Bootstrapped Personalized Popularity for Cold Start Recommender Systems
PROCEEDINGS OF THE 17TH ACM CONFERENCE ON RECOMMENDER SYSTEMS, RECSYS 2023(2023)
摘要
Recommender Systems are severely hampered by the well-known Cold Start problem, identified by the lack of information on new items and users. This has led to research efforts focused on data imputation and augmentation models as predominantly data preprocessing strategies, yet their improvement of cold-user performance is largely indirect and often comes at the price of a reduction in accuracy for warmer users. To address these limitations, we propose Bootstrapped Personalized Popularity (B2P), a novel framework that improves performance for cold users (directly) and cold items (implicitly) via popularity models personalized with item metadata. B2P is scalable to very large datasets and directly addresses the Cold Start problem, so it can complement existing Cold Start strategies. Experiments on a real-world dataset from the BBC iPlayer and a public dataset demonstrate that B2P (1) significantly improves cold-user performance, (2) boosts warm-user performance for bootstrapped models by lowering their training sparsity, and (3) improves total recommendation accuracy at a competitive diversity level relative to existing high-performing Collaborative Filtering models. We demonstrate that B2P is a powerful and scalable framework for strongly cold datasets.
更多查看译文
关键词
recommender systems,collaborative filtering,cold-start problem,popularity modelling,high-order personalization
AI 理解论文
溯源树
样例
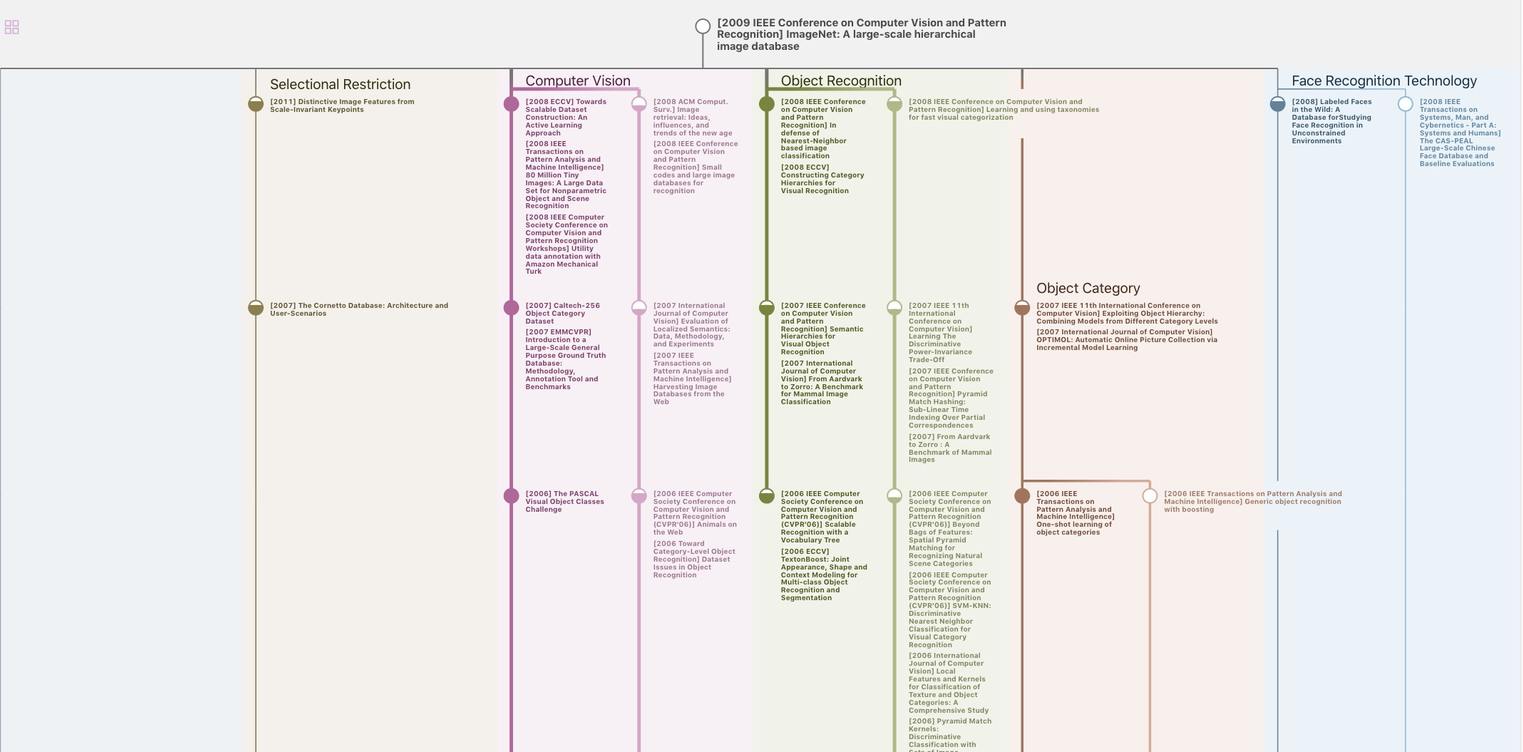
生成溯源树,研究论文发展脉络
Chat Paper
正在生成论文摘要