Federated Learning at the Edge: An Interplay of Mini-batch Size and Aggregation Frequency.
INFOCOM Workshops(2023)
摘要
Federated Learning (FL) is a distributed learning paradigm that can coordinate heterogeneous edge devices to perform model training without sharing private raw data. Prior works on the convergence analysis of FL have focused on mini-batch size and aggregation frequency separately. However, increasing the batch size and the number of local updates can differently affect model performance and system overhead. This paper proposes a novel model in quantifying the interplay of FL mini-batch size and aggregation frequency to navigate the unique trade-offs among convergence, completion time, and resource cost. We obtain a new convergence bound for synchronous FL with respect to these decision variables under heterogeneous training datasets at different devices. Based on this bound, we derive closed-form solutions for co-optimized mini-batch size and aggregation frequency, uniformly among devices. We then design an efficient exact algorithm to optimize heterogeneous mini-batch configurations, further improving the model accuracy. An adaptive control algorithm is also proposed to dynamically adjust the batch sizes and the number of local updates per round. Extensive experiments demonstrate the superiority of our offline optimized solutions and online adaptive algorithm.
更多查看译文
关键词
aggregation frequency,convergence analysis,cooptimized mini-batch size,distributed learning paradigm,federated learning,FL mini-batch size,heterogeneous edge devices,heterogeneous mini-batch configurations,heterogeneous training datasets,local updates,model accuracy,model training,private raw data,synchronous FL,system overhead
AI 理解论文
溯源树
样例
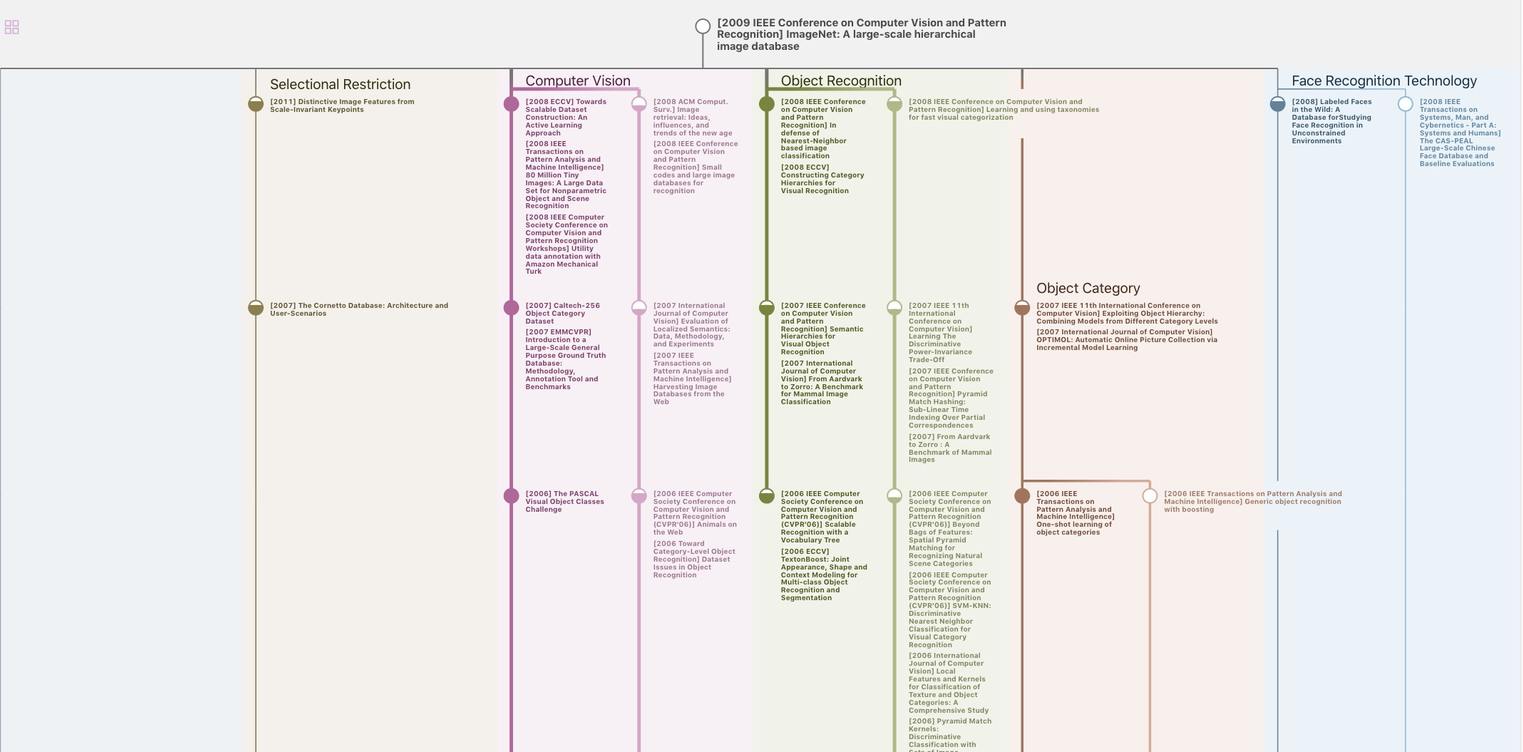
生成溯源树,研究论文发展脉络
Chat Paper
正在生成论文摘要