Scalable Deep Q-Learning for Session-Based Slate Recommendation
PROCEEDINGS OF THE 17TH ACM CONFERENCE ON RECOMMENDER SYSTEMS, RECSYS 2023(2023)
摘要
Reinforcement learning (RL) has demonstrated great potential to improve slate-based recommender systems by optimizing recommendations for long-term user engagement. To handle the combinatorial action space in slate recommendation, recent works decompose the Q-value of a slate into item-wise Q-values, using an item-wise value-based policy. However, the common case where the value function is a parameterized function taking state and action as input results in a linearly increasing number of evaluations required to select an action, proportional to the number of candidate items. While slow training may be acceptable, this becomes intractable when considering the costly evaluation of the parameterized function, such as with deep neural networks, during model serving time. To address this issue, we propose an actor-based policy that reduces the evaluation of the Q-function to a subset of items, significantly reducing inference time and enabling practical deployment in real-world industrial settings. In our empirical evaluation, we demonstrate that our proposed approach achieves equivalent user session engagement to a value-based policy, while significantly reducing the slate serving time by at least 4 times.
更多查看译文
关键词
Recommender systems,Slate recommendation,Reinforcement learning
AI 理解论文
溯源树
样例
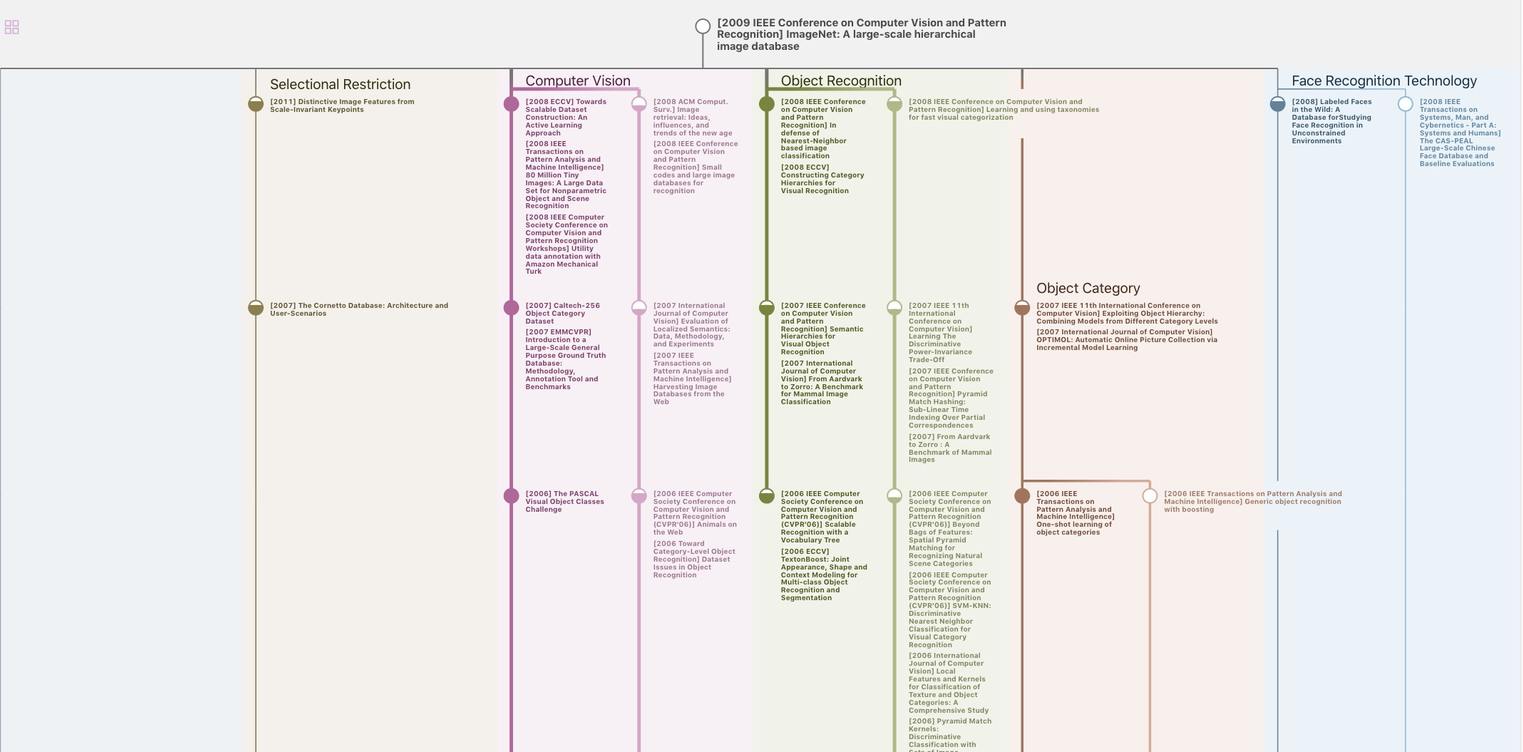
生成溯源树,研究论文发展脉络
Chat Paper
正在生成论文摘要