On the Consistency, Discriminative Power and Robustness of Sampled Metrics in Offline Top-N Recommender System Evaluation
PROCEEDINGS OF THE 17TH ACM CONFERENCE ON RECOMMENDER SYSTEMS, RECSYS 2023(2023)
摘要
Negative item sampling in offline top-n recommendation evaluation has become increasingly wide-spread, but remains controversial. While several studies have warned against using sampled evaluation metrics on the basis of being a poor approximation of the full ranking (i.e. using all negative items), others have highlighted their improved discriminative power and potential to make evaluation more robust. Unfortunately, empirical studies on negative item sampling are based on relatively few methods (between 3-12) and, therefore, lack the statistical power to assess the impact of negative item sampling in practice. In this article, we present preliminary findings from a comprehensive benchmarking study of negative item sampling based on 52 recommendation algorithms and 3 benchmark data sets. We show how the number of sampled negative items and different sampling strategies affect the consistency and discriminative power of sampled evaluation metrics. Furthermore, we investigate the impact of sparsity bias and popularity bias on the robustness of these metrics. In brief, we show that the optimal parameterizations for negative item sampling are dependent on data set characteristics and the goals of the investigator, suggesting a need for greater transparency in related experimental design decisions.
更多查看译文
关键词
recommender systems,offline evaluation
AI 理解论文
溯源树
样例
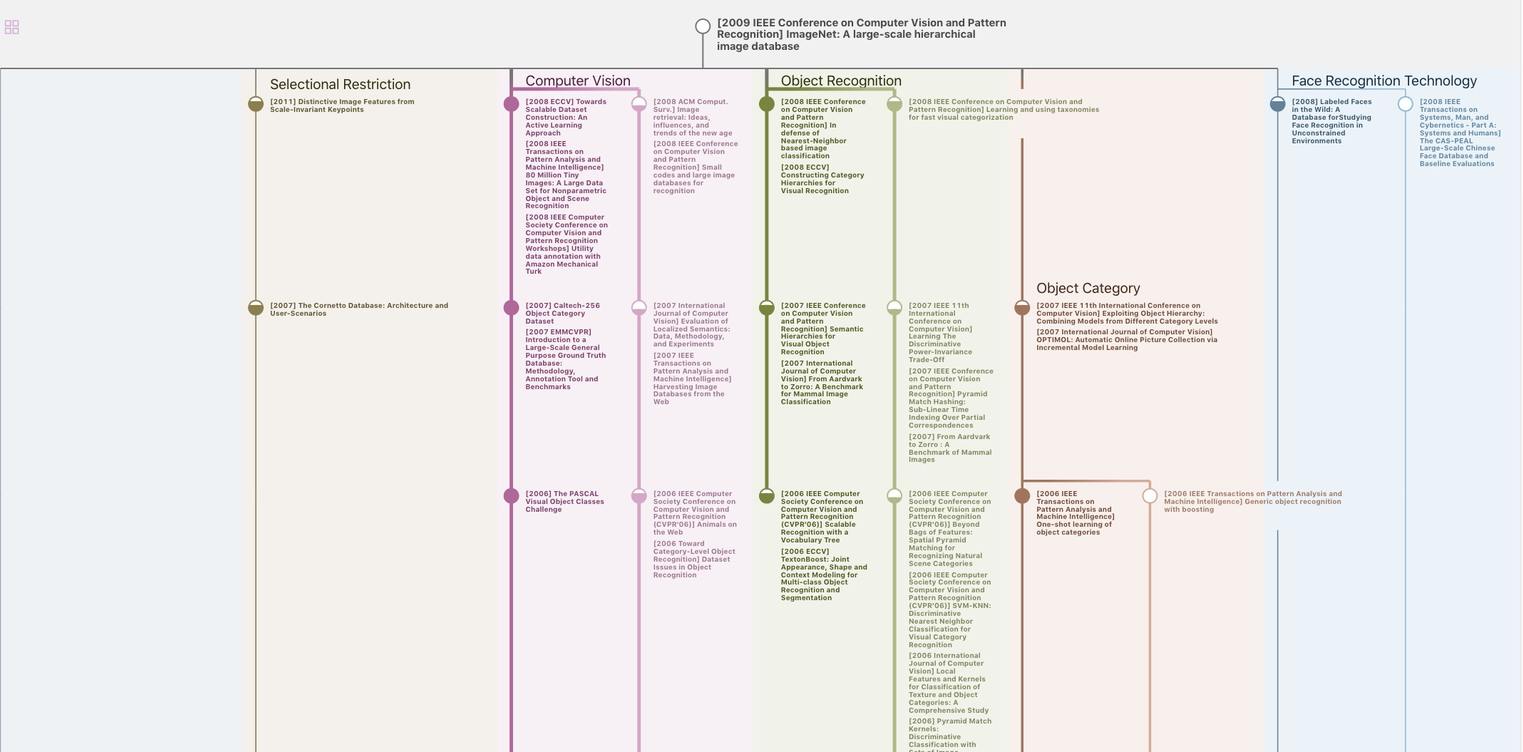
生成溯源树,研究论文发展脉络
Chat Paper
正在生成论文摘要