Spatiotemporal Transformer for Data Inference and Long Prediction in Sparse Mobile CrowdSensing.
INFOCOM(2023)
摘要
Mobile CrowdSensing (MCS) is a data sensing paradigm that recruits users carrying mobile terminals to collect data. As its variant, Sparse MCS has been further proposed for large-scale and fine-grained sensing task with the advantage of collecting only a few data to infer unsensed data. However, in many real-world scenarios, such as early prevention of epidemic, people are interested in not only the data at the current, but also in the future or even long-term future, and the latter may be more important. Long-term prediction not only reduces sensing cost, but also identifies trends or other characteristics of the data. In this paper, we propose a spatiotemporal model based on Transformer to infer and predict the data with sparse sensed data by utilizing spatiotemporal relationships. We design a spatiotemporal feature embedding to embed the prior spatiotemporal information of sensing map into the model to guide model learning. Moreover, we also design a novel multi-head spatiotemporal attention mechanism to dynamically capture spatiotemporal relationships among data. Extensive experiments have been conducted on three types of typical urban sensing tasks, which verify the effectiveness of our proposed algorithms in improving the inference and long-term prediction accuracy with the sparse sensed data.
更多查看译文
关键词
Mobile crowdsensing,transformer,spatiotemporal relationships,attention mechanism
AI 理解论文
溯源树
样例
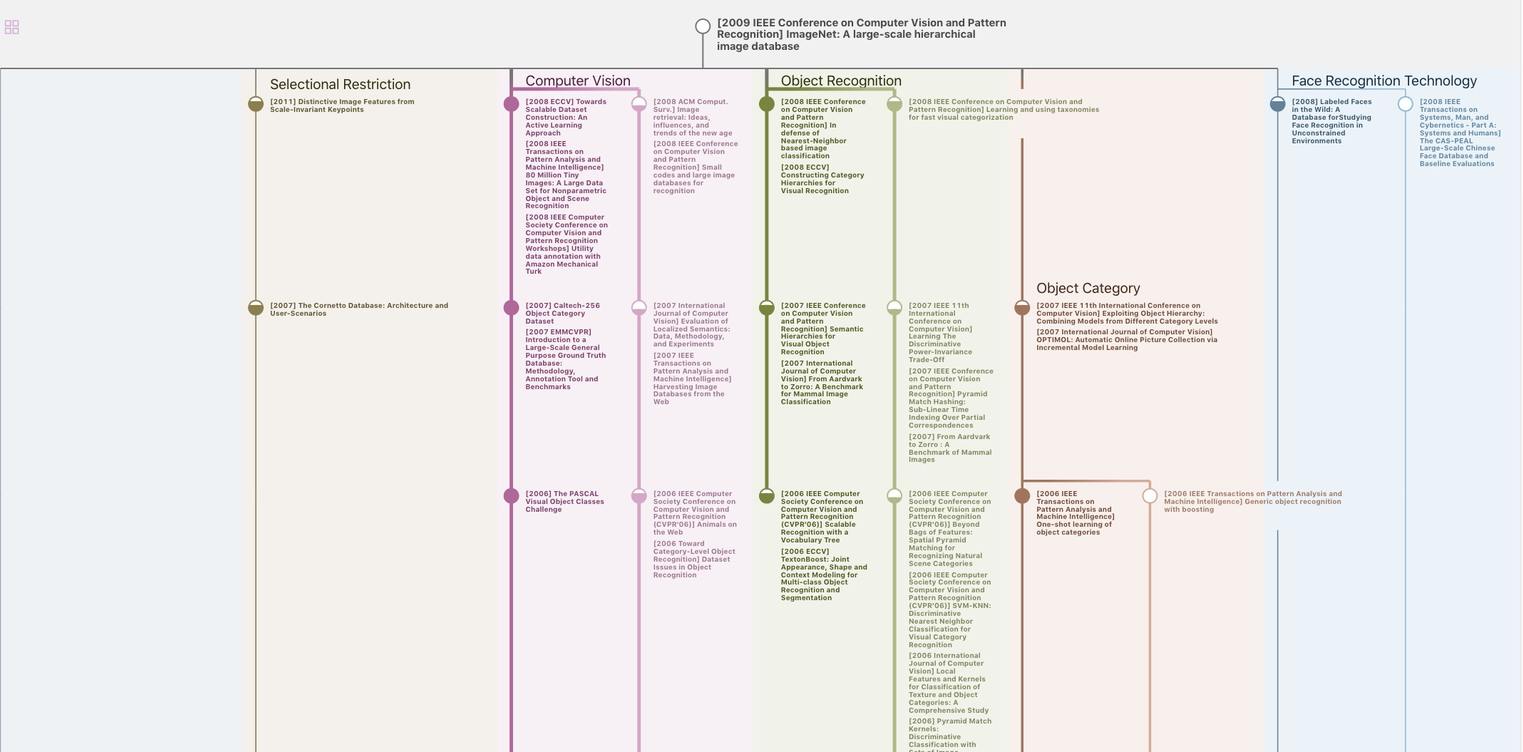
生成溯源树,研究论文发展脉络
Chat Paper
正在生成论文摘要