SS-FL: Self-Supervised Federated Learning for COVID-19 Detection from Chest X-Ray Images.
ICCCI (CCIS Volume)(2023)
摘要
Recently Federated Learning has gained considerable popularity and becomes an attractive learning paradigm due to the privacy and the computational advantages that it offers. However, it is facing a major challenge: the lack of labeled data on certain federated settings. This is known as label deficiency challenge and it remains less studied. To address this challenge and related problems, we propose in this work a simple Self-Supervised Federated Learning based framework for COVID-19 detection from chest X-ray images, named (SS-FL). Due to the scarcity of radiological images of COVID-19 and the confidentiality considerations, the aim of this framework is to benefit from the large amount of available unlabeled chest X-ray images to learn useful visual representations that will help in distinguishing COVID-19 from other pathologies. We propose to train a deep neural network in a self-supervised way on large unlabeled dataset at server-side and then to fine-tune it, in a federated fashion, on a small amount of labeled data at clients-sides. Our simulation results show that the use of the self-supervised pre-training step at server level allows to improve the accuracy compared with the classic supervised FL: an improvement of 3.1% on COVID-19 dataset. We also highlight the ability of pre-training a global model with unlabeled data to speed up the overall federated training process.
更多查看译文
关键词
federated learning,detection,self-supervised,x-ray
AI 理解论文
溯源树
样例
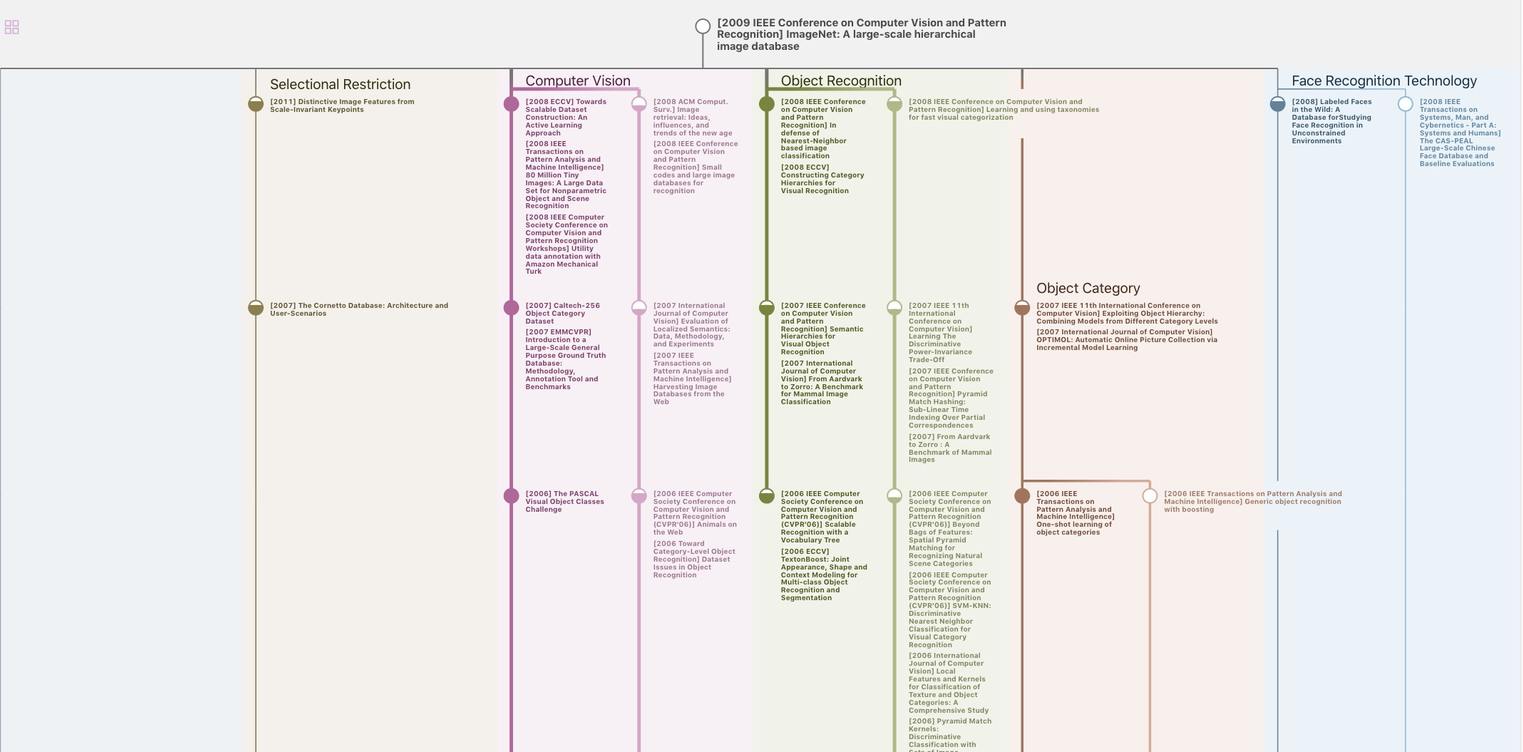
生成溯源树,研究论文发展脉络
Chat Paper
正在生成论文摘要