QueuePilot: Reviving Small Buffers With a Learned AQM Policy.
INFOCOM(2023)
摘要
There has been much research effort on using small buffers in backbone routers, to provide lower delays for users and free up capacity for vendors. Unfortunately, with small buffers, the droptail policy has an excessive loss rate, and existing AQM (active queue management) policies can be unreliable.We introduce QueuePilot, an RL (reinforcement learning)-based AQM that enables small buffers in backbone routers, trading off high utilization with low loss rate and short delay. QueuePilot automatically tunes the ECN (early congestion notification) marking probability. After training once offline with a variety of settings, QueuePilot produces a single lightweight policy that can be applied online without further learning. We evaluate QueuePilot on real networks with hundreds of TCP connections, and show how its performance in small buffers exceeds that of existing algorithms, and even exceeds their performance with larger buffers.
更多查看译文
关键词
active queue management,backbone routers,droptail policy,ECN marking probability,excessive loss rate,existing AQM policies,larger buffers,learned AQM policy,low loss rate,lower delays,QueuePilot,reinforcement learning,RL-based AQM,short delay,single lightweight policy
AI 理解论文
溯源树
样例
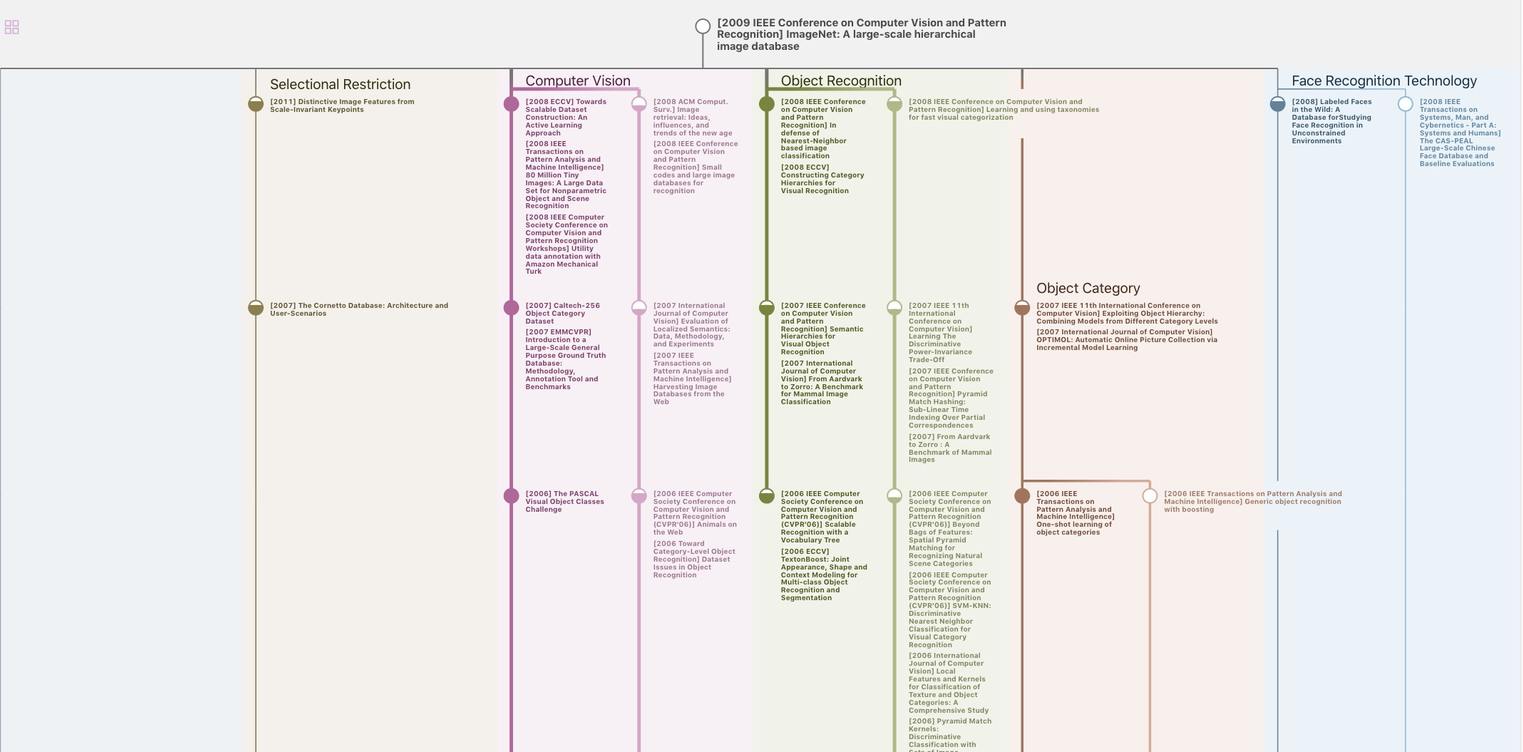
生成溯源树,研究论文发展脉络
Chat Paper
正在生成论文摘要