A Multi-label Imbalanced Data Classification Method Based on Label Partition Integration.
WISA(2023)
摘要
The problem of multi-label classification is widespread in real life, and its imbalanced characteristics seriously affect classification performance. Currently, resampling methods can be used to solve the problem of imbalanced classification of multi-label data. However, resampling methods ignore the correlation between labels, which may pull in new imbalance while changing the distribution of the original dataset, resulting in a decrease in classification performance instead of an increase. In addition, the resampling ratio needs to be manually set, resulting in significant fluctuations in classification performance. To address this issue, a multi-label imbalanced data classification method ESP based on label partition integration is proposed. ESP divides the dataset into single label datasets and label pair datasets without changing its original distribution, and then learns each dataset to construct multiple binary classification models. Finally, all binary classification models are integrated into a multi-label classification model. The experimental results show that ESP outperforms the five commonly used resampling methods in two common measures: F-Measure and Accuracy.
更多查看译文
关键词
classification,multi-label
AI 理解论文
溯源树
样例
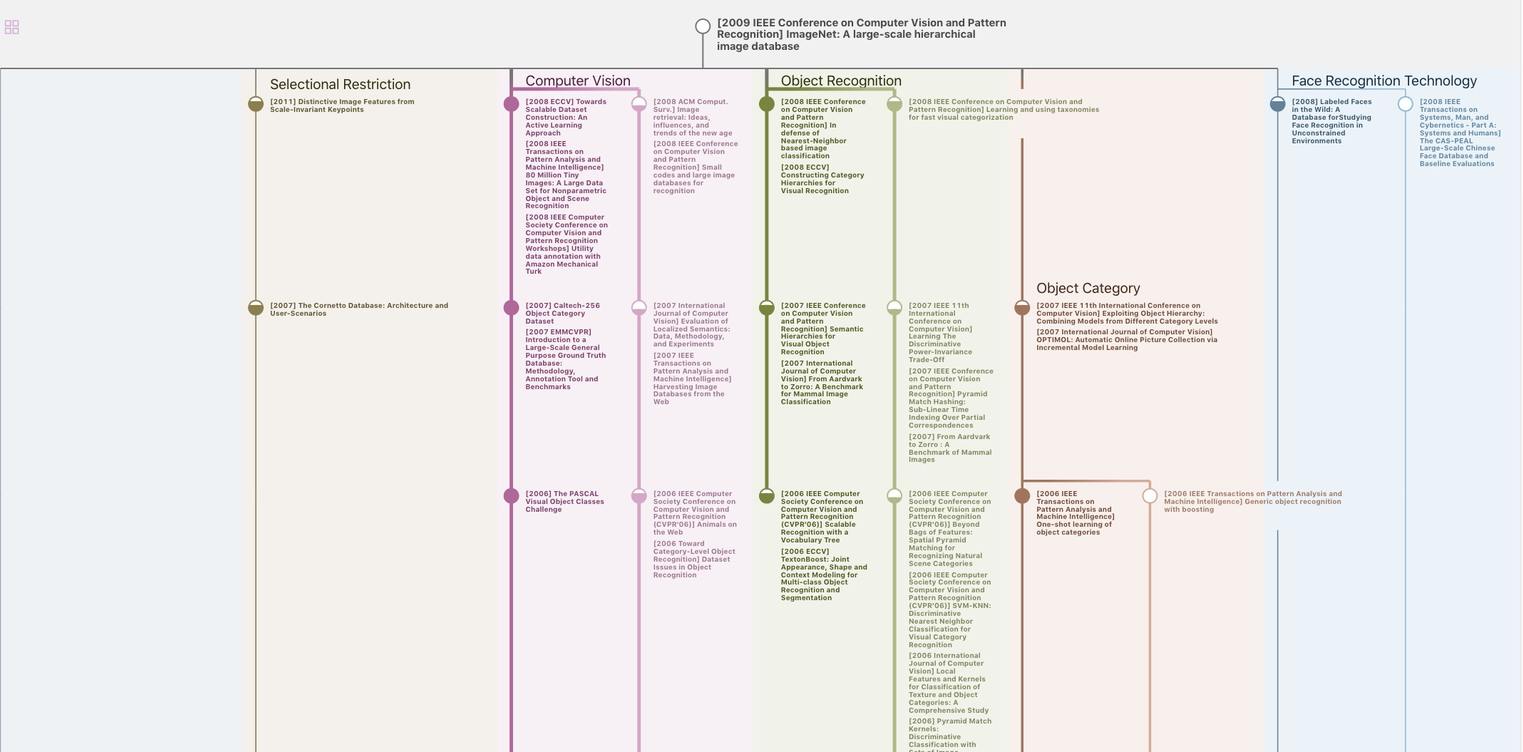
生成溯源树,研究论文发展脉络
Chat Paper
正在生成论文摘要