Differentially Private Copulas, DAG and Hybrid Methods: A Comprehensive Data Utility Study
COMPUTATIONAL COLLECTIVE INTELLIGENCE, ICCCI 2023(2023)
摘要
Differentially Private (DP) synthetic data generation (SDG) algorithms take as input a dataset containing private, confidential information and produce synthetic data with comparable statistical characteristics. The significance of such techniques is rising due to the growing awareness of the extent of data collection and usage in organizational contexts, as well as the implementation of new stricter data privacy regulations. Given the growing academic interest in DP SDG techniques, our study intends to perform a comparative evaluation of the statistical similarities and utility (in terms of machine learning performances) of a specific set of related algorithms in the realistic context of credit-risk and banking. The study compares PrivBayes, Copula-Shirley, and DPCopula algorithms and their variants using a proposed evaluation framework across three different datasets. The purpose of this study is to perform a thorough assessment of the score and to investigate the impact of different values of the privacy budget (epsilon) on the quality and usability of synthetic data generated by each method. As a result, we highlight and examine the deficiencies and capabilities of each algorithm in relation to the features' properties of the original data.
更多查看译文
关键词
Synthetic Data Generation,Differential Privacy,Evaluation Metrics,Copula Functions,Bayesian Networks
AI 理解论文
溯源树
样例
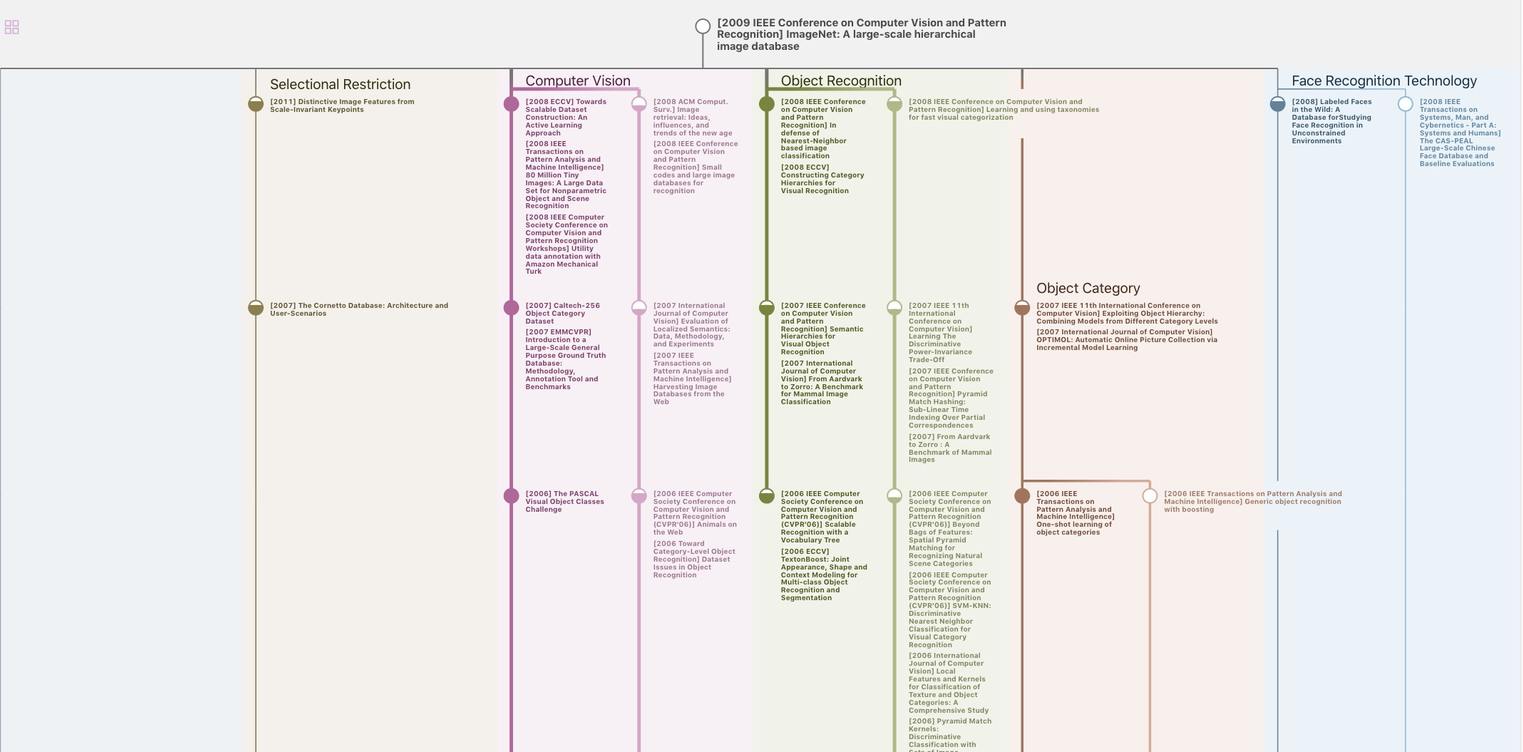
生成溯源树,研究论文发展脉络
Chat Paper
正在生成论文摘要