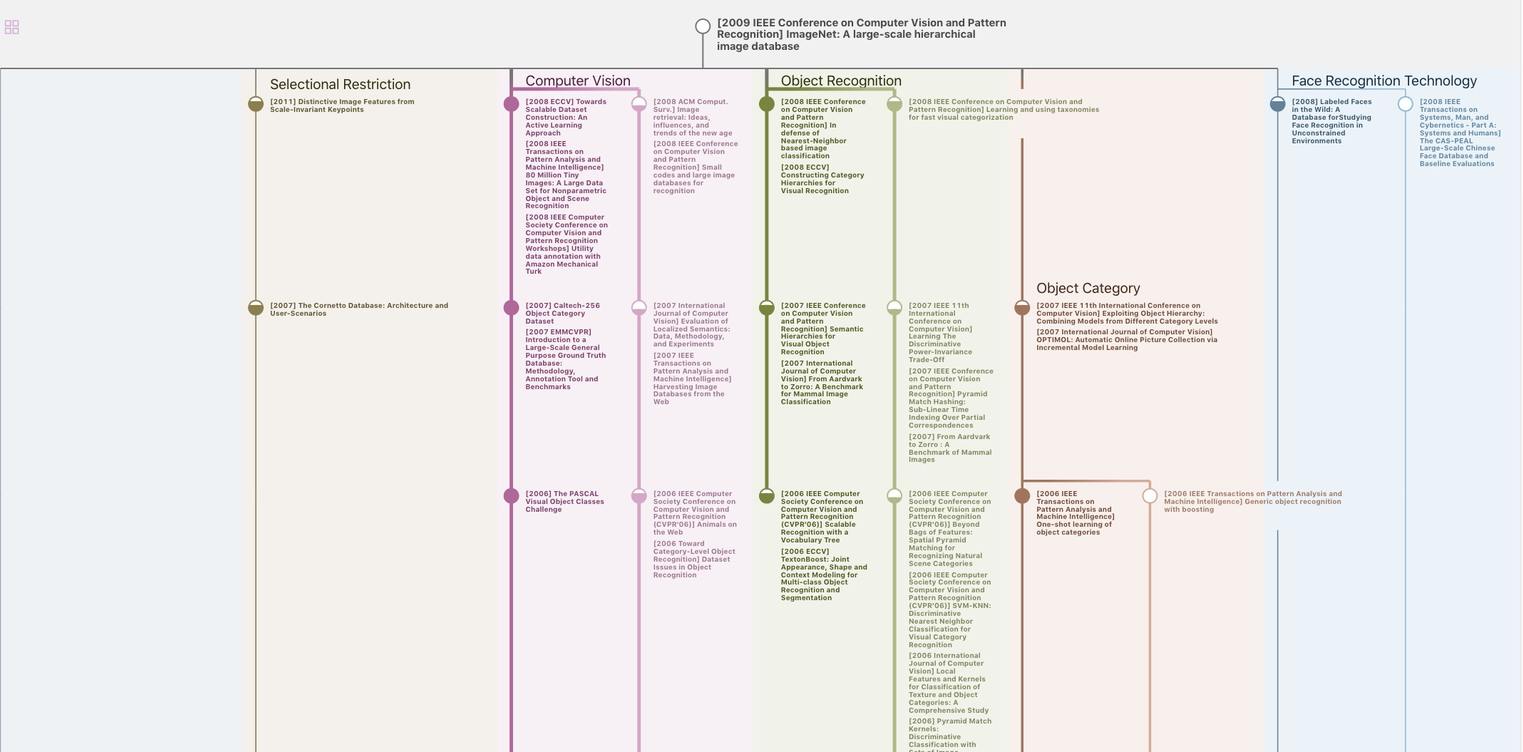
生成溯源树,研究论文发展脉络
Chat Paper
正在生成论文摘要