Collaborative Global-Local Networks for Fine-Grained Few-shot Learning.
ICCAI(2023)
摘要
In this paper, we focus on addressing the fine-grained few-shot learning task, which aims at recognizing the novel classes with a few fine-grained samples for each class, via learning a transfer model with some sample-rich base classes. Compared with the few-shot learning tasks on the coarse-grained classes, the fine-grained few-shot learning task is more challenging due to the intra-class dissimilarity and the inter-class similarity. To this end, we propose a Collaborative Global-Local Network (CGLNet) to exploit both global and local visual features for few-shot fine-grained classification. Through the features processing and multiple distance functions, the proposed CGLNet could effectively exploit the global and local information in features, thus it brings in benefits for the downstream few-shot tasks. To evaluate the effectiveness of the proposed approach, we conduct extensive experiments on three popular fine-grained datasets. The experimental results show that our model achieves superior performance to state-of-the-art methods.
更多查看译文
AI 理解论文
溯源树
样例
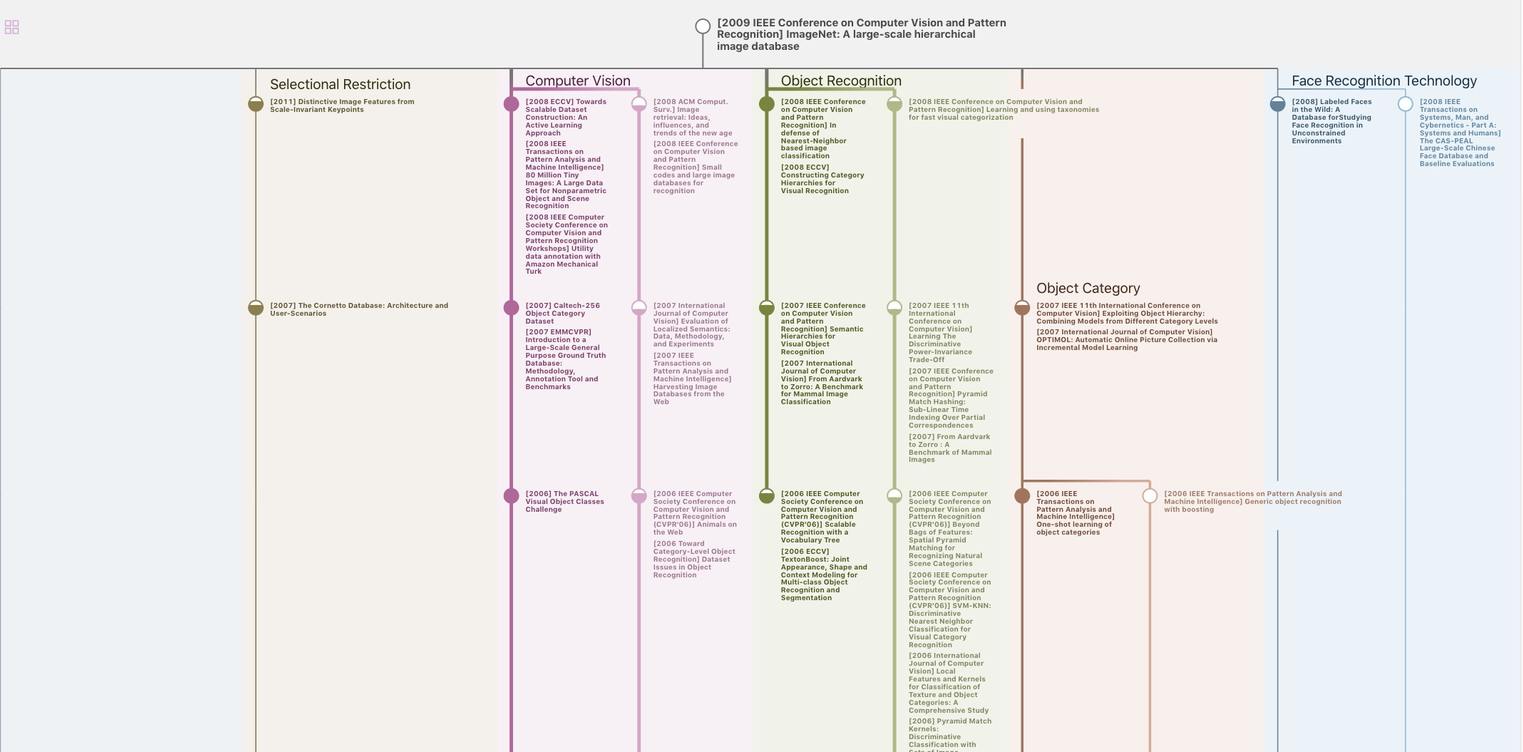
生成溯源树,研究论文发展脉络
Chat Paper
正在生成论文摘要