REST: Enhancing Group Robustness in DNNs Through Reweighted Sparse Training
MACHINE LEARNING AND KNOWLEDGE DISCOVERY IN DATABASES: RESEARCH TRACK, ECML PKDD 2023, PT II(2023)
摘要
The deep neural network (DNN) has been proven effective in various domains. However, they often struggle to perform well on certain minority groups during inference, despite showing strong performance on the majority of data groups. This is because over-parameterized models learned bias attributes from a large number of bias-aligned training samples. These bias attributes are strongly spuriously correlated with the target variable, causing the models to be biased towards spurious correlations (i.e., bias-conflicting). To tackle this issue, we propose a novel reweighted sparse training framework, dubbed as REST, which aims to enhance the performance of biased data while improving computation and memory efficiency. Our proposed REST framework has been experimentally validated on three datasets, demonstrating its effectiveness in exploring unbiased subnetworks. We found that REST reduces the reliance on spuriously correlated features, leading to better performance across a wider range of data groups with fewer training and inference resources. We highlight that the REST framework represents a promising approach for improving the performance of DNNs on biased data, while simultaneously improving computation and memory efficiency. By reducing the reliance on spurious correlations, REST has the potential to enhance the robustness of DNNs and improve their generalization capabilities. Code is released at https://github.com/zhao1402072392/REST.
更多查看译文
关键词
Unbiased Learning,Minority group,Sparse training
AI 理解论文
溯源树
样例
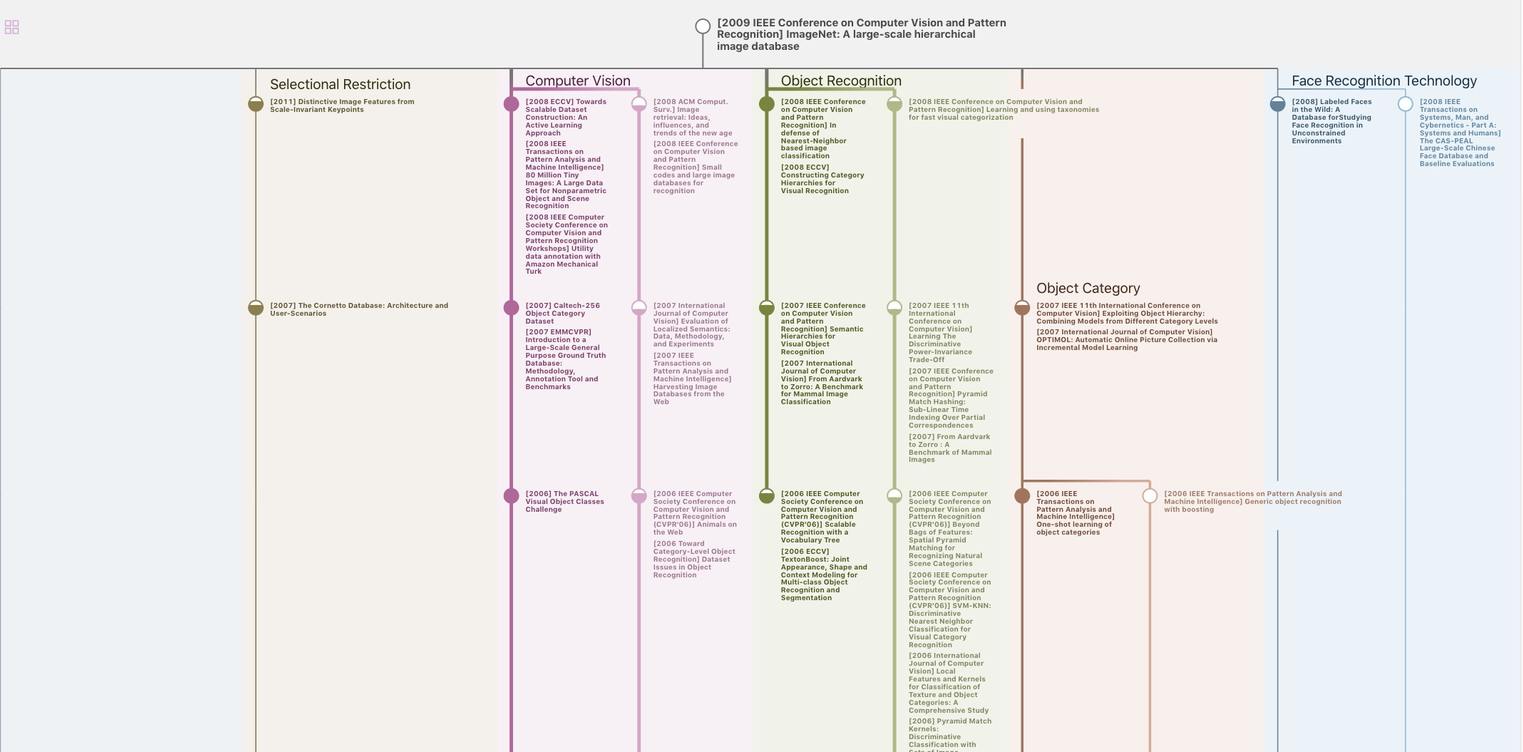
生成溯源树,研究论文发展脉络
Chat Paper
正在生成论文摘要