Group Fairness for Content Creators: the Role of Human and Algorithmic Biases under Popularity-based Recommendations
PROCEEDINGS OF THE 17TH ACM CONFERENCE ON RECOMMENDER SYSTEMS, RECSYS 2023(2023)
摘要
The Creator Economy faces concerning levels of unfairness. Content creators (CCs) publicly accuse platforms of purposefully reducing the visibility of their content based on protected attributes, while platforms place the blame on viewer biases. Meanwhile, prior work warns about the "rich-get-richer" effect perpetuated by existing popularity biases in recommender systems: Any initial advantage in visibility will likely be exacerbated over time. What remains unclear is how the biases based on protected attributes from platforms and viewers interact and contribute to the observed inequality in the context of popularity-biased recommender systems. The difficulty of the question lies in the complexity and opacity of the system. To overcome this challenge, we design a simple agent-based model (ABM) that unifies the platform systems which allocate the visibility of CCs (e.g., recommender systems, moderation) into a single popularity-based function, which we call the visibility allocation system (VAS). Through simulations, we find that although viewer homophilic biases do alone create inequalities, small levels of additional biases in VAS are more harmful. From the perspective of interventions, our results suggest that (a) attempts to reduce attribute-biases in moderation and recommendations should precede those reducing viewers' homophilic tendencies, (b) decreasing the popularity-biases in VAS decreases but not eliminates inequalities, (c) boosting the visibility of protected CCs to overcome viewers' homophily with respect to one fairness metric is unlikely to produce fair outcomes with respect to all metrics, and (d) the process is also unfair for viewers and this unfairness could be overcome through the same interventions. More generally, this work demonstrates the potential of using ABMs to better understand the causes and effects of biases and interventions within complex sociotechnical systems.
更多查看译文
关键词
algorithmic fairness,agent-based modeling,network formation,popularity bias
AI 理解论文
溯源树
样例
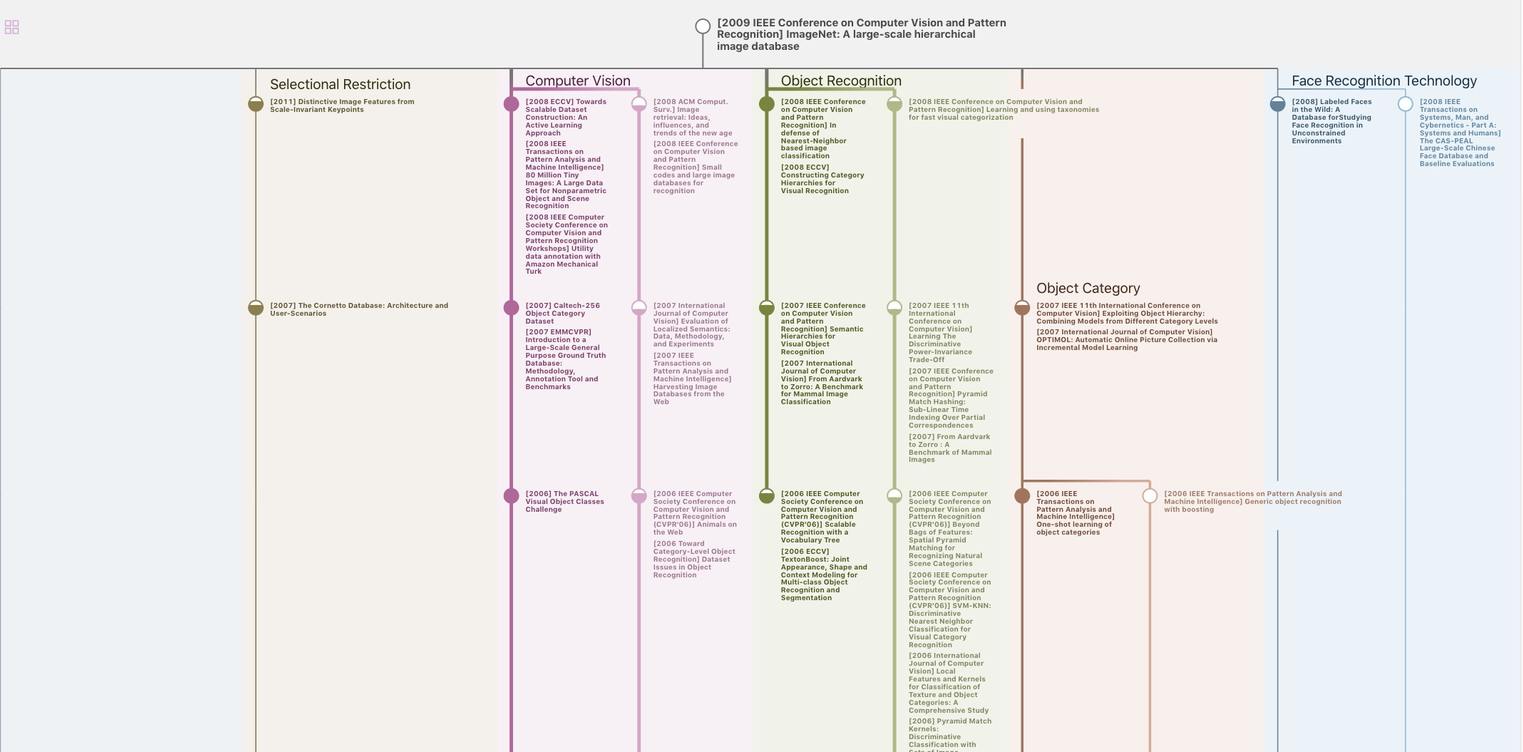
生成溯源树,研究论文发展脉络
Chat Paper
正在生成论文摘要