Homomorphic Filtering Adversarial Defense for Automatic Modulation Classification.
INFOCOM Workshops(2023)
摘要
Deep neural networks provide an intelligent means for automatic modulation classification (AMC) in the communication field. However, due to their interpretability flaws, neural networks are vulnerable to adversarial examples that lead to decision anomalies. In this paper, we propose a homomorphic filtering adversarial defense (HFAD) algorithm for filtering in the signal frequency domain to defend against adversarial examples and promote the safe and reliable application of AMC models. The algorithm attenuates the low frequency components of the signal by performing homomorphic filtering on it, effectively alleviates the error induction of the model output by the adversarial perturbation. Our experimental results demonstrate that the defense algorithm we propose can not only ensure a high recognition accuracy for the original signal, but also effectively resist a variety of white-box adversarial attacks and improve the robustness of the AMC model against adversarial examples.
更多查看译文
关键词
Automatic modulation classification,adversarial defense,homomorphic filtering
AI 理解论文
溯源树
样例
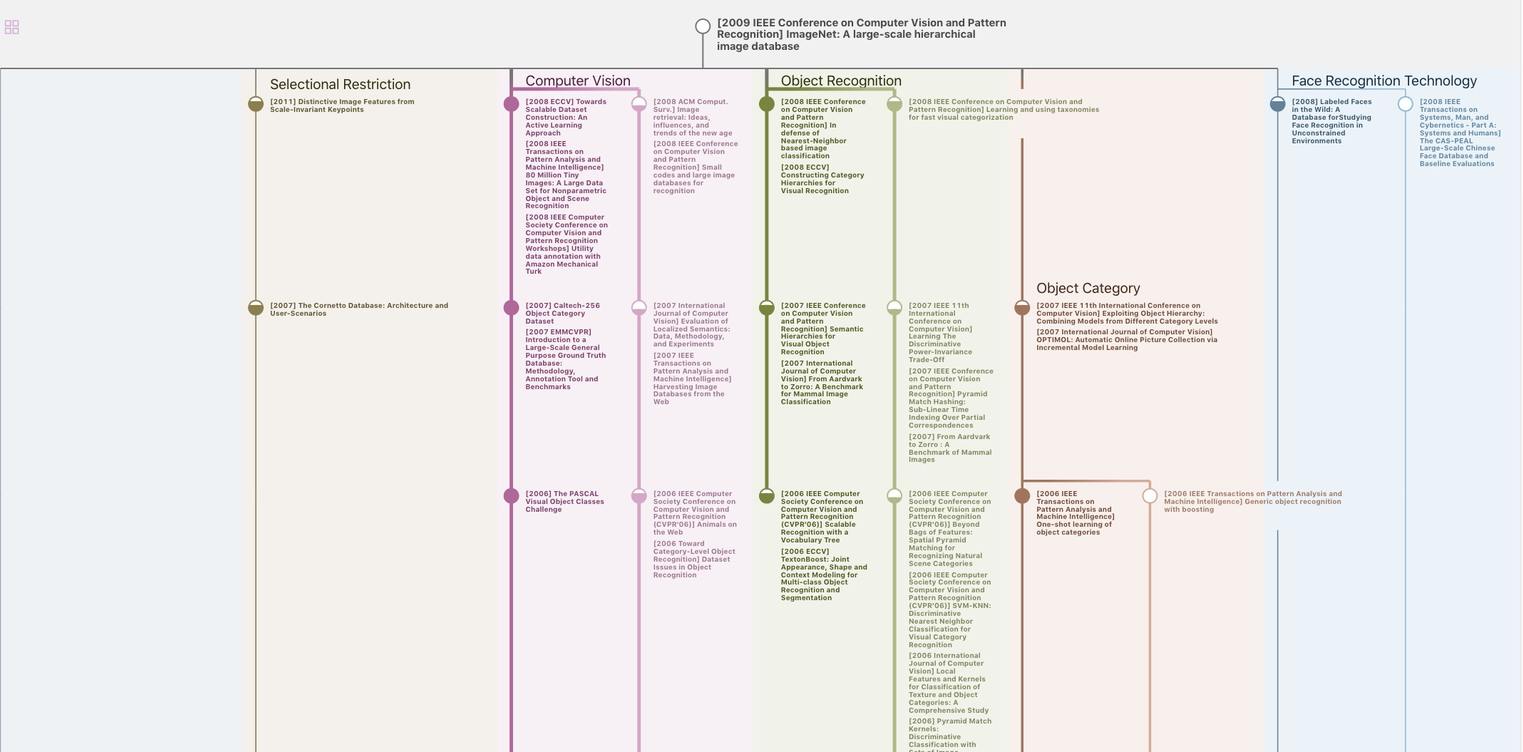
生成溯源树,研究论文发展脉络
Chat Paper
正在生成论文摘要