RDladder: Resolution-Duration Ladder for VBR-encoded Videos via Imitation Learning.
INFOCOM(2023)
摘要
With the rapid development of the streaming system, a large number of videos need to be transcoded to multiple copies according to the encoding ladder, which significantly increases the storage overhead than before. This scenario demonstrates the prosperity of streaming media but also presents new challenges about achieving the balance between better quality for users and less storage cost. In our work, we observe two significant points. The first one is selecting proper resolutions under certain network conditions can reduce storage costs while maintaining a great quality of experience. The second one is the segment duration is critical, especially in VBR-encoded videos. Considering these points, we propose RDladder, a resolution-duration ladder for VBR-encoded videos via imitation learning. We jointly optimize resolutions and duration using neural networks to determine the combination of these two metrics considering network capacity, video information, and storage cost. To get more faithful results, we use over 500 videos, encoded to over 2,000,000 chunks, and collect real-world network traces for more than 50 hours. We test RDladder in simulation, emulation, and real-world environments under various network conditions, and our method can achieve near-optimal performance. Furthermore, we discuss the influence between the RDladder and ABR algorithms and summarize some characteristics of RDladder.
更多查看译文
关键词
ABR algorithms,encoding ladder,imitation learning,less storage cost,multiple copies,near-optimal performance,network conditions,neural networks,proper resolutions,quality of experience,RDladder,real-world environments,real-world network traces,resolution-duration ladder,segment duration,storage cost,storage overhead,streaming media,streaming system,VBR-encoded videos,video information
AI 理解论文
溯源树
样例
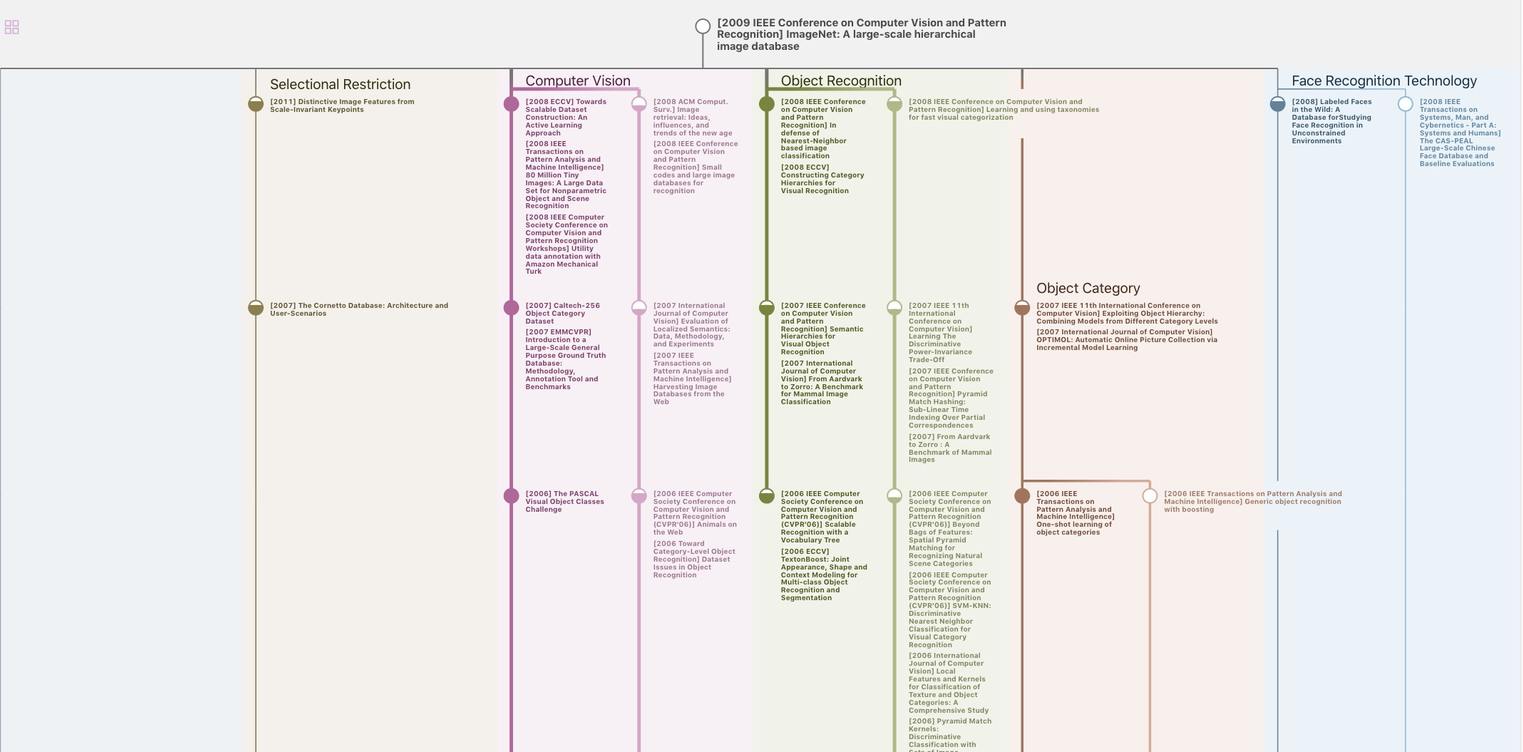
生成溯源树,研究论文发展脉络
Chat Paper
正在生成论文摘要