A Fully Distributed Training for Class Incremental Learning in Multihead Networks.
INFOCOM Workshops(2023)
摘要
Due to good elastic scalability, multi-head network is favored in incremental learning (IL). During IL process, the model size of multi-head network continually grows with the increasing number of branches, which makes it difficult to store and train within a single node. To this end, within model parallelism framework, a distributed training architecture together with its pre-requisite is proposed. Based on the assumption that the pre-requisite is satisfied, a distributed training algorithm is proposed. In addition, to avoid the dilemma that prevalent cross-entropy (CE) loss function does not fit distributed setting, a fully distributed cross-entropy (D-CE) loss function is proposed, which avoids information exchange among nodes. Corresponding training based on D-CE is proposed (D-CE-Train). This method avoids model size expansion problem in centralized training. It employs distributed implementation to speed up training, and reduces the interaction between multiple nodes that may significantly slow down the training. A series of experiments verify the effectiveness of the proposed method.
更多查看译文
关键词
class incremental learning,multi-head network,cross-entropy,distributed implementation
AI 理解论文
溯源树
样例
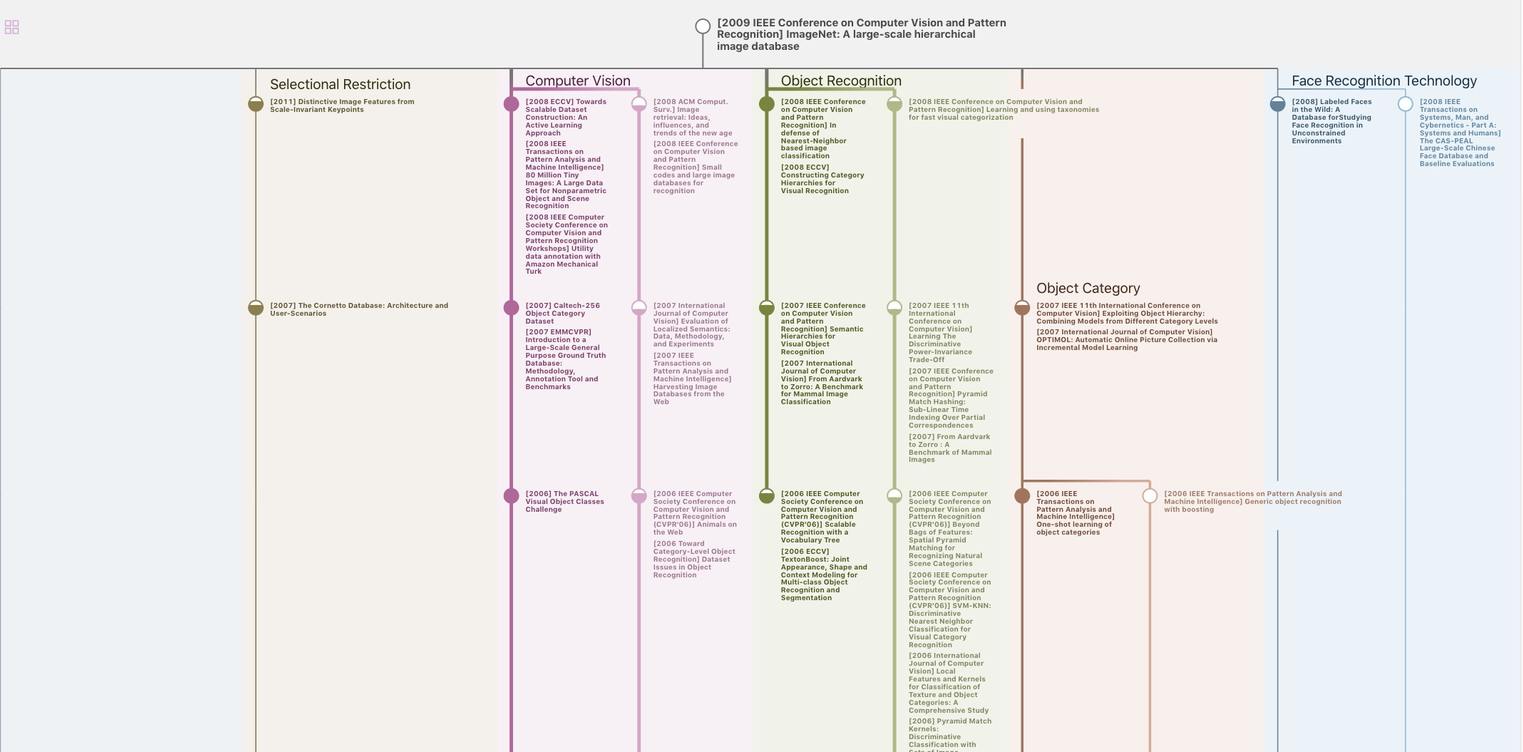
生成溯源树,研究论文发展脉络
Chat Paper
正在生成论文摘要