COBALT: A Content-Based Similarity Approach for Link Discovery over Geospatial Knowledge Graphs
KNOWLEDGE GRAPHS: SEMANTICS, MACHINE LEARNING, AND LANGUAGES(2023)
摘要
Purpose: Data integration and applications across knowledge graphs (KGs) rely heavily on the discovery of links between resources within these KGs. Geospatial link discovery algorithms have to deal with millions of point sets containing billions of points. Methodology: To speed up the discovery of geospatial links, we propose COBALT. COBALT combines the content measures with R-tree indexing. The content measures are based on the area, diagonal and distance of the minimum bounding boxes of the polygons which speeds up the process but is not perfectly accurate. We thus propose two polygon splitting approaches for improving the accuracy of COBALT. Findings: Our experiments on real-world datasets show that COBALT is able to speed up the topological relation discovery over geospatial KGs by up to 1.47x104 times over state-of-the-art linking algorithms while maintaining an F-Measure between 0.7 and 0.9 depending on the relation. Furthermore, we were able to achieve an F-Measure of up to 0.99 by applying our polygon splitting approaches before applying the content measures. Value: The process of discovering links between geospatial resources can be significantly faster by sacrificing the optimality of the results. This is especially important for realtime data-driven applications such as emergency response, location-based services and traffic management. In future work, additional measures, like the location of polygons or the name of the entity represented by the polygon, could be integrated to further improve the accuracy of the results.
更多查看译文
关键词
Knowledge graphs,Data Integration,Linked Data,Geospatial Knowledge graphs,Content Measure Similarity,Topological Relations
AI 理解论文
溯源树
样例
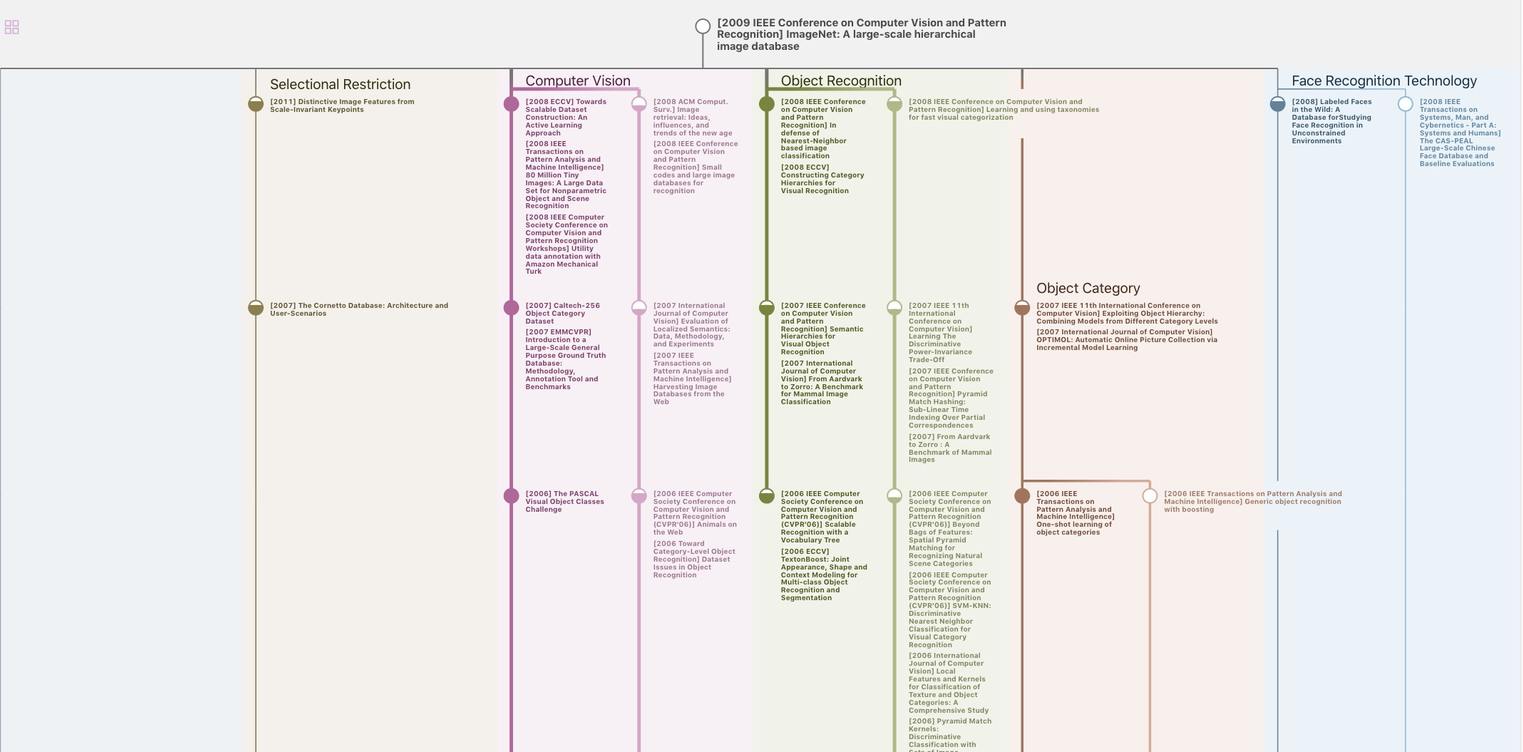
生成溯源树,研究论文发展脉络
Chat Paper
正在生成论文摘要