Sparsity-Invariant Convolution for Forecasting Irregularly Sampled Time Series
COMPUTATIONAL COLLECTIVE INTELLIGENCE, ICCCI 2023(2023)
摘要
Time series forecasting techniques range from ARIMA over exponential smoothing to neural approaches, such as convolutional neural networks. However, most of them were designed to work with regularly sampled and complete time series, i.e., time series which can be represented as a sequence of numbers without missing values. In contrast, we consider the task of forecasting irregularly sampled time series in this paper. We argue that, compared with "usual" convolution, sparsity-invariant convolution is better suited for the case of irregularly sampled time series, therefore, we propose to use neural networks with sparsity-invariant convolution. We perform experiments on 30 publicly-available real-world time series datasets and show that sparsity-invariant convolution significantly improves the performance of convolutional neural networks in case of forecasting irregularly sampled time series. In order to support reproduction, independent validation and follow-up works, we made our implementation (software code) publicly available at https://github.com/kr7/timeseriesforecast-siconv.
更多查看译文
关键词
time series forecasting,convolutional neural network,sparsity-invariant convolution
AI 理解论文
溯源树
样例
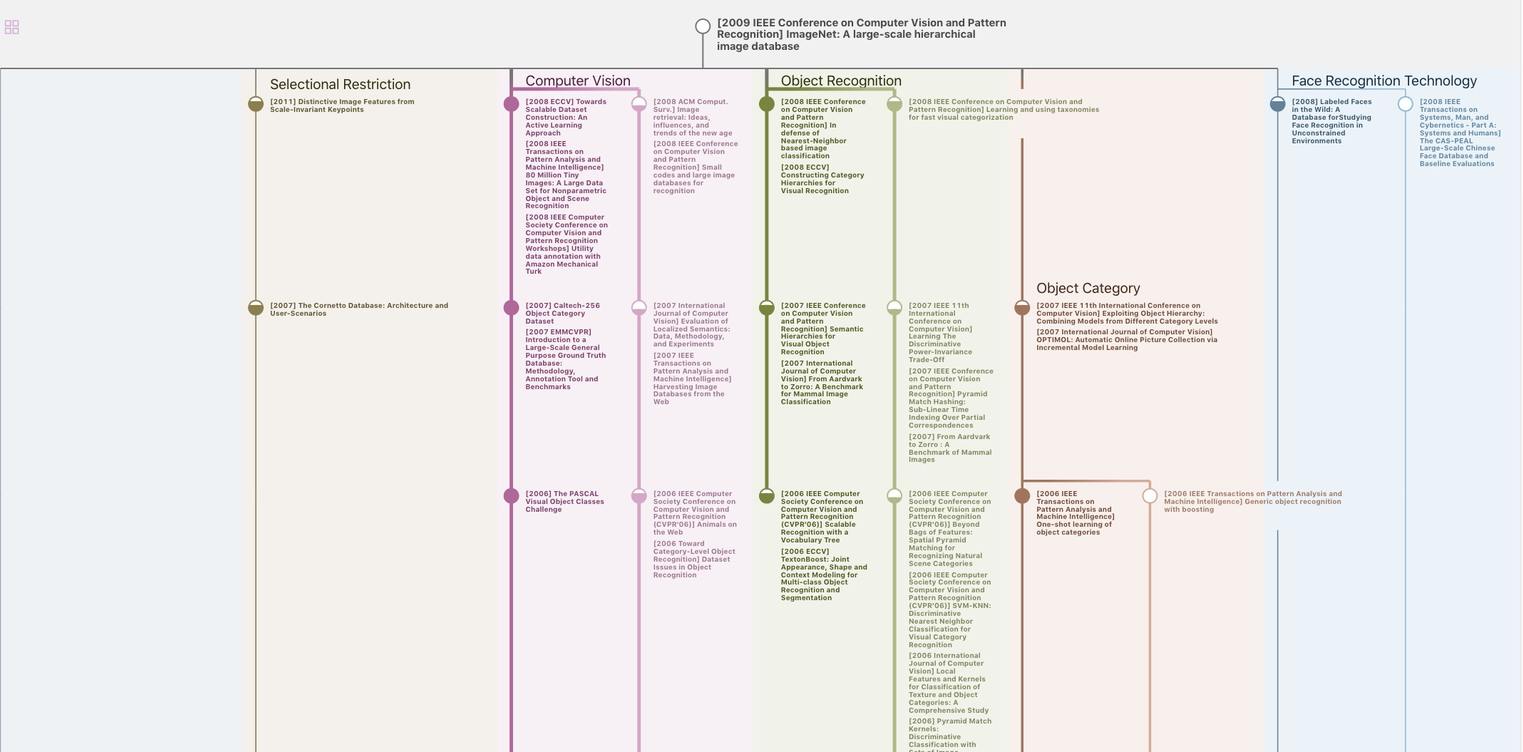
生成溯源树,研究论文发展脉络
Chat Paper
正在生成论文摘要