Integrating the ACT-R Framework with Collaborative Filtering for Explainable Sequential Music Recommendation
PROCEEDINGS OF THE 17TH ACM CONFERENCE ON RECOMMENDER SYSTEMS, RECSYS 2023(2023)
摘要
Music listening sessions often consist of sequences including repeating tracks. Modeling such relistening behavior with models of human memory has been proven effective in predicting the next track of a session. However, these models intrinsically lack the capability of recommending novel tracks that the target user has not listened to in the past. Collaborative filtering strategies, on the contrary, provide novel recommendations by leveraging past collective behaviors but are often limited in their ability to provide explanations. To narrow this gap, we propose four hybrid algorithms that integrate collaborative filtering with the cognitive architecture ACT-R. We compare their performance in terms of accuracy, novelty, diversity, and popularity bias, to baselines of different types, including pure ACT-R, kNN-based, and neural-networks-based approaches. We show that the proposed algorithms are able to achieve the best performances in terms of novelty and diversity, and simultaneously achieve a higher accuracy of recommendation with respect to pure ACT-R models. Furthermore, we illustrate how the proposed models can provide explainable recommendations.
更多查看译文
关键词
Adaptive Control Thought-Rational (ACT-R),Collaborative Filtering,Sequential Recommendation,Music Recommender Systems,Psychology-Informed Recommender Systems,Explainability
AI 理解论文
溯源树
样例
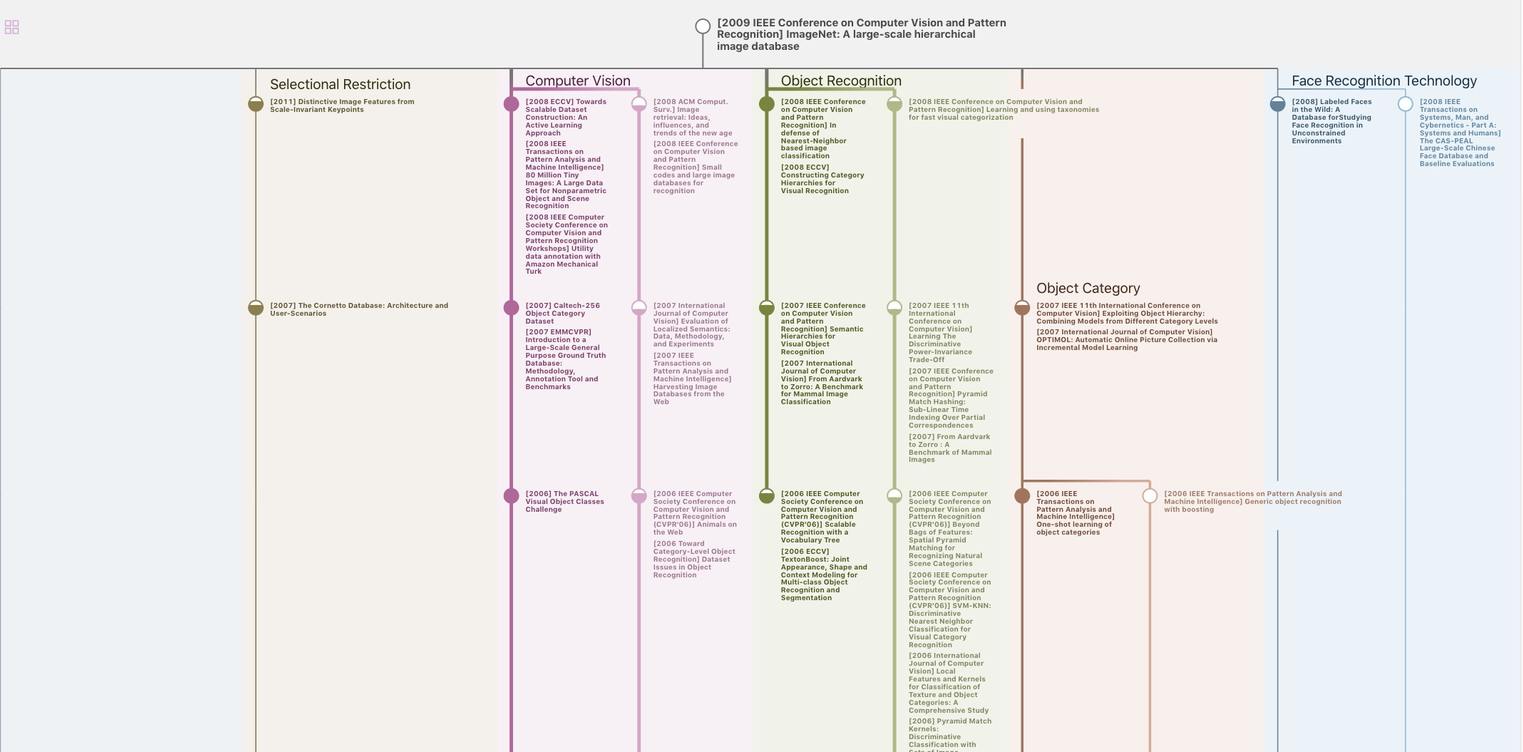
生成溯源树,研究论文发展脉络
Chat Paper
正在生成论文摘要