Poster: Digital Network Twin via Learning-Based Simulator.
INFOCOM Workshops(2023)
摘要
Digital network twin (DNT) allows network operators to test their network management policy before their actual deployment in real-world networks. Achieving DNT, however, can be challenging and compute-intensive if every detail needs to be replicated exactly. In this work, we propose a new compute-efficient approach to realize DNT by augmenting existing network simulators. First, we build a real-world testbed by using OpenAirInterface and replicate its settings with the NS-3 simulator. Second, we observe the non-trivial distributional discrepancy between the simulator and the real-world testbed. Third, we use deep learning techniques to bridge the sim-to-real discrepancy under different network states. The experimental results show our method can reduce up to 91% sim-to-real discrepancy.
更多查看译文
关键词
Digital Network Twin,Simulation-to-Reality Discrepancy,Machine Learning
AI 理解论文
溯源树
样例
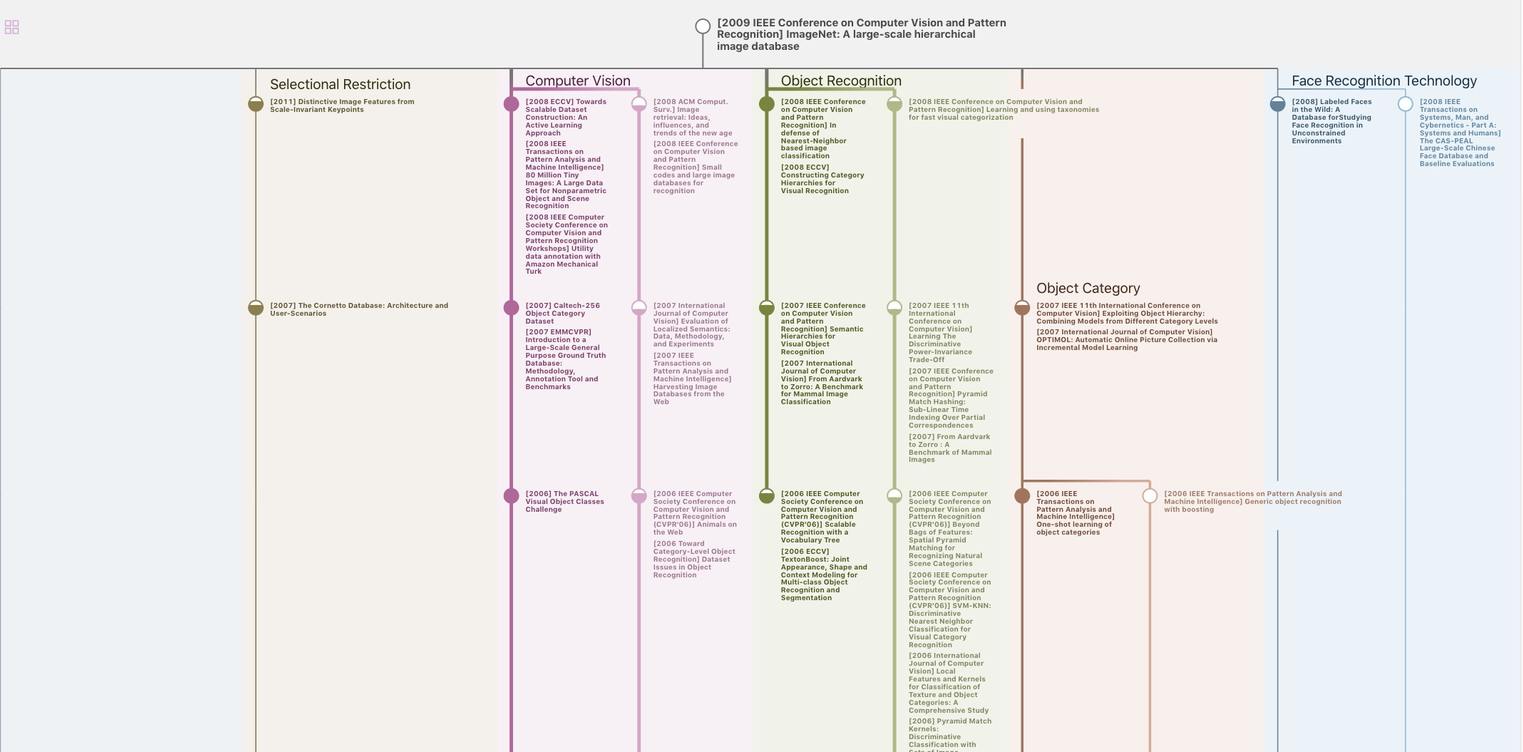
生成溯源树,研究论文发展脉络
Chat Paper
正在生成论文摘要